Task-aware adaptive attention learning for few-shot semantic segmentation
Neurocomputing(2022)
摘要
Few-shot semantic segmentation is a newly developing and challenging computer vision task which aims to predict pixel-wise segmentation on the novel categories where only a few annotated samples are supplied. Because of the scarcity of the annotated novel class samples, the main obstacle of this issue is the diversity of objects in the support set and query set. This paper proposes a novel network aiming to bridge the gap by exploring the correlation between the support feature and the query feature. Specifically, a task-aware adaptive attention module(TAAM) is introduced to extract the task-specific information from the current input and integrates it into the feature representations both in channel dimension and spatial dimension for adaptive reinforcement. Besides, an additional prediction refinement module(RPM) is attached to further optimize the predictions to present more details of objects. Furthermore, through a non-parameter aggregation operation, the proposed network is easy to generalize to k-shot segmentation without developing specific architectures. Extensive experiments on three benchmarks demonstrate that our method exceeds previous state-of-the-arts with a sizable margin, verifying the effectiveness of the proposed method.
更多查看译文
关键词
Few-shot semantic segmentation,Adaptive feature learning,Attention mechanism,Task-aware
AI 理解论文
溯源树
样例
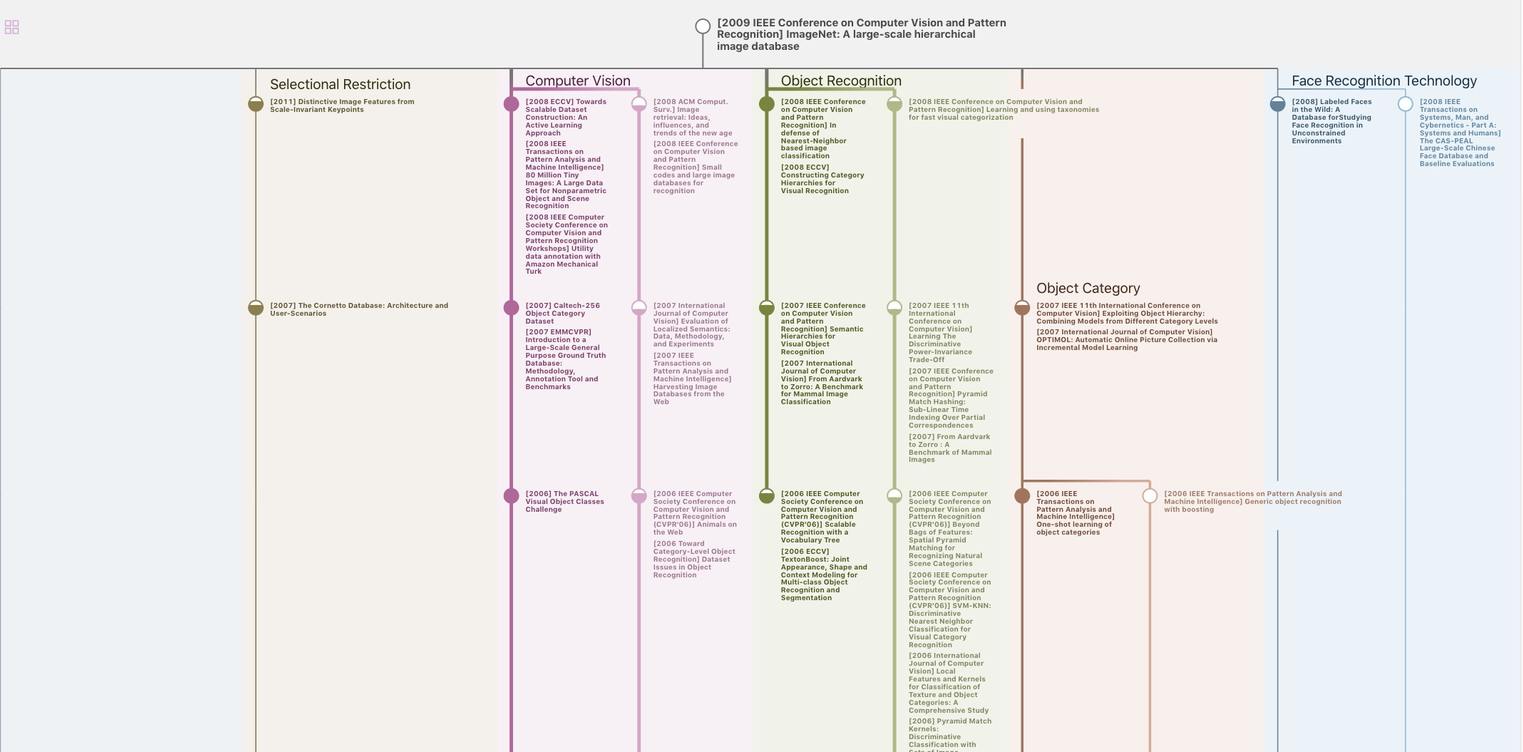
生成溯源树,研究论文发展脉络
Chat Paper
正在生成论文摘要