Binary Many-Objective Particle Swarm Optimization of EEG Channel Selection for Emotion Recognition with Recurrent Convolutional Autoencoder
semanticscholar(2021)
摘要
Electroencephalogray devices are popular for both medical and commercial use such as in emotion recognition. One of the remaining challenges in processing EEG signals is the selection of optimal channels. This paper proposes a new approach based on binary multi-swarm optimization handling classification error rate, specificity, sensitivity as objectives. More specifically, we perform an unsupervised feature learning with recurrent convolutional layers based on autoencoder architecture directly from clean EEG signals. In order to provide a scientific evidence, extensive validation on three public affective benchmarks, i. e. DASPS, DEAP, and SEED which are different in channel number, used stimuli, and participant ratings, was carried out with subject independent scheme.
更多查看译文
AI 理解论文
溯源树
样例
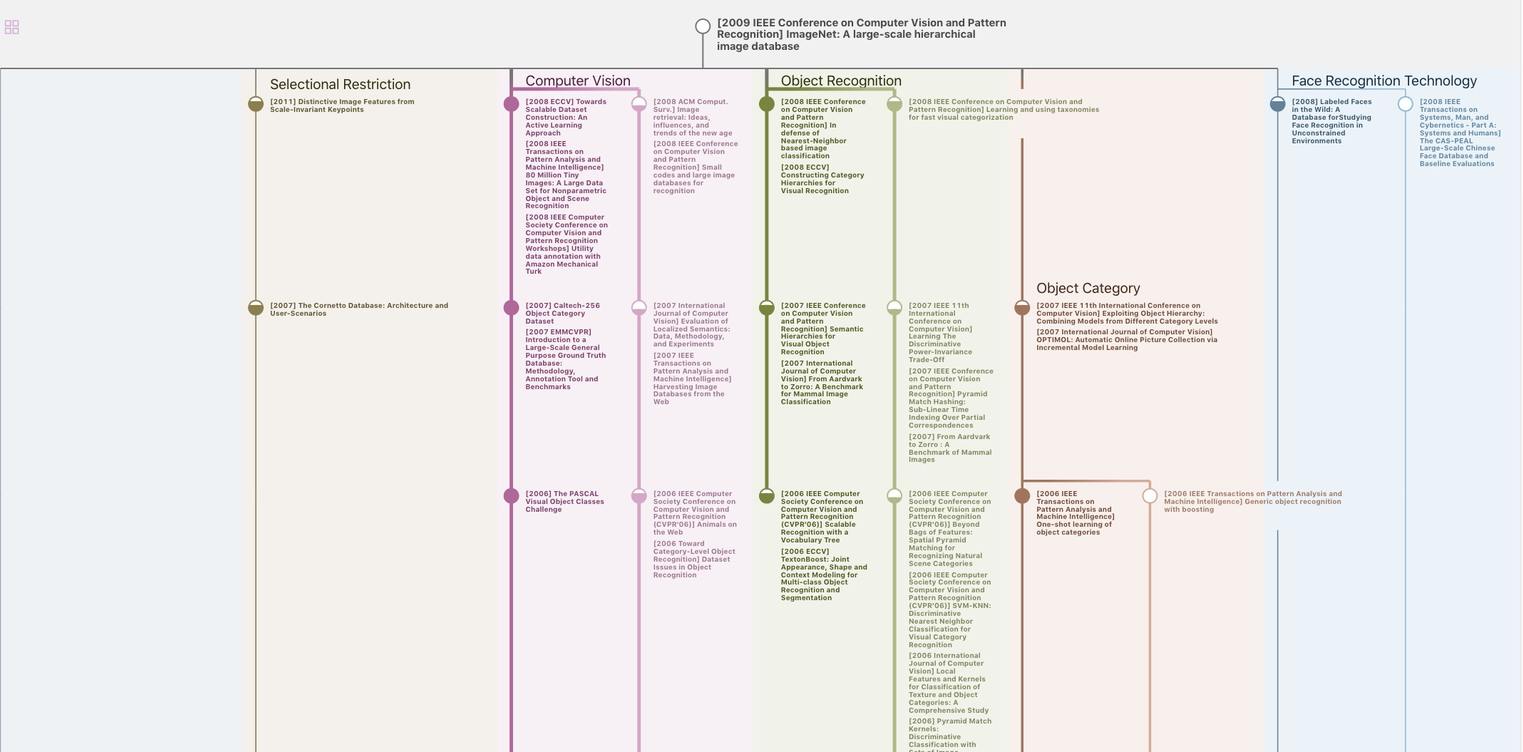
生成溯源树,研究论文发展脉络
Chat Paper
正在生成论文摘要