Detecting outlying demand in multi-leg bookings for transportation networks
arXiv (Cornell University)(2021)
摘要
Planners require accurate demand forecasts when optimising offers to maximise revenue on network products, such as railway itineraries. However, network effects complicate demand forecasting in general and outlier detection in particular. For example, sudden increases in demand for a specific destination in a transportation network not only affect legs arriving at that destination, but also their feeder legs. Network effects are particularly relevant when transport service providers, such as railway or coach companies, offer many multi-leg itineraries. In this paper, we present a novel method for generating automated ranked outlier lists to support analysts in adjusting demand forecasts. To that end, we propose a two-step method for detecting outlying demand from transportation network bookings. The first step clusters network legs to appropriately partition and pool booking patterns. The second step identifies outliers within each cluster and creates a ranked alert list of affected legs. We show that this method outperforms analyses that independently consider leg data without regard for network implications, especially in highly-connected networks where most passengers book multi-leg itineraries. A simulation study demonstrates the robustness of the approach and quantifies the potential revenue benefits from adjusting network demand forecasts for offer optimisation. Finally, we illustrate the applicability on empirical data obtained from Deutsche Bahn.
更多查看译文
关键词
outlying demand,transportation,multi-leg
AI 理解论文
溯源树
样例
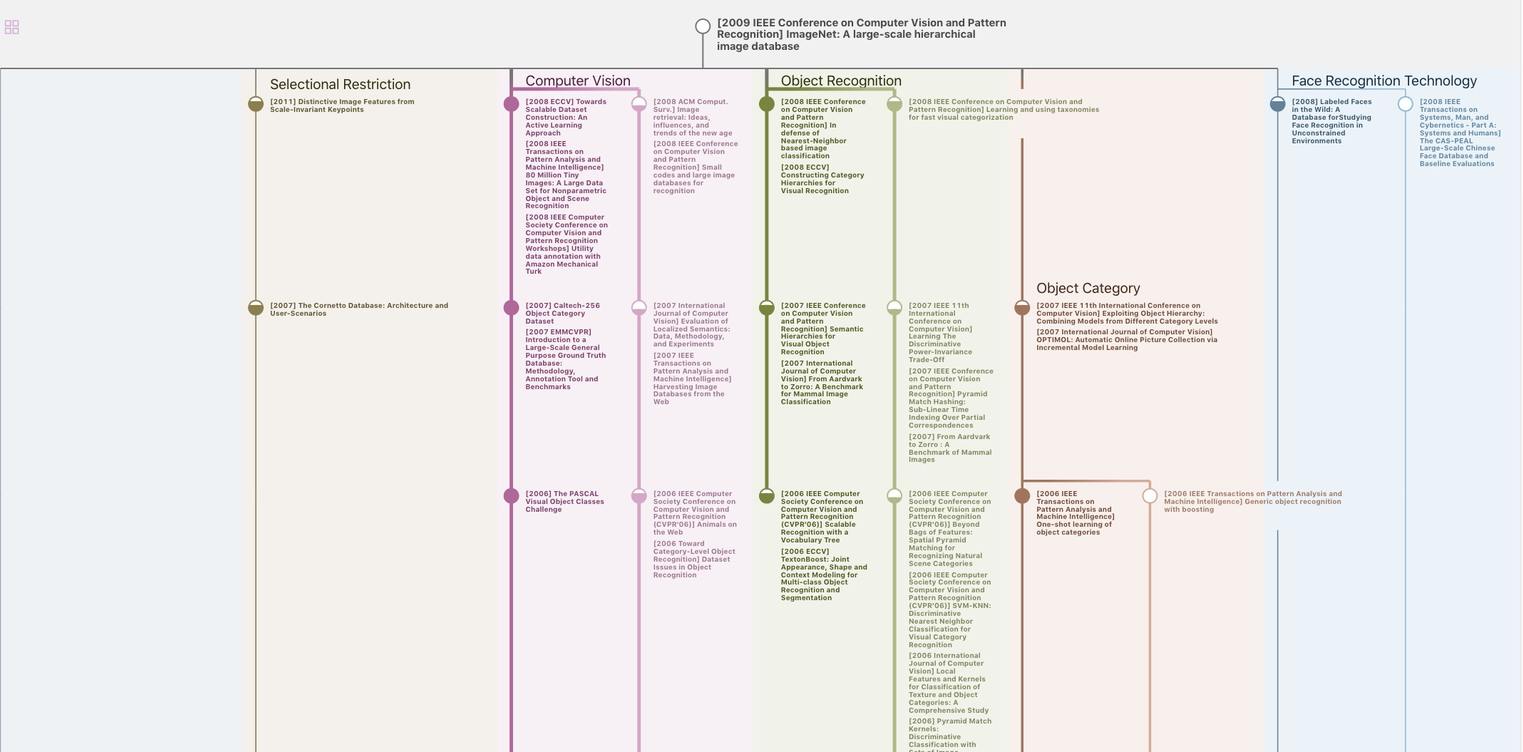
生成溯源树,研究论文发展脉络
Chat Paper
正在生成论文摘要