Self-Supervised Learning for Efficient Antialiasing Seismic Data Interpolation
IEEE TRANSACTIONS ON GEOSCIENCE AND REMOTE SENSING(2022)
摘要
Reconstruction of seismic data is an important but challenging task in seismic data processing. Different machine-learning-based algorithms have been developed to solve this ill-posed problem and achieved great progress. However, most machine-learning-based methods rely on supervised learning where a good training dataset with many complete shot-gathers are required to train the model. Although the generative model has been used for unsupervised learning and reconstructing signals in a shot-gather, it fails to accurately resolve the fine features, especially when aliasing is the main concern. In addition, multiple shots' interpolation problems have not been fully investigated by the unsupervised machine-learning-based approaches. In this work, we propose a self-supervised learning method using a blind-trace network and two antialiasing techniques (automatic spectrum suppression and mix-training) for seismic data reconstruction. The method is validated using challenging and realistic scenarios. Test results show that the method can be applied to single-shot or multiple shots' cases and adapt well to different decimation patterns.
更多查看译文
关键词
Interpolation,Training,Image reconstruction,Standards,Task analysis,Neural networks,Supervised learning,Antialiasing,blind-trace networks (BTNs),seismic interpolation,self-supervised learning,unsupervised learning
AI 理解论文
溯源树
样例
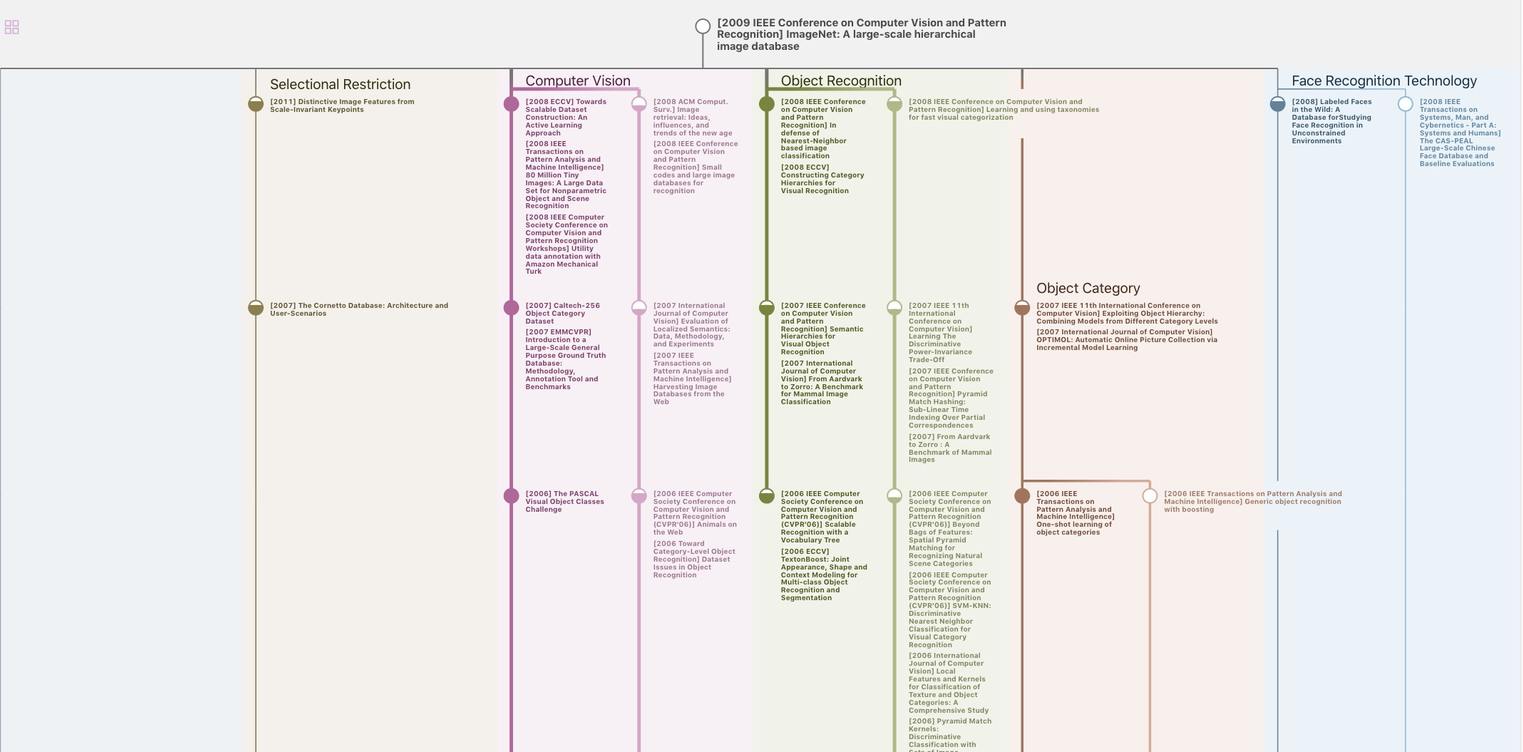
生成溯源树,研究论文发展脉络
Chat Paper
正在生成论文摘要