Automated freezing of gait assessment with marker-based motion capture and multi-stage graph convolutional neural networks approaches expert-level detection
arXiv (Cornell University)(2021)
摘要
Freezing of gait (FOG) is a common and debilitating gait impairment in Parkinson's disease. Further insight in this phenomenon is hampered by the difficulty to objectively assess FOG. To meet this clinical need, this paper proposes a motion capture-based FOG assessment method driven by a novel deep neural network. The proposed network, termed multi-stage graph convolutional network (MS-GCN), combines the spatial-temporal graph convolutional network (ST-GCN) and the multi-stage temporal convolutional network (MS-TCN). The ST-GCN captures the hierarchical motion among the optical markers inherent to motion capture, while the multi-stage component reduces over-segmentation errors by refining the predictions over multiple stages. The proposed model was validated on a dataset of fourteen freezers, fourteen non-freezers, and fourteen healthy control subjects. The experiments indicate that the proposed model outperforms state-of-the-art baselines. An in-depth quantitative and qualitative analysis demonstrates that the proposed model is able to achieve clinician-like FOG assessment. The proposed MS-GCN can provide an automated and objective alternative to labor-intensive clinician-based FOG assessment.
更多查看译文
关键词
gait assessment,motion capture,convolutional neural networks,freezing,marker-based,multi-stage,expert-level
AI 理解论文
溯源树
样例
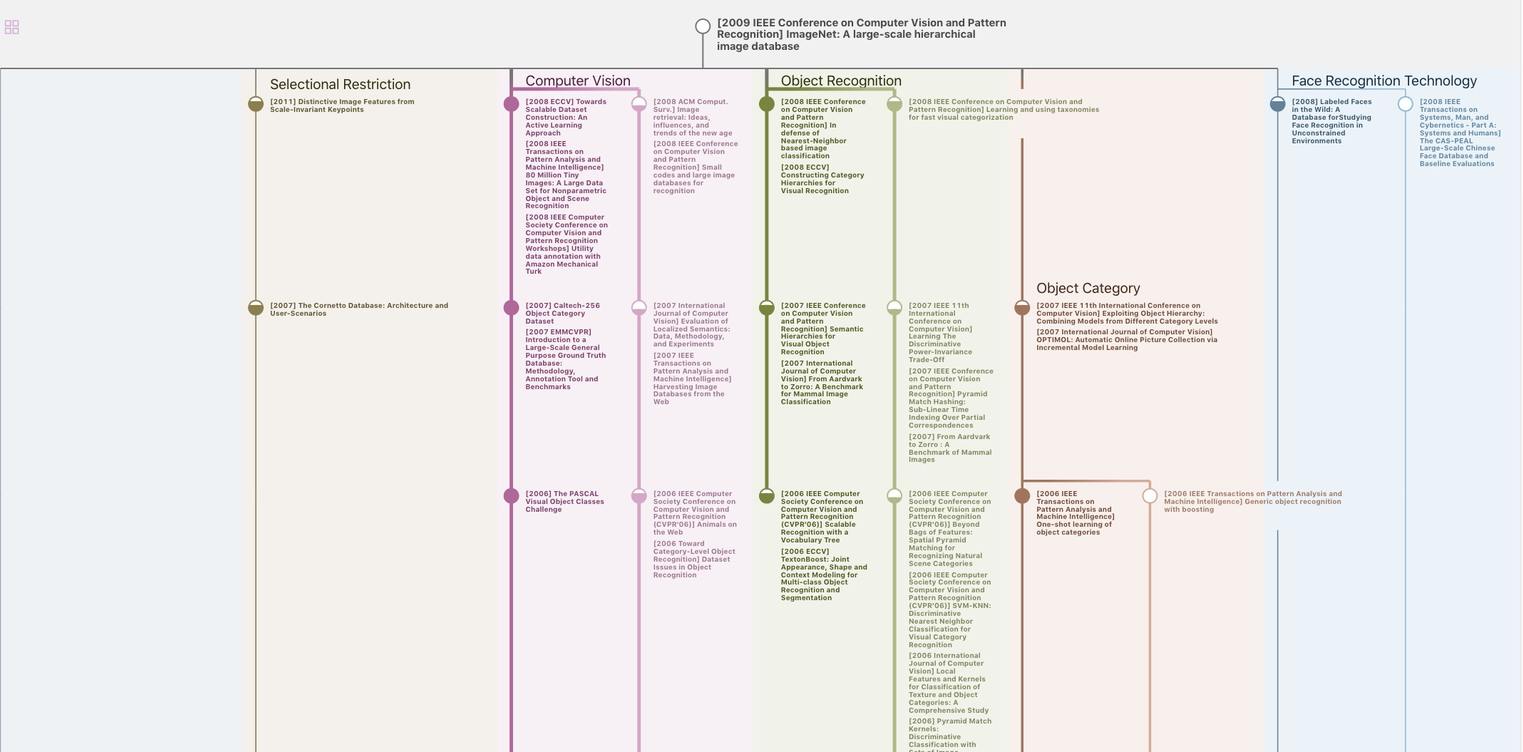
生成溯源树,研究论文发展脉络
Chat Paper
正在生成论文摘要