Automatic Classification of Tweets about Eating Disorders: Traditional Machine Learning techniques and BERT models (Preprint)
semanticscholar(2021)
摘要
BACKGROUND
Eating disorders (EDs) are diseases that affect an increasing number of people. Social networks provide information that can help to solve problems in this domain. In our study, we use artificial intelligence applied to social media data on eating disorders.
OBJECTIVE
We set out to find efficient models capable of categorizing tweets into four different binary categories in the EDs domain. We sought to obtain the most accurate model at the best computational cost.
METHODS
For three consecutive months, 1,058,957 tweets related to eating disorders were collected. The data were preprocessed and a subset of 2,000 tweets was labeled into four binary categories: (i) messages written by people suffering from EDs or not, (ii) messages promoting suffering from EDs or not, (iii) informative messages or not, and (iv) scientific or non-scientific messages. Following this, traditional machine learning and deep learning models capable of classifying text were applied to make use of this data.
RESULTS
F1-score of between 71.1% and 86.4% were obtained in the four categorizations, with the BERT models getting the best score from among the machine learning and deep learning techniques applied. In particular, RoBERTa and DistilBERT are the highest-scoring models.
CONCLUSIONS
The use of the machine and deep learning techniques for the classification of tweets related to eating disorders has shown promise in the field of detecting people who may suffer from some type of ED, as well as in three other categorizations. BERT models respond with better performance, although their computational cost is significantly higher than other traditional techniques.
CLINICALTRIAL
更多查看译文
AI 理解论文
溯源树
样例
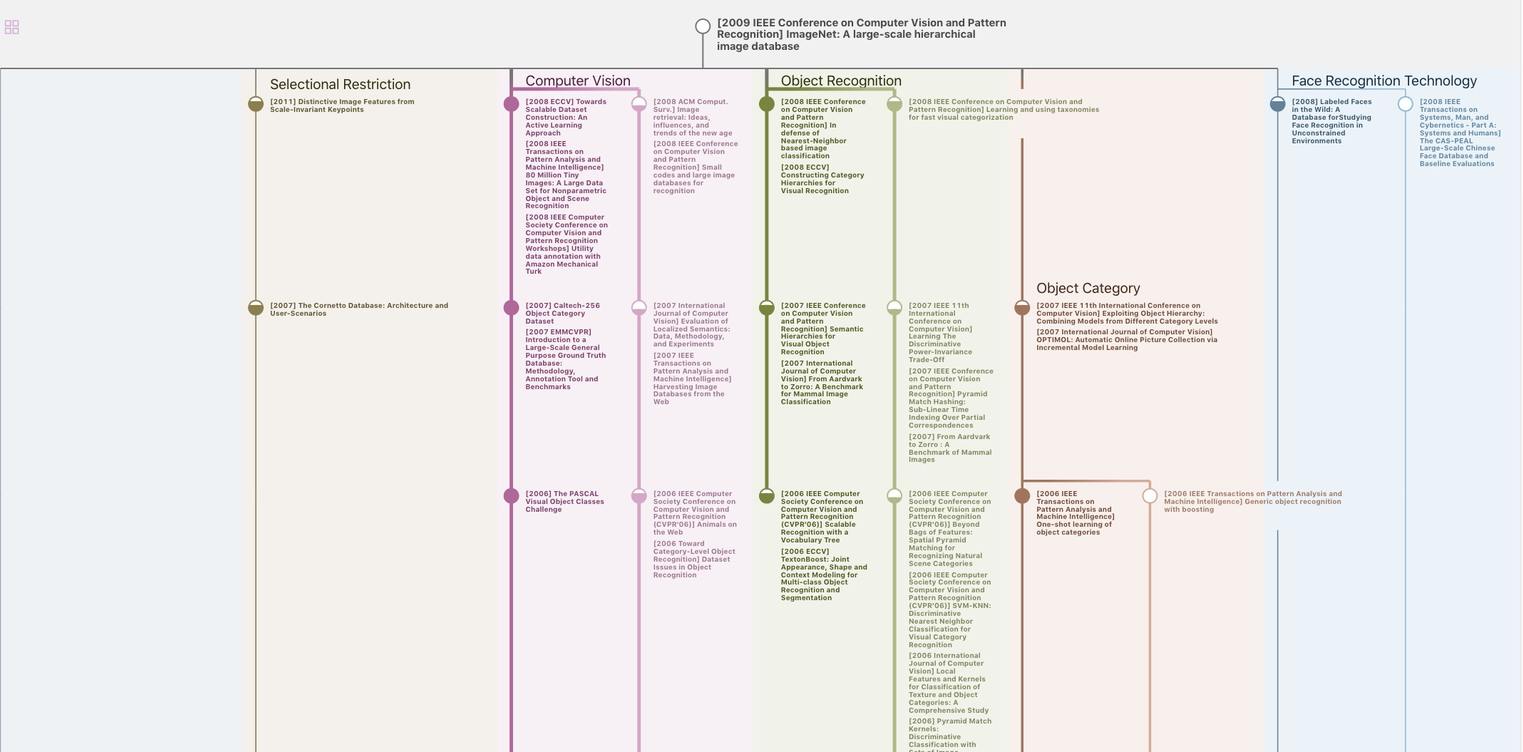
生成溯源树,研究论文发展脉络
Chat Paper
正在生成论文摘要