Conditioning of Reinforcement Learning Agents and its Policy Regularization Application
semanticscholar(2020)
摘要
The role of conditioning was studied for supervised learning problems (Pennington et al., 2017). It also was shown that conditioning regularization can help to avoid the “mode-collapse” problem in Generative Adversarial Networks (Odena et al., 2018). In this paper, we try to answer the following question: Can information about policy conditioning help to shape a more stable and general policy of reinforcement learning agents? To answer this question, we conduct a study of conditioning behavior during policy optimization. To the best of our knowledge, this is the first work that research condition number in reinforcement learning agents. We propose a conditioning regularization algorithm and test its performance on the range of continuous control tasks. Finally, we compare algorithms on the CoinRun (Cobbe et al., 2019) environment with separated train end test levels to analyze how conditioning regularization contributes to agents’ generalization.
更多查看译文
AI 理解论文
溯源树
样例
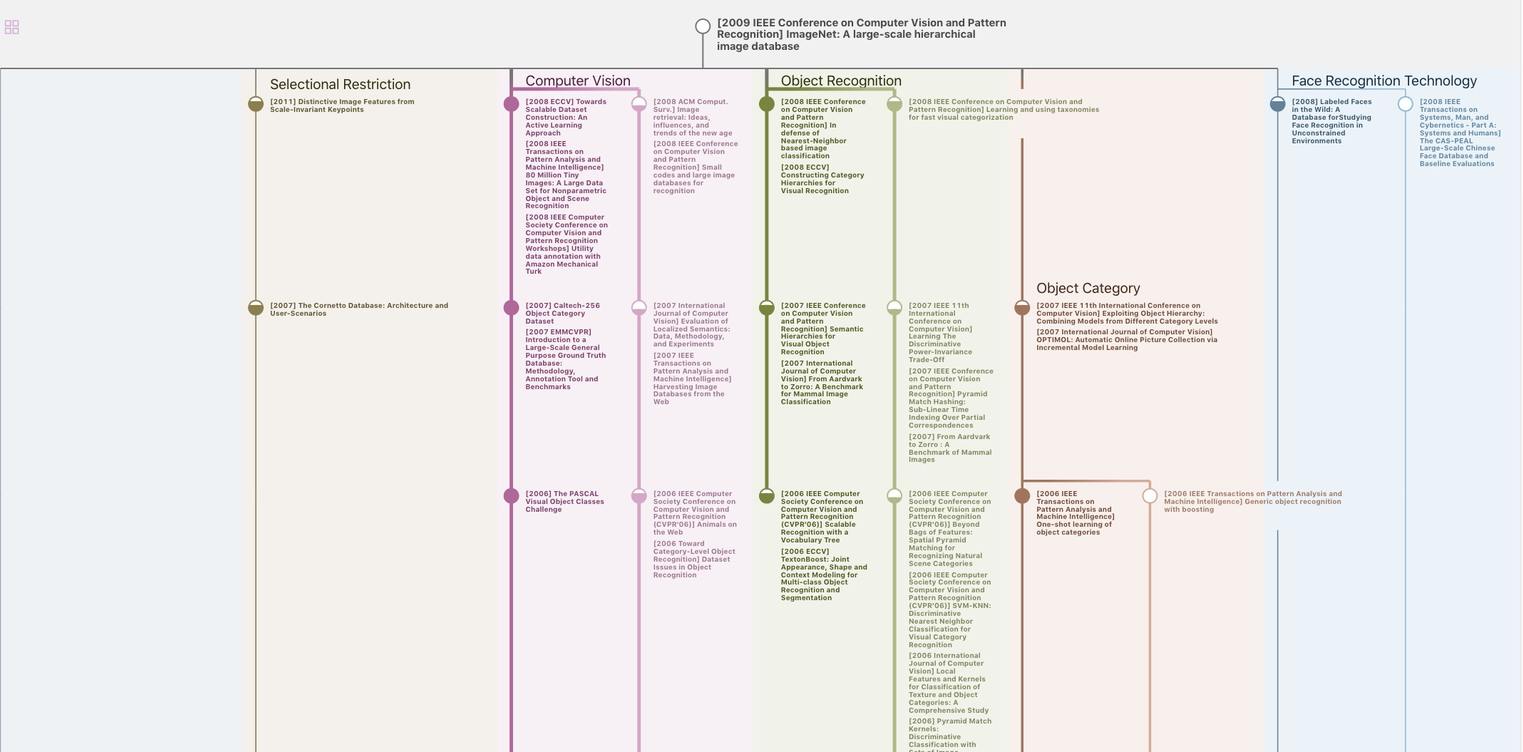
生成溯源树,研究论文发展脉络
Chat Paper
正在生成论文摘要