Computational Imaging Methods for Analysis of DaTScan SPECT Images
semanticscholar(2022)
摘要
OF THE THESIS Computational Imaging Methods for Analysis of DaTScan SPECT Images by Hae Sol Moon Master of Science in Biomedical Engineering Washington University in St. Louis, May 2020 Research Advisor: Professor Abhinav K. Jha There is an important need to develop biomarkers to improve the diagnosis and assess the severity of Parkinson’s disease (PD). The potential to derive such biomarkers from quantitative dopamine transporter scan (DaT-Scan) single-photon emission computed tomography (SPECT) imaging, in particular the uptake of DaT in the caudate, putamen, and globus pallidus regions, is highly appealing as imaging is non-invasive and DaTScan is already used in the management of patients with PD. However, reliable quantification requires reliable segmentation of these regions in these images. Reliable segmentation is challenging due to the limited spatial resolution and high image noise in SPECT images and the physiological variability in these regions. To address this issue, we propose a three-dimensional physicsguided estimation-based method for segmenting SPECT images. The method implicitly incorporates the prior distribution of boundaries of caudate, putamen and globus pallidus, as can be obtained from high-resolution MR images of patients scanned previously, during the training process. Our approach is guided by the physics of the SPECT imaging, and thus inherently accounts for the two sources of partial volume effects in SPECT images, ix namely limited system resolution and tissue-fraction effects. The proposed method was evaluated both qualitatively and quantitatively using highly realistic simulation studies. The method yielded accurate boundaries of the caudate, putamen, and globus pallidus regions, provided reliable estimates of the specific binding ratios of these regions, and significantly out-performed several commonly used segmentation methods. We have implemented geometric transfer matrix (GTM) method that uses this delineated boundary to compensate for partial volume effects, with the goal of estimating more accurate quantification results.
更多查看译文
AI 理解论文
溯源树
样例
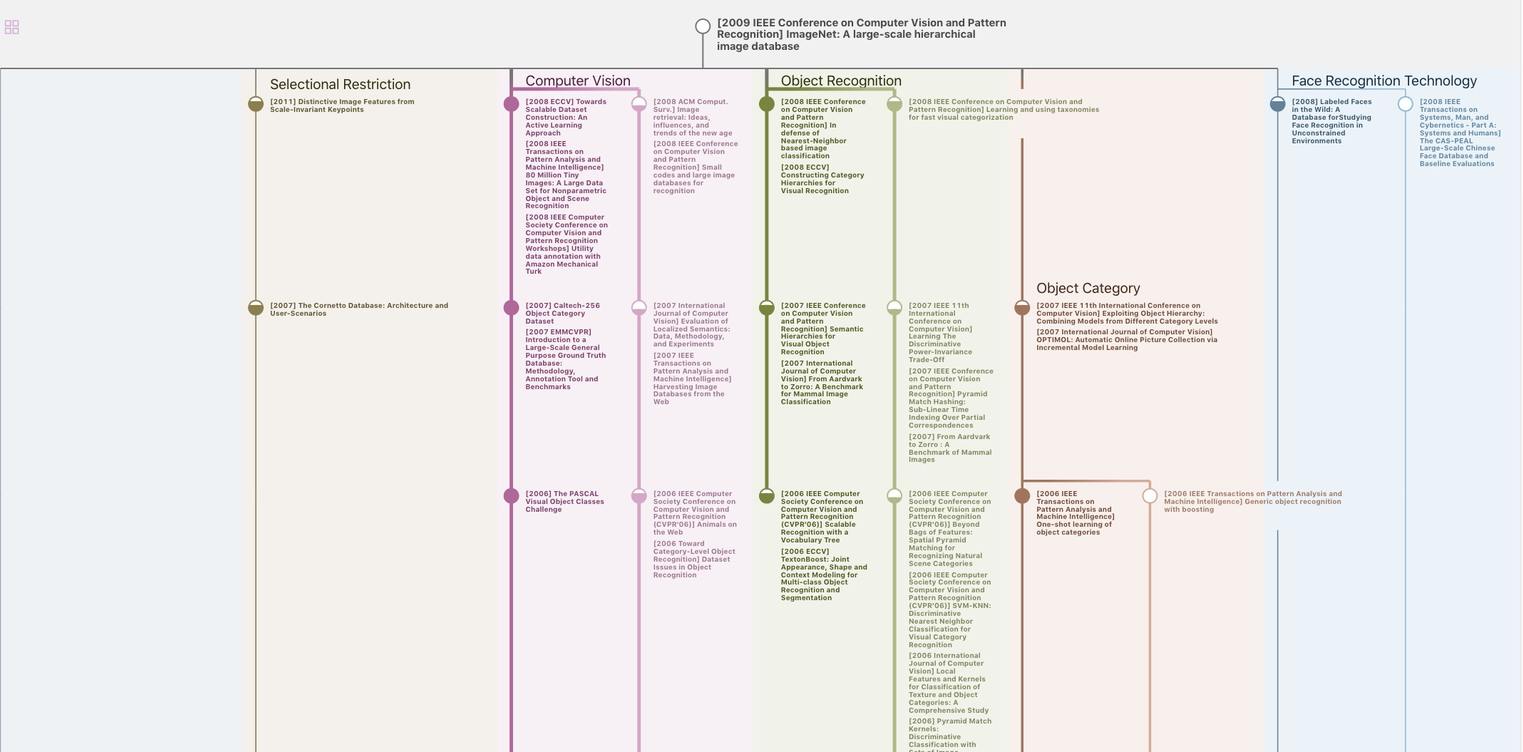
生成溯源树,研究论文发展脉络
Chat Paper
正在生成论文摘要