Adaptive Evolution Strategy with Ensemble of Mutations for Reinforcement Learning
Knowledge-Based Systems(2022)CCF CSCI 1区
Abstract
Evolving the weights of learning networks through evolutionary computation (neuroevolution) has proven scalable over a range of challenging Reinforcement Learning (RL) control tasks. However, similar to most black-box optimization problems, existing neuroevolution approaches require an additional adaptation process to effectively balance exploration and exploitation through the selection of sensitive hyper-parameters throughout the evolution process. Therefore, these methods are often plagued by the computation complexities of such adaptation processes which often rely on a number of sophisticatedly formulated strategy parameters. In this paper, Evolution Strategy (ES) with a simple yet efficient ensemble of mutation strategies is proposed. Specifically, two distinct mutation strategies coexist throughout the evolution process where each strategy is associated with its own population subset. Consequently, elites for generating a population of offspring are realized by co-evaluation of the combined population. Experiments on testbed of six (6) black-box optimization problems which are generated using a classical control problem and six (6) proven continuous RL agents demonstrate the efficiency of the proposed method in terms of faster convergence and scalability than the canonical ES. Furthermore, the proposed Adaptive Ensemble ES (AEES) shows an average of 5 - 10000x and 10 - 100x better sample complexity in low and high dimension problems, respectively than their associated base DRL agents.
MoreTranslated text
Key words
Evolution strategy,Reinforcement Learning,Ensemble,Mutation strategy,Black-box optimization
PDF
View via Publisher
AI Read Science
AI Summary
AI Summary is the key point extracted automatically understanding the full text of the paper, including the background, methods, results, conclusions, icons and other key content, so that you can get the outline of the paper at a glance.
Example
Background
Key content
Introduction
Methods
Results
Related work
Fund
Key content
- Pretraining has recently greatly promoted the development of natural language processing (NLP)
- We show that M6 outperforms the baselines in multimodal downstream tasks, and the large M6 with 10 parameters can reach a better performance
- We propose a method called M6 that is able to process information of multiple modalities and perform both single-modal and cross-modal understanding and generation
- The model is scaled to large model with 10 billion parameters with sophisticated deployment, and the 10 -parameter M6-large is the largest pretrained model in Chinese
- Experimental results show that our proposed M6 outperforms the baseline in a number of downstream tasks concerning both single modality and multiple modalities We will continue the pretraining of extremely large models by increasing data to explore the limit of its performance
Try using models to generate summary,it takes about 60s
Must-Reading Tree
Example
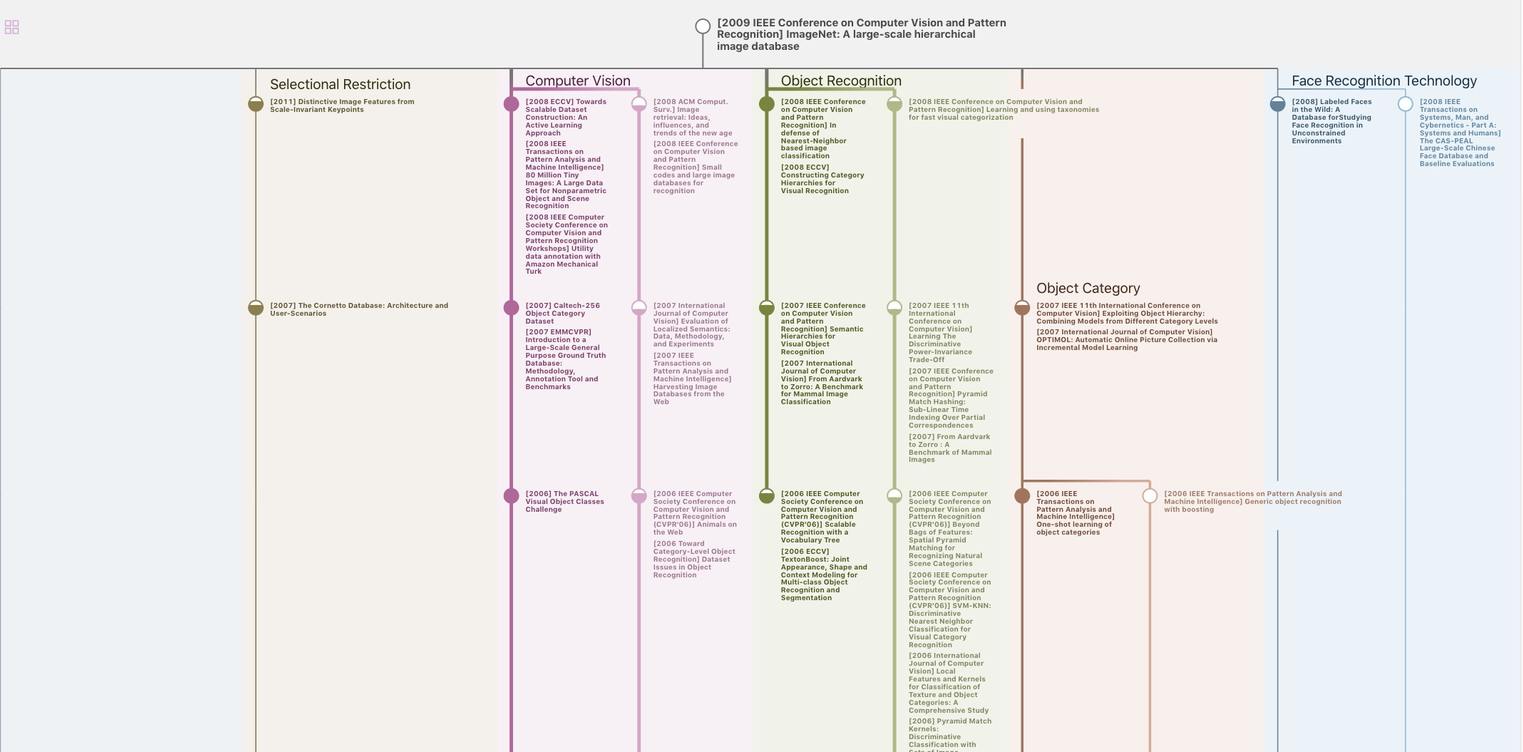
Generate MRT to find the research sequence of this paper
Related Papers
Deep Reinforcement Learning Versus Evolution Strategies: A Comparative Survey
IEEE TRANSACTIONS ON NEURAL NETWORKS AND LEARNING SYSTEMS 2024
被引用15
Automatic Frequency-Based Feature Selection Using Discrete Weighted Evolution Strategy.
Applied Soft Computing 2022
被引用9
Optimization of Large-Scale UAV Cluster Confrontation Game Based on Integrated Evolution Strategy
CLUSTER COMPUTING-THE JOURNAL OF NETWORKS SOFTWARE TOOLS AND APPLICATIONS 2024
被引用4
SWARM AND EVOLUTIONARY COMPUTATION 2023
被引用6
BiERL: A Meta Evolutionary Reinforcement Learning Framework Via Bilevel Optimization.
ECAI 2023 2023
被引用0
IEEE ACCESS 2023
被引用2
Gaussian Adaptation with Decaying Matrix Adaptation Weights
2023 IEEE Congress on Evolutionary Computation (CEC) 2023
被引用0
MEEDNets: Medical Image Classification Via Ensemble Bio-inspired Evolutionary DenseNets
KNOWLEDGE-BASED SYSTEMS 2023
被引用11
Evolutionary Machine Learning in Control
Handbook of Evolutionary Machine Learning Genetic and Evolutionary Computation 2023
被引用1
EXPERT SYSTEMS WITH APPLICATIONS 2024
被引用4
An Optimal Re-parametrization Scheme for Generalization in Reinforcement Learning
2023 14th International Conference on Information and Communication Technology Convergence (ICTC) 2023
被引用0
FACTA UNIVERSITATIS-SERIES MECHANICAL ENGINEERING 2023
被引用6
EXPERT SYSTEMS WITH APPLICATIONS 2024
被引用0
APPLIED SOFT COMPUTING 2024
被引用0
Swarm and Evolutionary Computation 2024
被引用0
Data Disclaimer
The page data are from open Internet sources, cooperative publishers and automatic analysis results through AI technology. We do not make any commitments and guarantees for the validity, accuracy, correctness, reliability, completeness and timeliness of the page data. If you have any questions, please contact us by email: report@aminer.cn
Chat Paper
去 AI 文献库 对话