A Computational Acquisition Model for Multimodal Word Categorization
North American Chapter of the Association for Computational Linguistics (NAACL)(2022)
摘要
Recent advances in self-supervised modeling of text and images open new opportunities for computational models of child language acquisition, which is believed to rely heavily on cross-modal signals. However, prior studies have been limited by their reliance on vision models trained on large image datasets annotated with a pre-defined set of depicted object categories. This is (a) not faithful to the information children receive and (b) prohibits the evaluation of such models with respect to category learning tasks, due to the pre-imposed category structure. We address this gap, and present a cognitively-inspired, multimodal acquisition model, trained from image-caption pairs on naturalistic data using cross-modal self-supervision. We show that the model learns word categories and object recognition abilities, and presents trends reminiscent of those reported in the developmental literature. We make our code and trained models public for future reference and use.
更多查看译文
关键词
multimodal word categorization,computational acquisition model
AI 理解论文
溯源树
样例
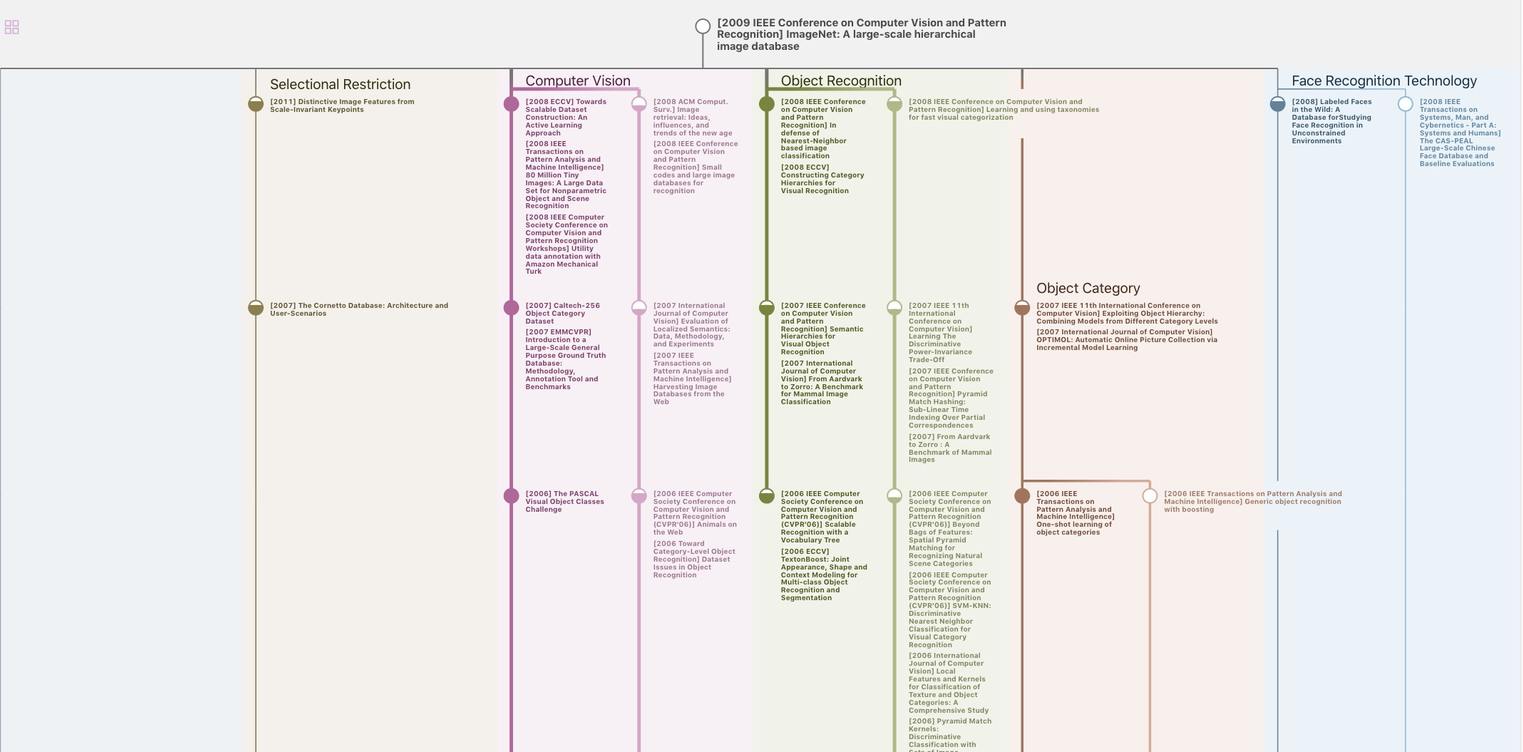
生成溯源树,研究论文发展脉络
Chat Paper
正在生成论文摘要