Clinical Implementation of Deep Learning- Accelerated HASTE and TSE
semanticscholar(2021)
摘要
Magnetic resonance imaging (MRI) has become a modality of choice for the diagnosis of several diseases and is currently indispensable in healthcare. One big disadvantage of MRI is the long duration of the examination, usually ranging between 20 and 60 minutes for body trunk imaging. Long acquisition times come with downsides, such as decreased image quality due to motion artifacts, increased costs, and reduced patient throughput [1]. In view of the limited availability of MRI scanners in general, MRI remains a scarce and more expensive resource than other imaging techniques with limited access to patients in need. In order to improve this situation, over the past few decades, different acceleration strategies such as compressed sensing (CS) and parallel imaging (PI) have been proposed and established. Recently a revolutionary development based on artificial intelligence has been implemented to further accelerate the acquisition and improve the image quality at the same time: Deep Learning (DL) reconstruction has come to the fore and is gradually being implemented in clinical routine [2‒4]. The aim of this report is to describe the first clinical implementation of DL-accelerated, T2-weighted (T2w) half-Fourier single-shot turbo spin echo (HASTE)1 sequences of the upper abdomen as well as T2w and proton density (PD)-weighted turbo spin echo (TSE) sequences for musculoskeletal imaging in routine daily workflow. The novel DL-accelerated sequences are evaluated in terms of feasibility and image quality compared to standard sequences. MRI technique
更多查看译文
AI 理解论文
溯源树
样例
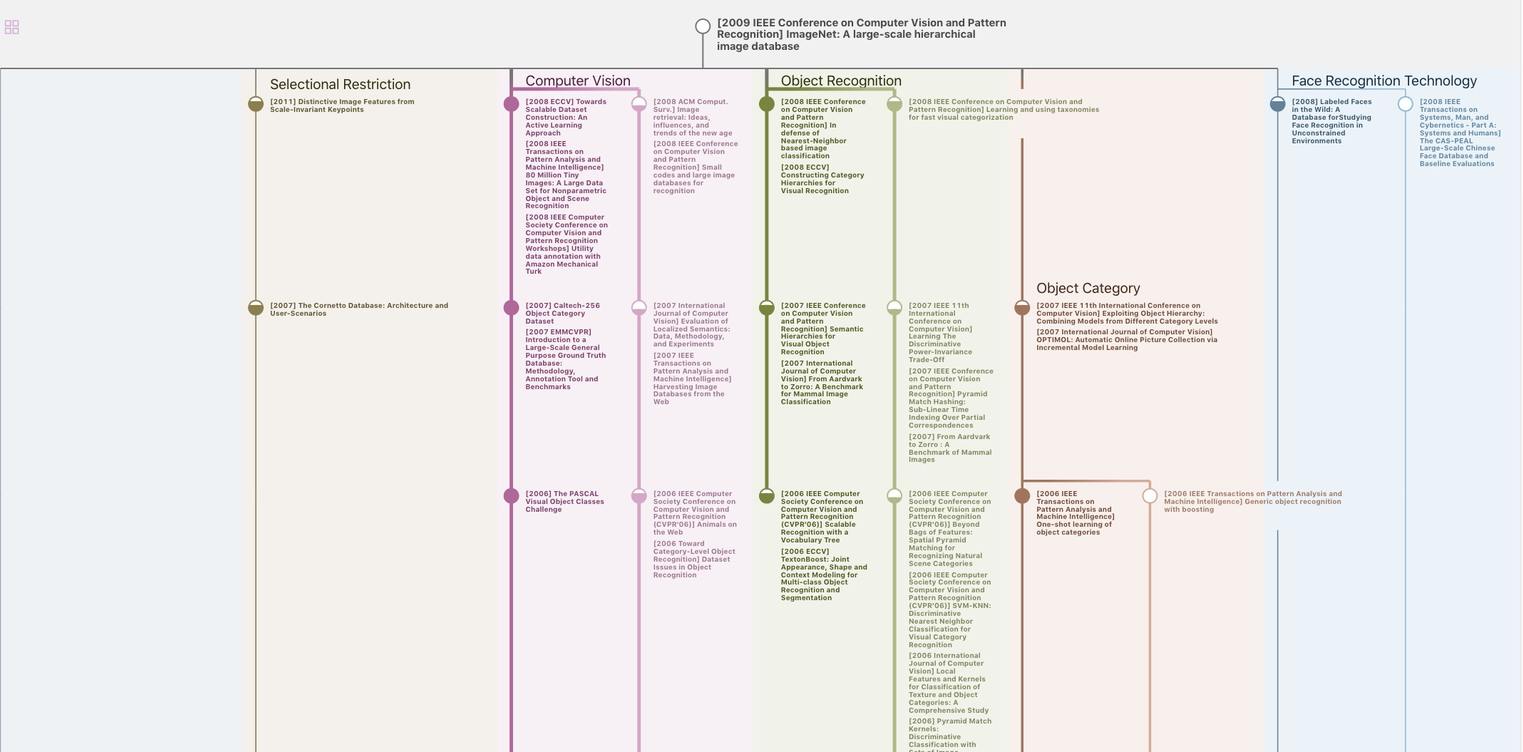
生成溯源树,研究论文发展脉络
Chat Paper
正在生成论文摘要