Authenticating the geographical origin of wine using fluorescence spectroscopy and machine learning
semanticscholar(2022)
摘要
Wine is a luxury product and a global beverage steeped in history and mystery. Over time, various regions have become renowned for the quality of wines they produce, which adds considerable value to the regions and the brands. On the whole, the international wine market is worth many hundreds of billions of dollars, which attracts unscrupulous operators intent on defrauding wine consumers. Countering such fraudulent activities requires the means to test and classify wine, but the task is considerable due to the complexity of wine. However, just as wine origin influences chemical and sensory profiles, indicators of wine provenance are naturally embedded in the chemical composition of wine. A range of methods of varying intricacy are available to analyse wine for authentication of variety or geographical origin. Instruments and techniques within the domain of research laboratories are not so practical or deployable in winery or supply chain settings, however. This is where spectroscopic methods are attractive, as they can be rapid, cost-effective and simple. In the search for such a method, we identified fluorescence spectroscopy, and more specifically, the collection of an excitation-emission matrix (EEM) that acts like a molecular fingerprint. Multivariate statistical modelling is then used in conjunction with the EEM data to develop classification models for wines from various regions. We have developed such a technique, using a relatively new type of machine learning algorithm known as extreme gradient boosting discriminant analysis. This unique approach, which can routinely achieve a level of accuracy of 100% in comparison to ICP-MS at an average of 85%, is being applied to a range of studies on Shiraz and Cabernet Sauvignon wines from different regions of Australia.
更多查看译文
AI 理解论文
溯源树
样例
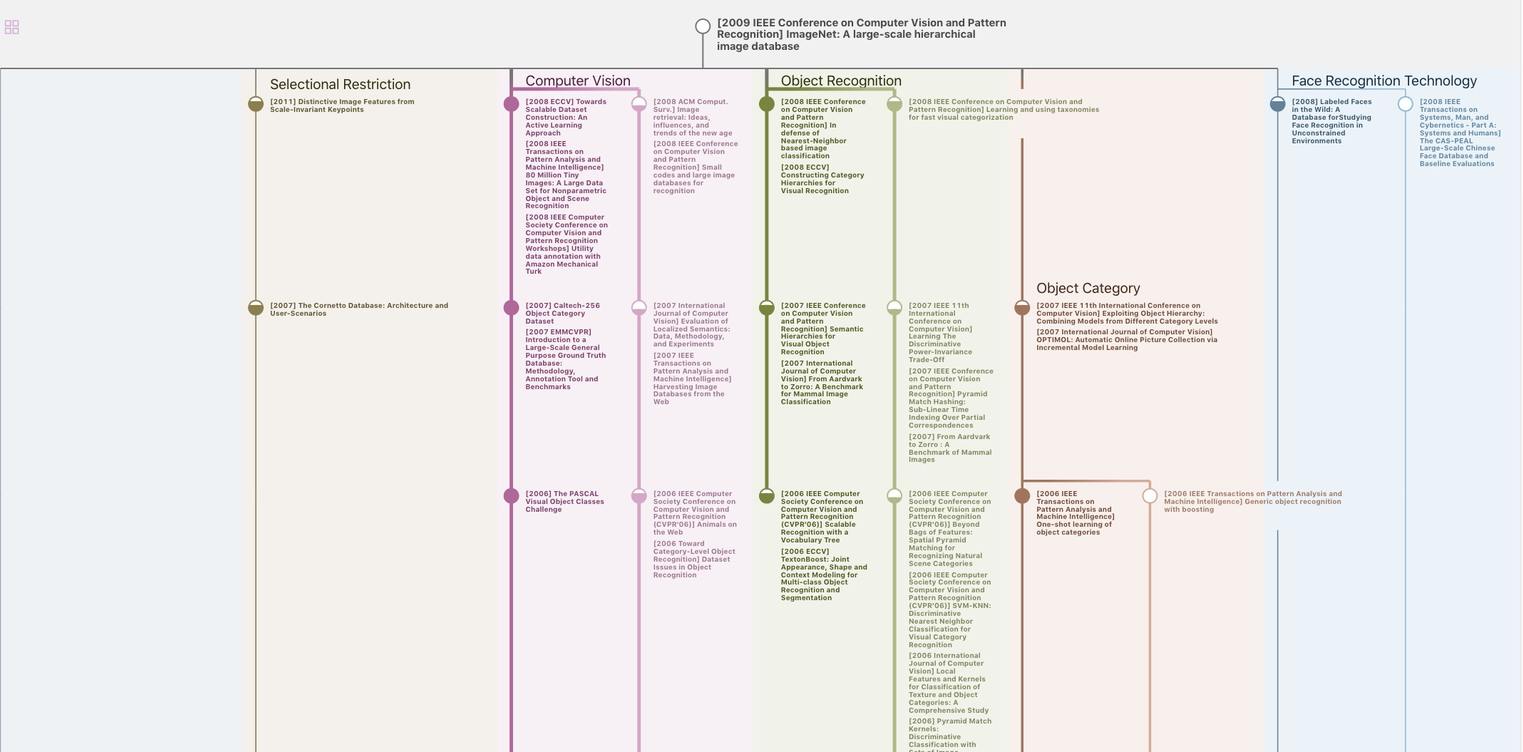
生成溯源树,研究论文发展脉络
Chat Paper
正在生成论文摘要