Bayesian inference by informative gaussian features of the data
semanticscholar(2021)
摘要
Sebastian Springer Bayesian inference by informative Gaussian features of the data Lappeenranta 2021 62 pages Acta Universitatis Lappeenrantaensis 950 Diss. Lappeenranta-Lahti University of Technology LUT ISBN 978-952-335-627-6, ISBN 978-952-335-628-3 (PDF), ISSN-L 1456-4491, ISSN 1456-4491 Given a set of measurements, a model can be calibrated to data by minimising a cost function that captures the model-data difference. The standard cost function for deterministic models is the residual sum of squares between the model output and the reference data. Sequential data assimilation methods can be used for stochastic models whenever it is possible to estimate the next state of the system from the current values. Chaotic dynamical systems are a specific type of models for which the classic approaches are often not available. Especially so, if the time gap between consecutive observations is higher than the predictable time interval. There is, therefore, a need for new methods for such problems, defined even as insolvable in the literature. In recent years, Haario et al. presented a solution to estimate the model parameters of chaotic systems by the so-called Correlation integral likelihood (CIL). The idea behind it is to use a generalisation of the correlation integral sum as a feature vector and build a Gaussian likelihood by estimating the variability from the repetitions of the experiment. In this work, we will further develop those ideas. We will first see how to generalise the CIL approach to use statistics that best fit the specific problem studied. Furthermore, we will see how it is possible to create different feature vectors from one or several data sets concerning the same phenomenon and combine them in a single likelihood to improve the estimation of the parameters. It will be shown how by using this method it is possible to estimate the parameters of chaotic systems when only the state vector is observed, or only a part of it, and when the measurements are arbitrarily sparse and scattered. We emphasise that the original distance-based CIL is by no means the only way to construct provably Gaussian feature vectors. Indeed, the selection of features to produce normally distributed empirical cumulative distribution function (eCDF) vectors should be carefully done case by case. Moreover, we will present tools to diagnose goodness-of-fit or a possible lack of fit after the posterior distribution has been obtained. To make our approach available also for cases with expensive forward models, we studied the combination with the Local Approximation MCMC (LA-MCMC) algorithms. The idea behind these types of sampling methods is to construct local polynomial approximations of the likelihood by regression over a set of neighbouring evaluations of the expensive likelihood, and make it more and more precise as the sampling proceeds. By this method, the number of expensive likelihood evaluations could be reduced by orders of magnitude without significantly impacting the precision of the resulting posterior distribution.
更多查看译文
AI 理解论文
溯源树
样例
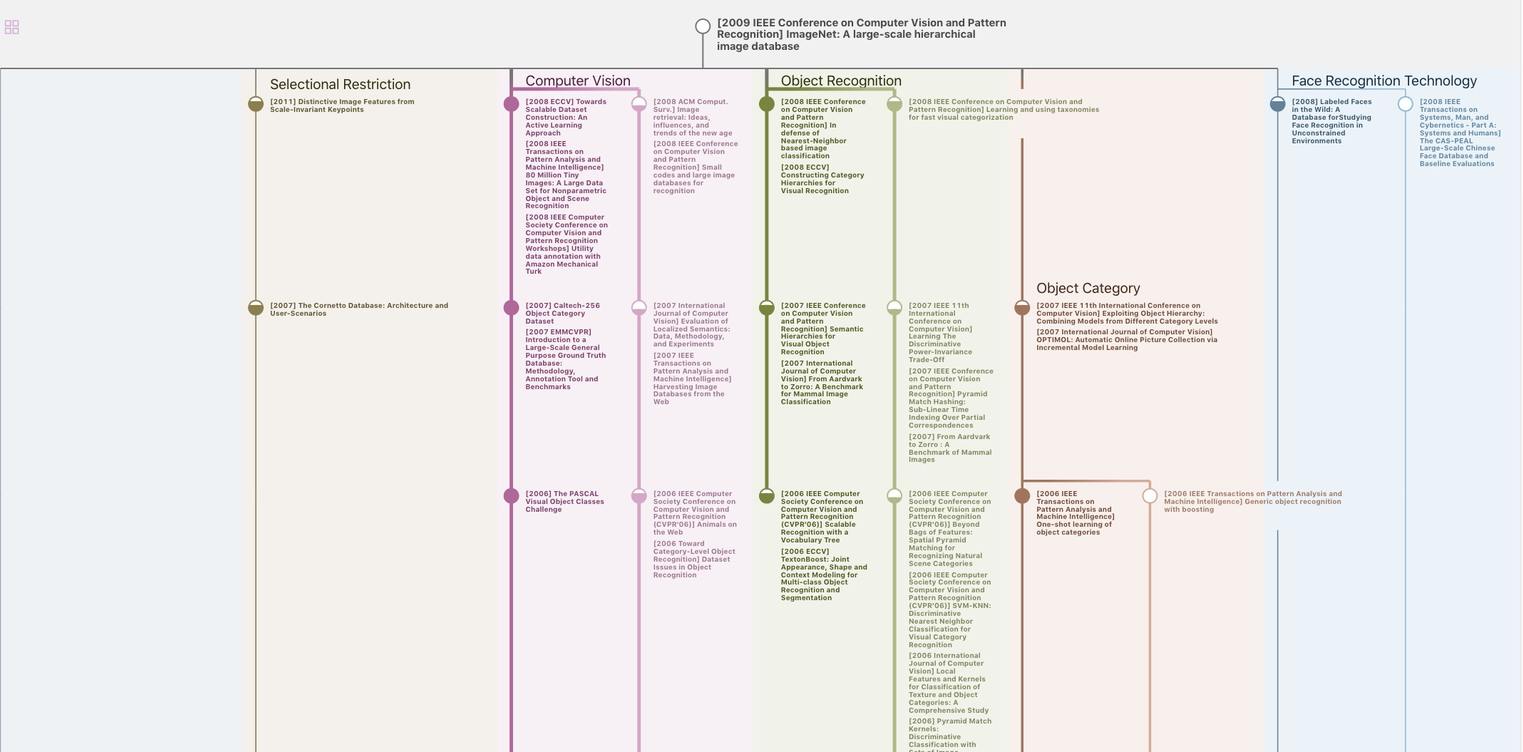
生成溯源树,研究论文发展脉络
Chat Paper
正在生成论文摘要