Smart neurocare approach for detection of epileptic seizures using deep learning based temporal analysis of EEG patterns
Multimedia Tools and Applications(2022)
摘要
Epilepsy is a psychosocial neurological disorder, which emerges as a major threat to public health. In this age of the internet of things, the smart diagnosis of epilepsy has gained huge research attention with machine learning-based seizure detection in cloud-fog assisted environments. The present paper also proposes a cloud-fog integrated smart neurocare approach, which performs a temporal analysis of raw electroencephalogram (EEG) signals using deep learning to detect the occurrence of epileptic seizures. This patient-independent approach makes use of single-channel EEG signals to achieve real-time and computationally efficient seizure detection at fog layer devices. It employs a maximum variance-based channel selection procedure to select only one channel of raw scalp EEG signals, followed by their filtering and segmentation into various short-duration temporal segments. To analyse EEG patterns, these segments are further fed to the proposed models of convolutional neural network, recurrent neural network and stacked autoencoder deep learning classifiers. The performance analysis through simulation results evidently reveals that the proposed convolutional neural network-based temporal analysis approach performs better than other approaches. It realises an optimum accuracy of 96.43%, sensitivity of 100% and specificity of 93.33% for 30 s duration EEG segments of CHB-MIT dataset and achieves 100% accuracy, sensitivity and specificity values for EEG segments of Bonn dataset for 23.6 s EEG segments. Thus, the proposed convolutional neural network-based approach emerges as an appropriate method for rapid and accurate detection of epileptic seizures in fog-cloud integrated environment.
更多查看译文
关键词
Deep learning,Epilepsy,EEG,Fog computing,Internet of things,Healthcare
AI 理解论文
溯源树
样例
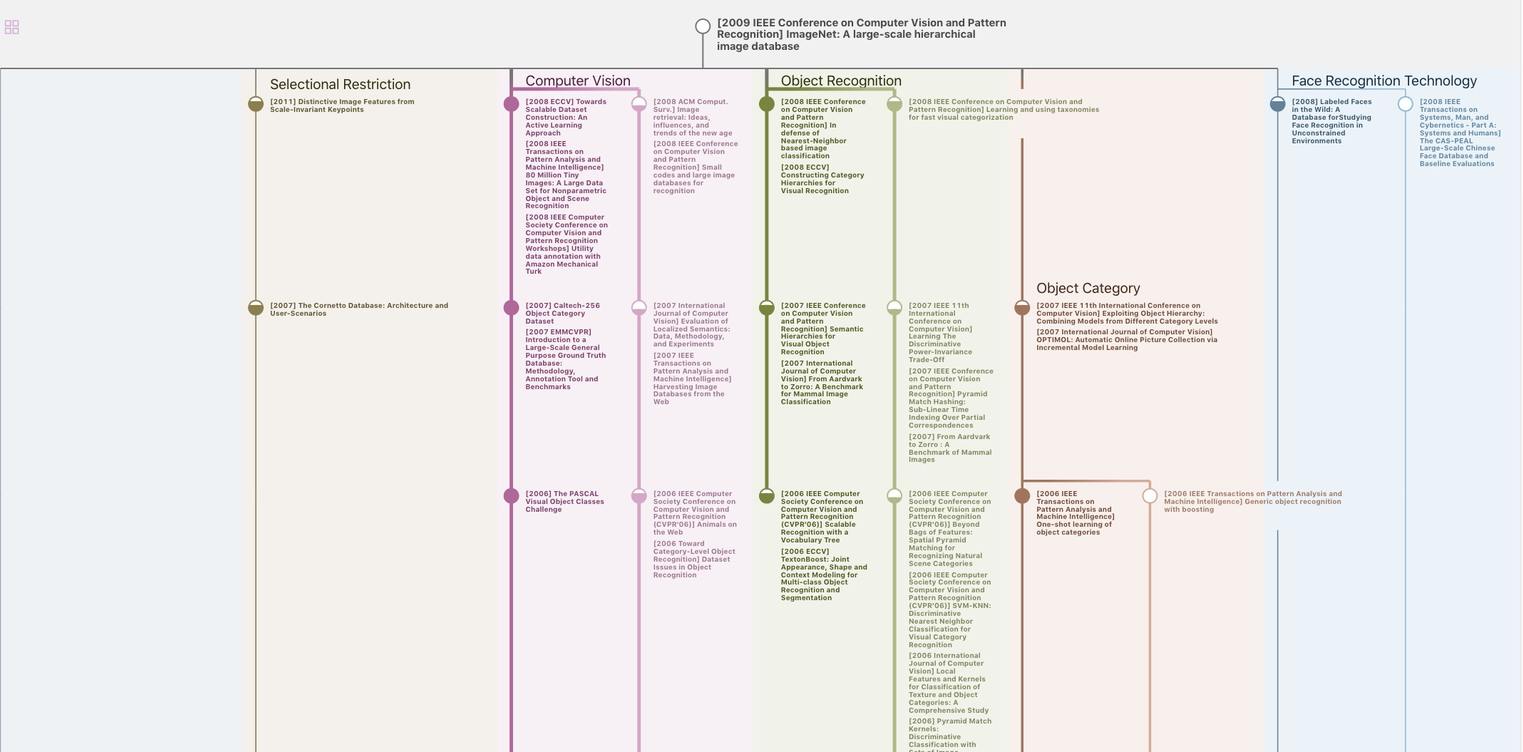
生成溯源树,研究论文发展脉络
Chat Paper
正在生成论文摘要