PEAF: Learnable Power Efficient Analog Acoustic Features for Audio Recognition
semanticscholar(2021)
摘要
At the end of Moore’s law, new computing paradigms are required to prolong the battery life of wearable and IoT smart audio devices. Theoretical analysis and physical validation have shown that analog signal processing (ASP) can be more power-efficient than its digital counterpart in the realm of low-to-medium signal-to-noise ratio applications. In addition, ASP allows a direct interface with an analog microphone without a power-hungry analog-to-digital converter. Here, we present power-efficient analog acoustic features (PEAF) that are validated by fabricated CMOS chips for running audio recognition. Linear, non-linear, and learnable PEAF variants are evaluated on two speech processing tasks that are demanded in many battery-operated devices: wake word detection (WWD) and keyword spotting (KWS). Compared to digital acoustic features, higher power efficiency with competitive classification accuracy can be obtained. A novel theoretical framework based on information theory is established to analyze the information flow in each individual stage of the feature extraction pipeline. The analysis identifies the information bottleneck and helps im-prove the KWS accuracy by up to 7%. This work may pave the way to building more power-efficient smart audio devices with best-in-class inference performance.
更多查看译文
AI 理解论文
溯源树
样例
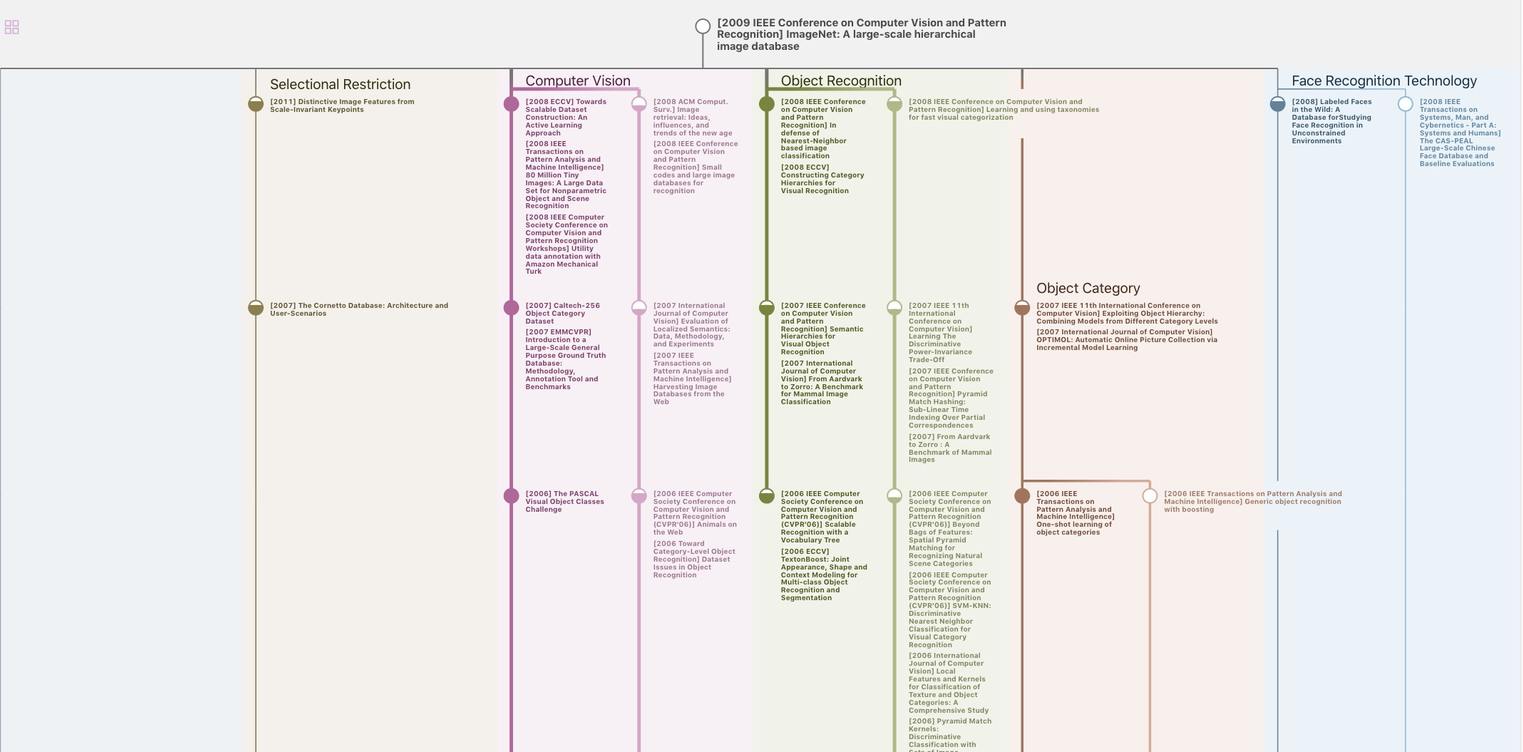
生成溯源树,研究论文发展脉络
Chat Paper
正在生成论文摘要