Few-Annotation Training by Data Augmentation in Agriculture
semanticscholar(2021)
摘要
Automation of agricultural management has become important by an aging population and decreasing agricultural population. A deep neural network can be applied to detect vegetables and fruits on a farm. Detecting objects in an image taken from a camera leads to counting the objects. However, the training cost for the deep neural network is generally high, and it requires a high-quality large dataset and time consumption. Also, there are various kinds of vegetables, and it is not feasible to train and tune neural networks for all of them because creating datasets for all vegetables requires a high cost. Then, we propose the method which employs a few-shot model and data augmentation for few-shot and few-annotated datasets. Finally, we create the novel dataset for detecting eggplants as a dataset for our experiment and evaluation. As a result, the proposed method with the few-annotation and few-shot settings obtained 5 to 20 points improvement of accuracy than that of the Faster R-CNN with the few-shot settings on AP50, and the model converges earlier 50 200 epoch than that of Faster R-CNN. However, the few-shot model with the few-shot setting obtains a higher performance than the few-shot and few-annotation settings on AP50 and converging time.
更多查看译文
AI 理解论文
溯源树
样例
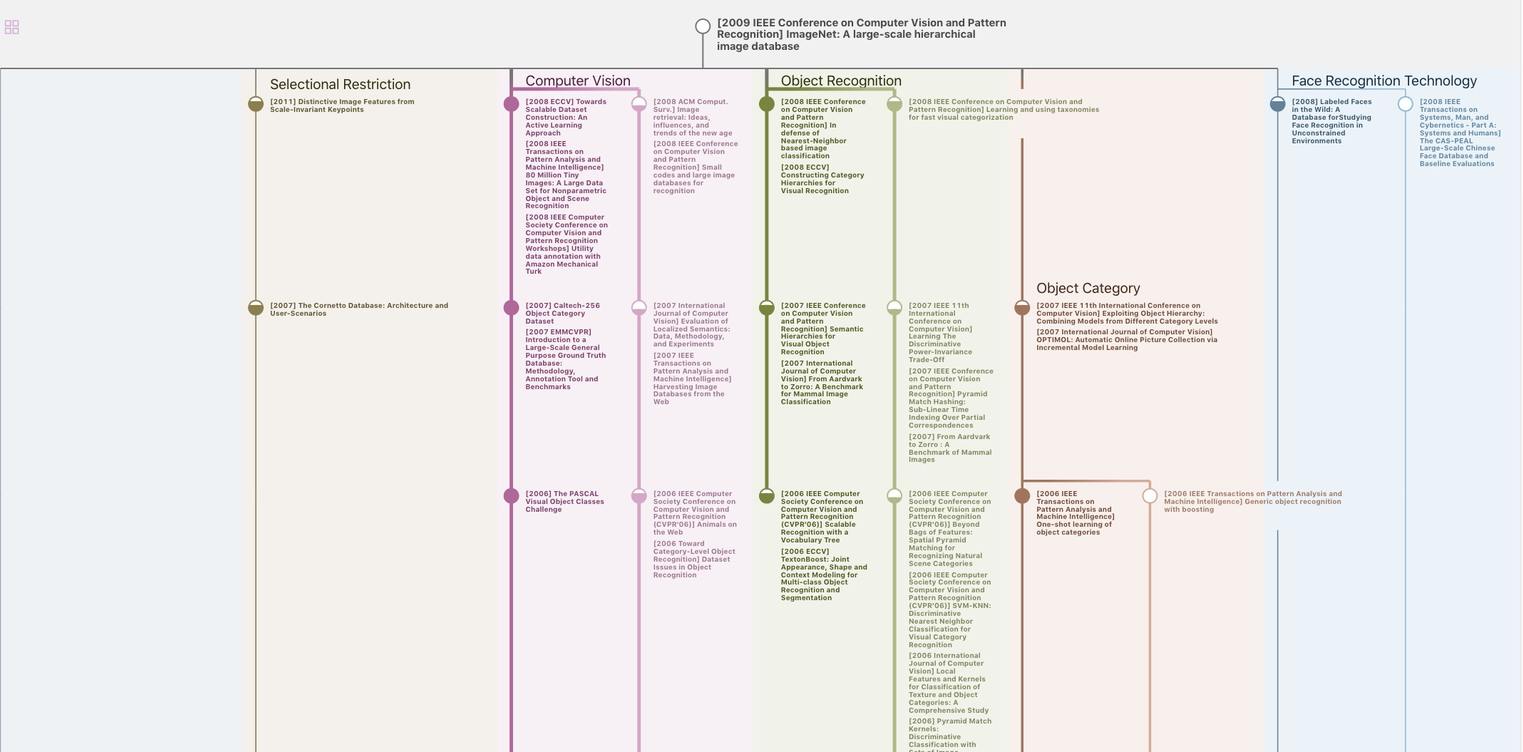
生成溯源树,研究论文发展脉络
Chat Paper
正在生成论文摘要