Performance of the SSM-iCrop model for predicting growth and nitrogen dynamics in winter wheat
European Journal of Agronomy(2022)
摘要
Process-based crop models are essential tools for representing the fundamental interactions between the cropping environment (weather, soil, and management) and plant development, growth, resource use, and yield formation. Due to these capabilities, crop models are considered as an integral component of smart farming tools for evaluating and improving crop management at field, farm, and regional scales. However, prior to application of a crop model in geospatial decision support tools, its robustness should be established by comparing model predictions with observations from the target cropping environment. The objective of this study was to assess the performance of the Simple Simulation Model (SSM-iCrop) for predicting growth and nitrogen (N) dynamics of winter wheat (Triticum aestivum) cultivars in a temperate environment. Detailed plant and soil data were collected from three field experiments conducted with four widely-grown cultivars under four N application rates in Austria. Variation in N fertilisation and differences in soil properties and weather conditions in the three field experiments generated a wide range of observed crop total dry mass (585–2034 g m−2), N uptake (5–32 g N m−2), and grain yield (211–898 g m−2). The SSM-iCrop model required parameterisation of a relatively small number of plant input parameters. As these parameters could be directly calculated from the experimental data, except for two phenology-related coefficients, there was no need for calibrating the model. In initial simulations, SSM-iCrop was not able to predict the response of leaf area index (LAI) to decreasing N supply. Introducing an additional parameter defining the minimum stem N concentration from emergence to begin grain growth improved the model performance substantially. The simulated time-course of crop attributes through the growing season showed good overall correspondence with observed data. Across the three field experiments, the model performed well in simulating above-ground dry mass (CV=5.9, RMSE=115.6 g N m−2), grain yield (CV=1.9, RMSE=60.5 g N m−2), total crop N uptake (CV=4.5, RMSE=1.9 g N m−2), and grain N content (CV=1.1; RMSE=2.2 g N m−2). Overall, The results of this study confirmed the robustness of SSM-iCrop for predicting wheat development, growth, N dynamics, and yield in the target cropping environments. The relatively simple structure and high degree of transparency make the SSM-iCrop suitable for integration in smart farming tools for improving tactical decision making in crop production. This study also highlights the essential role of high-quality detailed experimental data for adequate parameterisation and evaluation of crop models..
更多查看译文
关键词
Wheat,Modelling,Nitrogen,SSM model,Model parameterisation,Smart farming
AI 理解论文
溯源树
样例
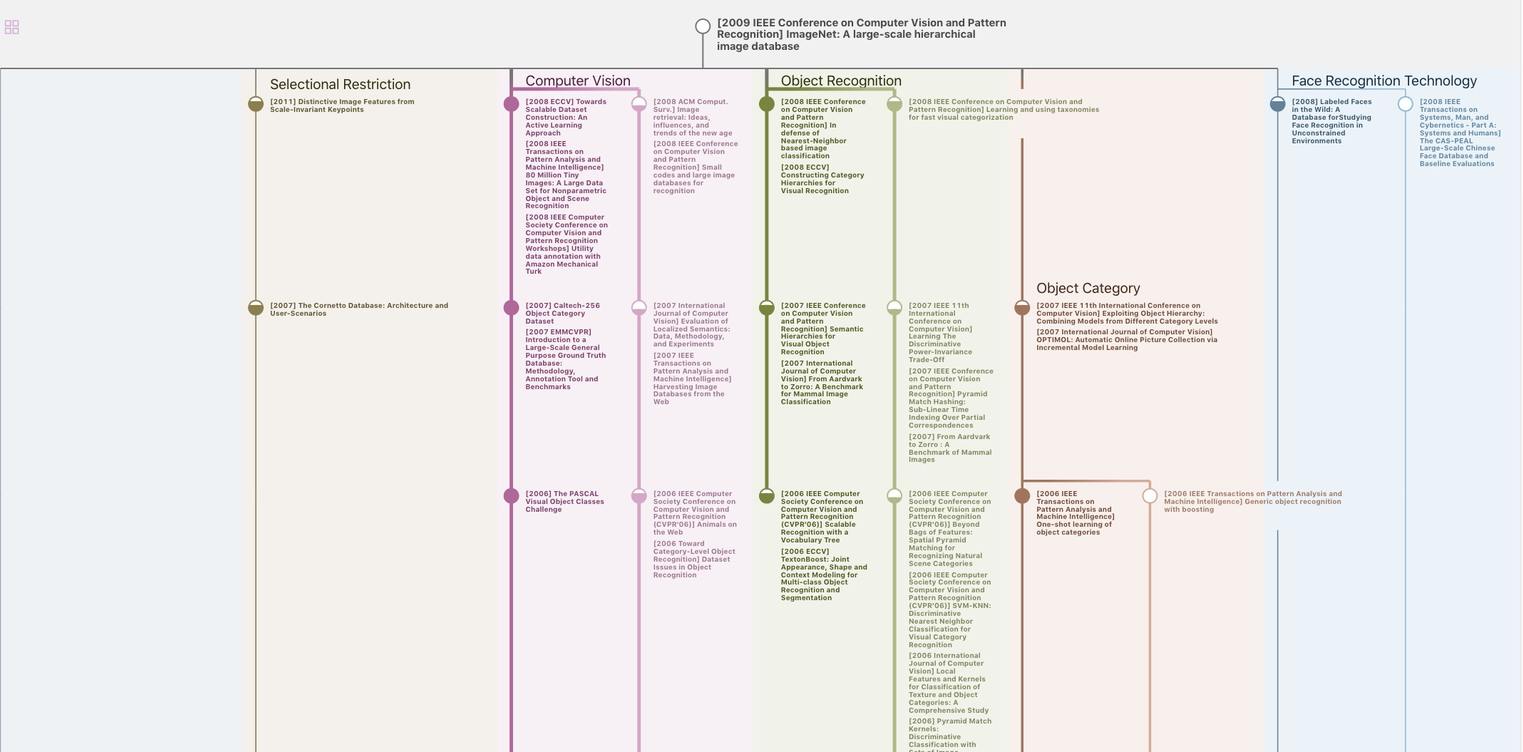
生成溯源树,研究论文发展脉络
Chat Paper
正在生成论文摘要