Decoupling GPGPU Voltage-Frequency Scaling for Deep-Learning Applications
Journal of parallel and distributed computing(2022)
摘要
The use of GPUs to accelerate DNN training and inference is already widely adopted, allowing for a significant performance increase. However, this performance is usually obtained at the cost of a consequent increase in energy consumption. While several solutions have been proposed to perform voltage-frequency scaling on GPUs, these are still one-dimensional, by simply adjusting the frequency while relying on default voltage settings. To overcome this limitation, this paper introduces a new methodology to fully characterize the impact of non-conventional DVFS on GPUs. The proposed approach was evaluated on two devices, an AMD Vega 10 Frontier Edition and an AMD Radeon 5700XT. When applying this non-conventional DVFS scheme to DNN training, the obtained results show that it is possible to safely decrease the GPU voltage, allowing for a significant reduction of the energy consumption (up to 38%) and of the EDP (up to 41%) on the training procedure of CNN models, with no degradation of the networks accuracy.
更多查看译文
关键词
GPU,DVFS,Undervoltage,Energy efficiency,CNN
AI 理解论文
溯源树
样例
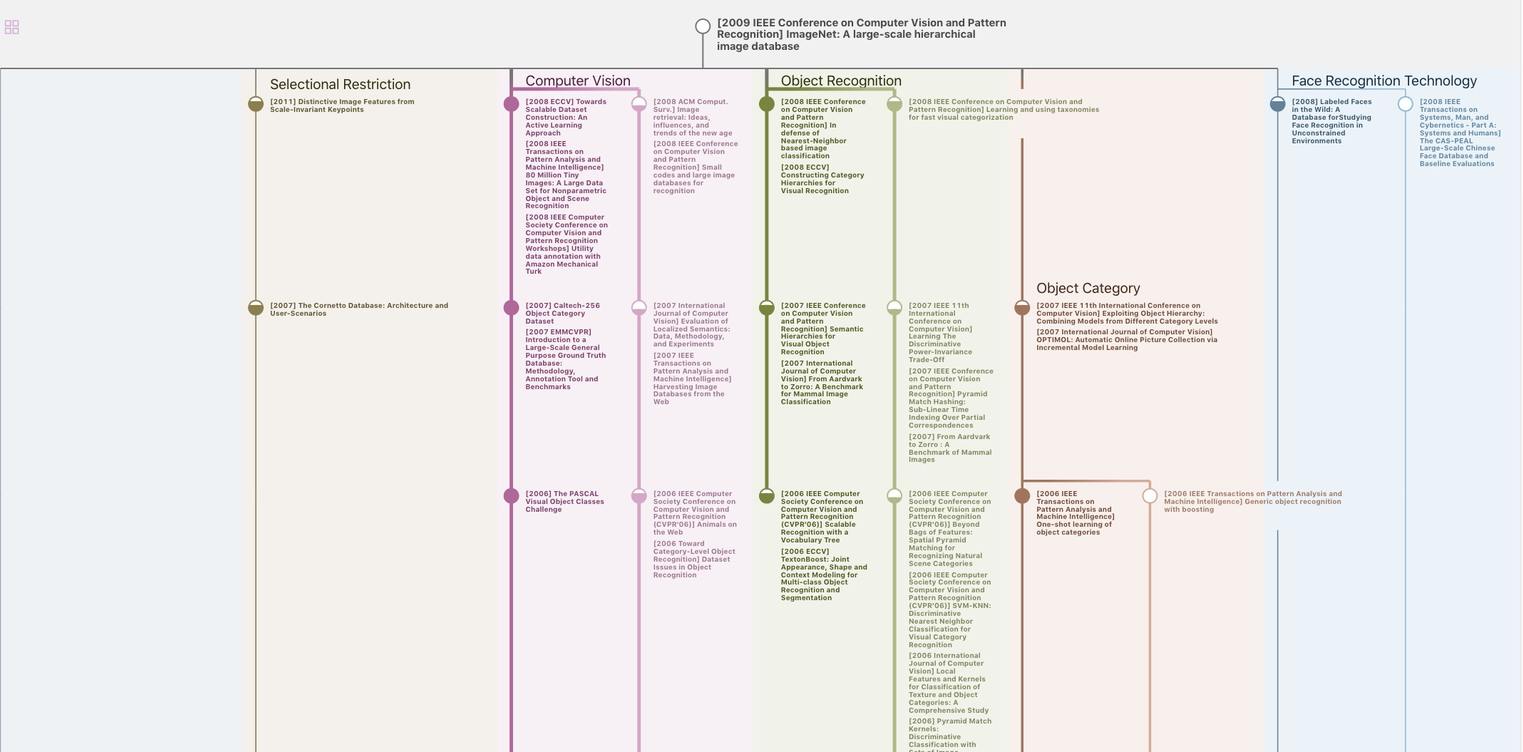
生成溯源树,研究论文发展脉络
Chat Paper
正在生成论文摘要