Time-Variance Aware Dynamic Kernel Generation for Real-Time Acoustic Echo Cancellation
IEEE SIGNAL PROCESSING LETTERS(2022)
摘要
Time-variant factors including dynamic delay and varying echo path often occur in real-world acoustic echo cancellation (AEC) applications. Current end-to-end deep neural network (DNN) based methods usually model the time-variant components implicitly and can hardly handle the unpredictable time-variance in real-time AEC. To explicitly capture the time-variant components, we propose a dynamic kernel generation (DKG) module that can be introduced as a learnable plug-in to a DNN-based end-to-end pipeline. Specifically, the DKG module generates a convolutional kernel regarding to each input audio frame, so that the DNN model is able to dynamically adjust its weights according to the input signal during inference. Experimental results verify that DKG module improves the AEC performance of the model under time-variant scenarios, especially in the double-talk cases.
更多查看译文
关键词
Kernel,Convolution,Microphones,Decoding,Delays,Adaptation models,Real-time systems,Acoustic echo cancellation,time-variance,dynamic kernel generation,deep neural network
AI 理解论文
溯源树
样例
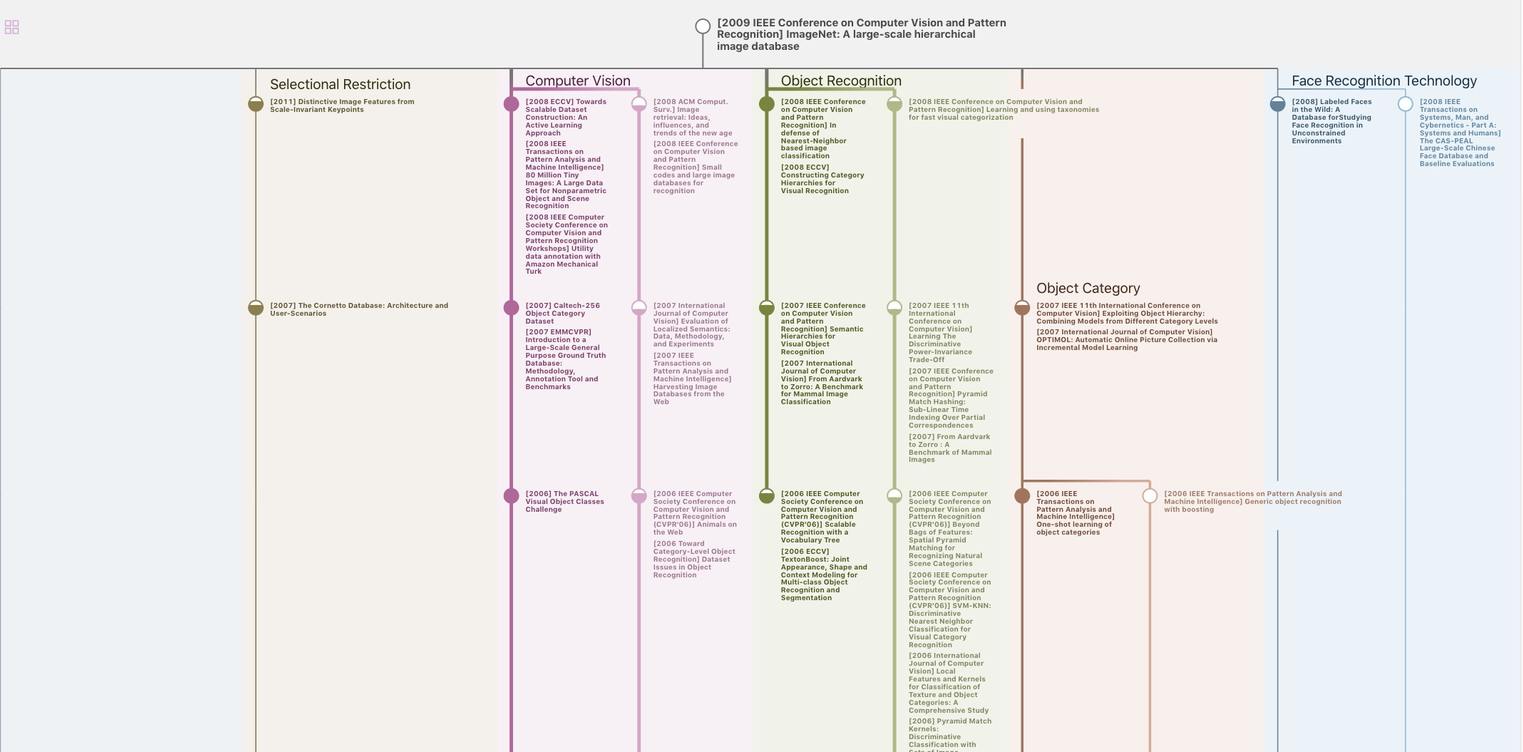
生成溯源树,研究论文发展脉络
Chat Paper
正在生成论文摘要