Rapid Discovery of Stable Materials by Coordinate-free Coarse Graining
Science Advances(2021)
摘要
A fundamental challenge in materials science pertains to elucidating the relationship between stoichiometry, stability, structure, and property. Recent advances have shown that machine learning can be used to learn such relationships, allowing the stability and functional properties of materials to be accurately predicted. However, most of these approaches use atomic coordinates as input and are thus bottlenecked by crystal structure identification when investigating novel materials. Our approach solves this bottleneck by coarse-graining the infinite search space of atomic coordinates into a combinatorially enumerable search space. The key idea is to use Wyckoff representations – coordinate-free sets of symmetry-related positions in a crystal – as the input to a machine learning model. Our model demonstrates exceptionally high precision in discovering new theoretically stable materials, identifying 1,569 materials that lie below the known convex hull of previously calculated materials from just 5,675 ab-initio calculations. Our approach opens up fundamental advances in computational materials discovery.
更多查看译文
AI 理解论文
溯源树
样例
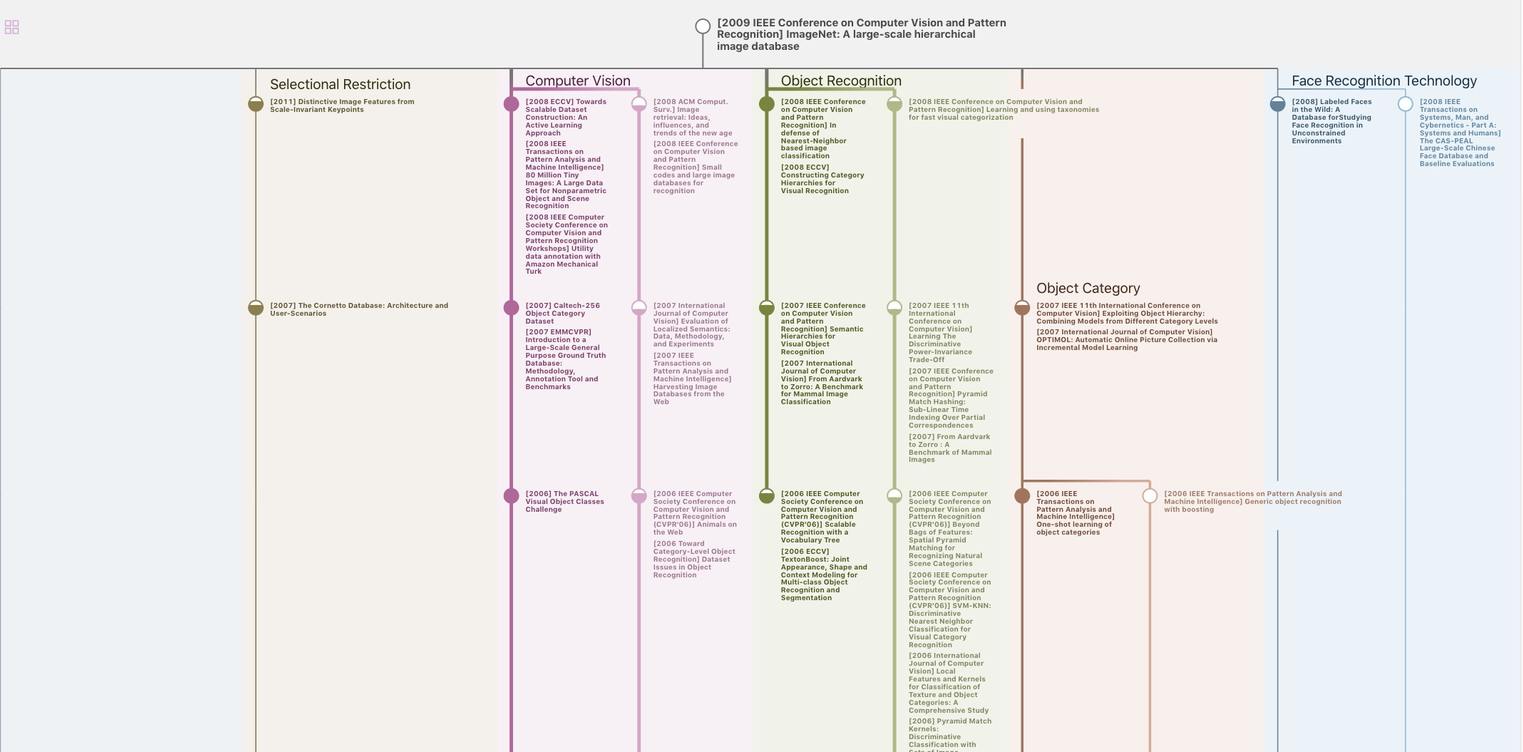
生成溯源树,研究论文发展脉络
Chat Paper
正在生成论文摘要