FETA: A Benchmark for Few-Sample Task Transfer in Open-Domain Dialogue
emnlp 2022(2022)
摘要
Task transfer, transferring knowledge contained in related tasks, holds the promise of reducing the quantity of labeled data required to fine-tune language models. Dialogue understanding encompasses many diverse tasks, yet task transfer has not been thoroughly studied in conversational AI. This work explores conversational task transfer by introducing FETA: a benchmark for few-sample task transfer in open-domain dialogue. FETA contains two underlying sets of conversations upon which there are 10 and 7 tasks annotated, enabling the study of intra-dataset task transfer; task transfer without domain adaptation. We utilize three popular language models and three learning algorithms to analyze the transferability between 132 source-target task pairs and create a baseline for future work. We run experiments in the single- and multi-source settings and report valuable findings, e.g., most performance trends are model-specific, and span extraction and multiple-choice tasks benefit the most from task transfer. In addition to task transfer, FETA can be a valuable resource for future research into the efficiency and generalizability of pre-training datasets and model architectures, as well as for learning settings such as continual and multitask learning.
更多查看译文
关键词
dialogue,task,benchmark,few-sample,open-domain
AI 理解论文
溯源树
样例
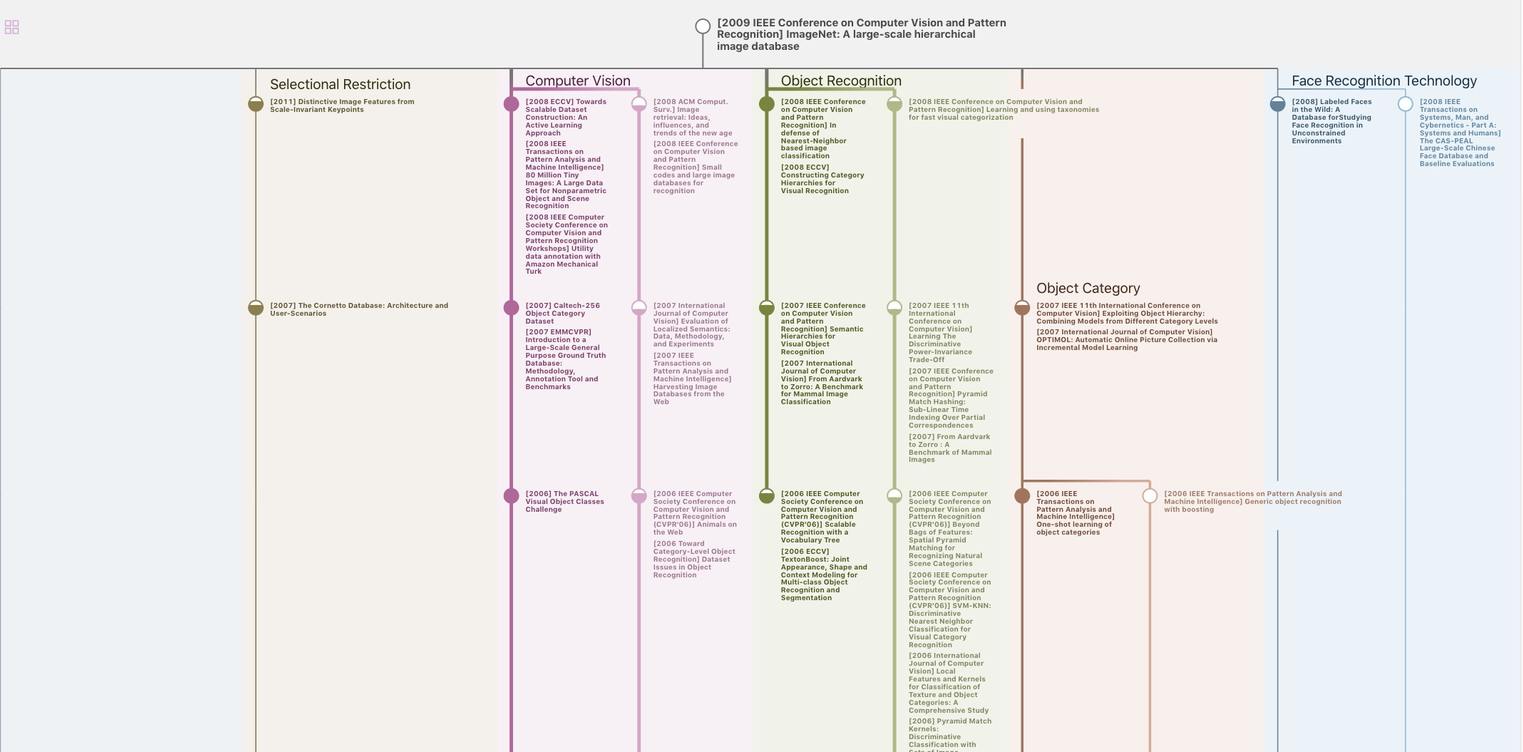
生成溯源树,研究论文发展脉络
Chat Paper
正在生成论文摘要