The Role of (non-)Topological Features as Drivers of Systemic Risk: a machine learning approach
RePEc: Research Papers in Economics(2021)
摘要
The purpose of this paper is to assess the role of financial and topological variables as determinants of systemic risk (SR). The SR, for different levels of the initial shock, is computed for institutions in the Brazilian interbank market by applying the differential DebtRank methodology. The financial institution(FI)-specific determinants of SR are evaluated through two machine learning techniques: XGBoost and random forest. Shapley values analysis provided a better interpretability for our results. Furthermore, we performed this analysis separately for banks and credit unions. We have found the importance of a given feature in driving SR varies with i) the level of the initial shock, ii) the type of FI, and iii) the dimension of the risk which is being assessed – i.e., potential loss caused by (systemic impact) or imputed to (systemic vulnerability) the FI. Systemic impact is mainly driven by topological features for both types of FIs. However, while the importance of topological features to the prediction of systemic impact of banks increases with the level of the initial shock, it decreases for credit unions. Concerning systemic vulnerability, this is mainly determined by financial features, whose importance increases with the initial shock level for both types of FIs.
更多查看译文
关键词
systemic risk,features,learning
AI 理解论文
溯源树
样例
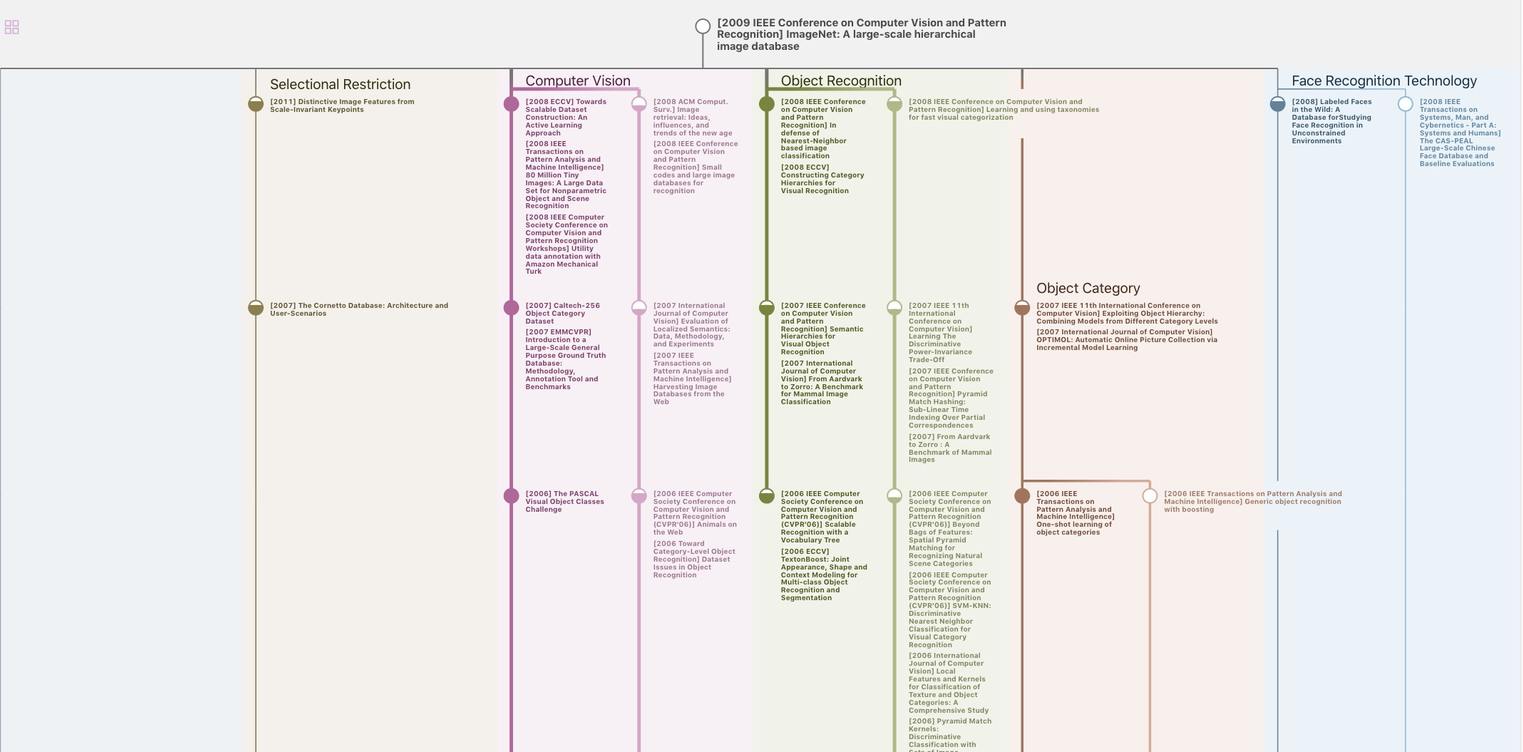
生成溯源树,研究论文发展脉络
Chat Paper
正在生成论文摘要