A gradient sampling method with complexity guarantees for Lipschitz functions in high and low dimensions
NeurIPS 2022(2021)
摘要
Zhang et al. [25] introduced a novel modification of Goldstein’s classical subgradient method, with an efficiency guarantee of O(ε) for minimizing Lipschitz functions. Their work, however, makes use of a nonstandard subgradient oracle model and requires the function to be directionally differentiable. In this paper, we show that both of these assumptions can be dropped by simply adding a small random perturbation in each step of their algorithm. The resulting method works on any Lipschitz function whose value and gradient can be evaluated at points of differentiability. We additionally present a new cutting plane algorithm that achieves better efficiency in low dimensions: O(dε) for Lipschitz functions and O(dε) for those that are weakly convex. School of ORIE, Cornell University, Ithaca, NY 14850, USA. people.orie.cornell.edu/dsd95/. Research of Davis supported by an Alfred P. Sloan research fellowship and NSF DMS award 2047637. Department of Mathematics, U. Washington, Seattle, WA 98195; www.math.washington.edu/∼ddrusv. Research of Drusvyatskiy was supported by NSF DMS-1651851 and CCF-2023166 awards. yintat@uw.edu. Paul G. Allen School of Computer Science and Engineering, U. Washington, Seattle, WA 98195. Supported by NSF awards CCF-1749609, DMS-1839116, DMS-2023166, CCF-2105772, a Microsoft Research Faculty Fellowship, Sloan Research Fellowship, and Packard Fellowship. pswati@uw.edu. U. Washington, Seattle, WA 98195. ghye@mit.edu. Department of Mathematics, Massachusetts Institute of Technology, Cambridge, MA 02139. Supported by an MIT Presidential Fellowship. Part of this work was done while the author was a student at University of Washington.
更多查看译文
关键词
nonconvex optimization,nonsmooth optimization,nonconvex nonsmooth optimization,Goldstein subdifferential,cutting plane method.
AI 理解论文
溯源树
样例
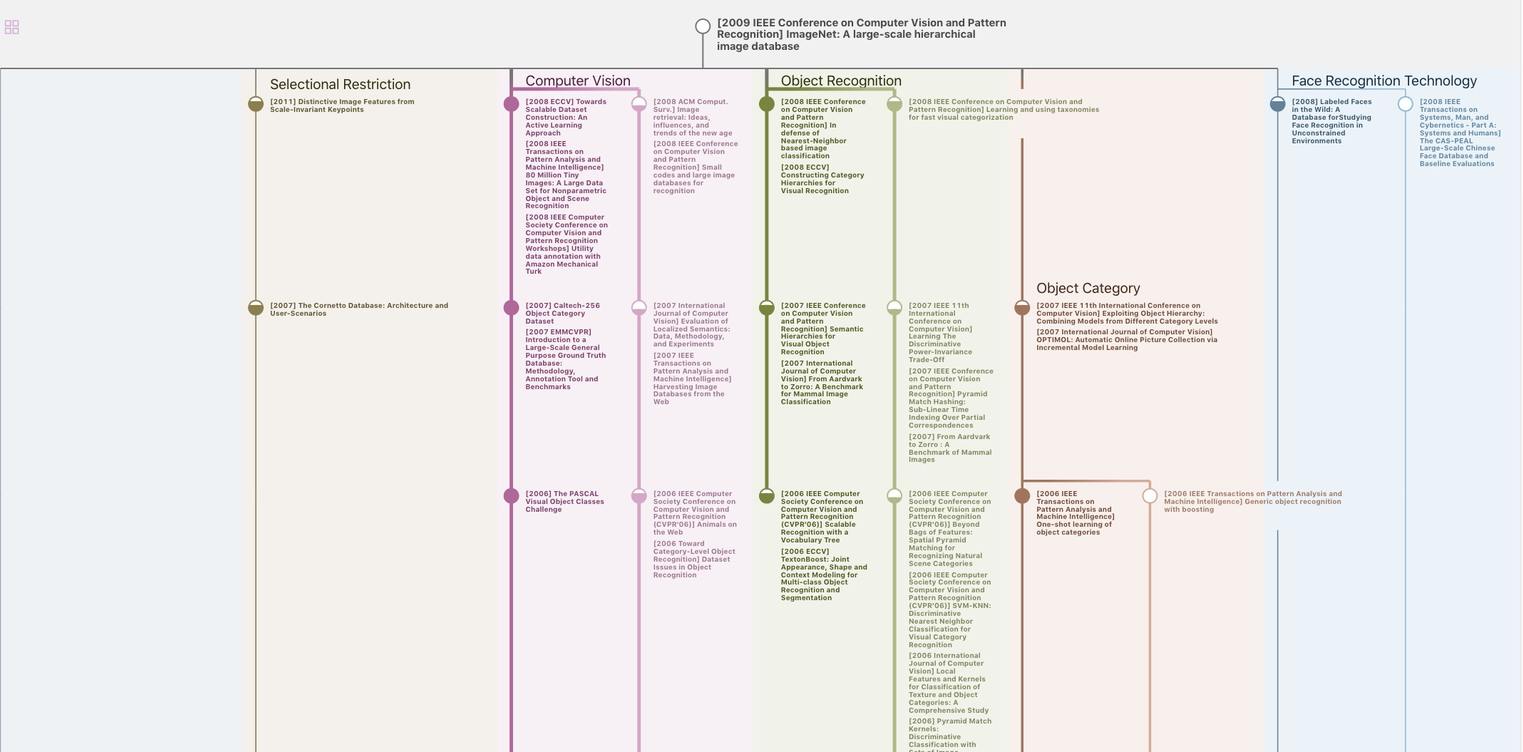
生成溯源树,研究论文发展脉络
Chat Paper
正在生成论文摘要