Optimal Robust Classification Trees
semanticscholar(2021)
摘要
In many high-stakes domains, the data used to drive machine learning algorithms is noisy (due to e.g., the sensitive nature of the data being collected, limited resources available to validate the data, etc). This may cause a distribution shift to occur, where the distribution of the training data does not match the distribution of the testing data. In the presence of distribution shifts, any trained model can perform poorly in the testing phase. In this paper, motivated by the need for interpretability and robustness, we propose a mixed-integer optimization formulation and a tailored solution algorithm for learning optimal classification trees that are robust to adversarial perturbations in the data features. We evaluate the performance of our approach on numerous publicly available datasets, and compare the performance to a regularized, nonrobust optimal tree. We show an increase of up to 14.16% in worst-case accuracy and increase of up to 4.72% in averagecase accuracy across several data sets and distribution shifts from using our robust solution in comparison to the nonrobust solution.
更多查看译文
AI 理解论文
溯源树
样例
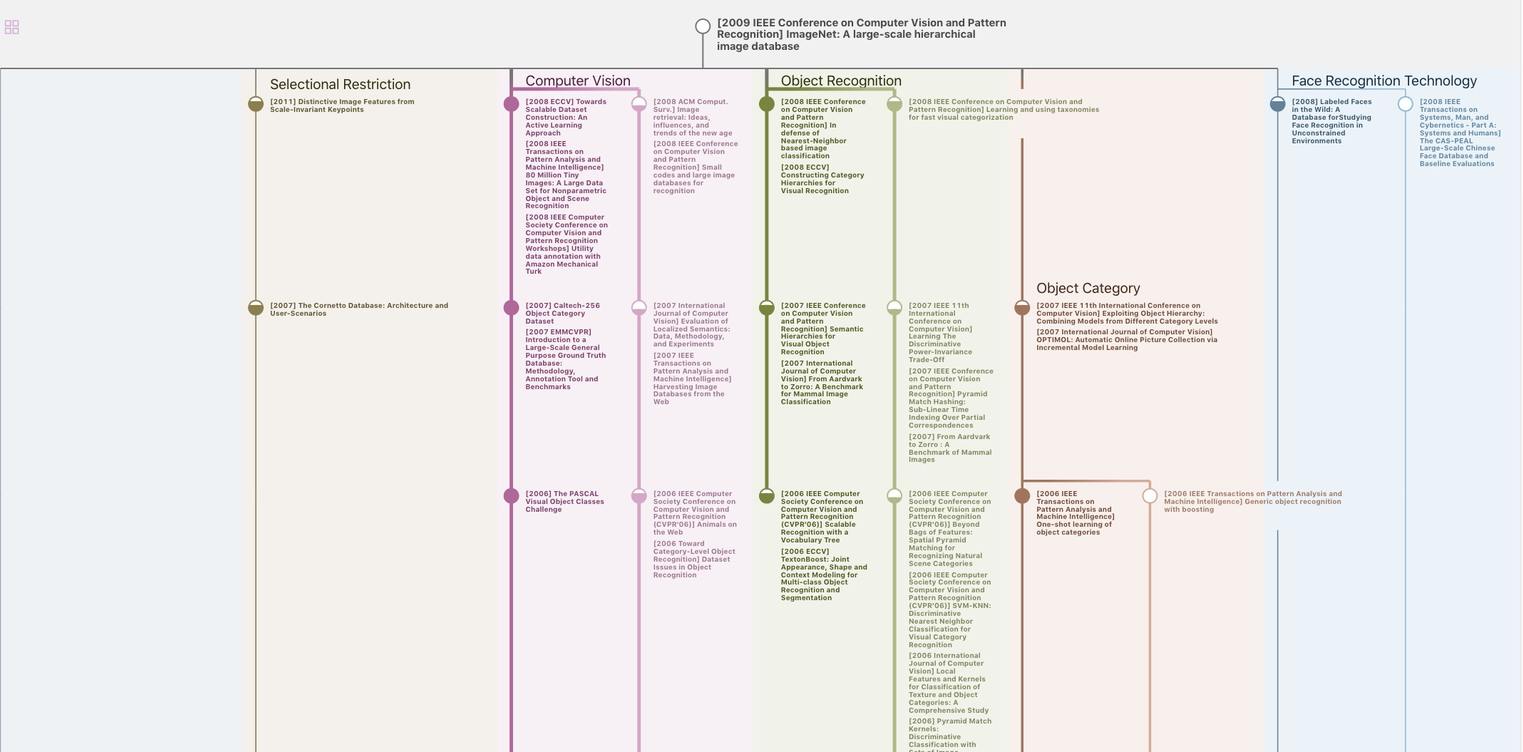
生成溯源树,研究论文发展脉络
Chat Paper
正在生成论文摘要