slo-nns: Service Level Objective-Aware Neural Networks
semanticscholar(2022)
摘要
Machine learning (ML) inference is a real-time workload that must comply with strict Service Level Objectives (SLOs), including latency and accuracy targets. Unfortunately, ensuring that SLOs are not violated in inference-serving systems is challenging due to inherent model accuracy-latency tradeoffs, SLO diversity across and within application domains, evolution of SLOs over time, unpredictable query patterns, and co-location interference. In this paper, we observe that neural networks exhibit high degrees of per-input activation sparsity during inference. . Thus, we propose SLO-Aware Neural Networks (slo-nns) which dynamically drop out nodes per-inference query, thereby tuning the amount of computation performed, according to specified SLO optimization targets and machine utilization. slo-nns achieve average speedups of 1.3 − 56.7× with little to no accuracy loss (less than 0.3%). When accuracy constrained, slo-nns are able to serve a range of accuracy targets at low latency with the same trained model. When latency constrained, slo-nns can proactively alleviate latency degradation from co-location interference while maintaining high accuracy to meet latency constraints.
更多查看译文
AI 理解论文
溯源树
样例
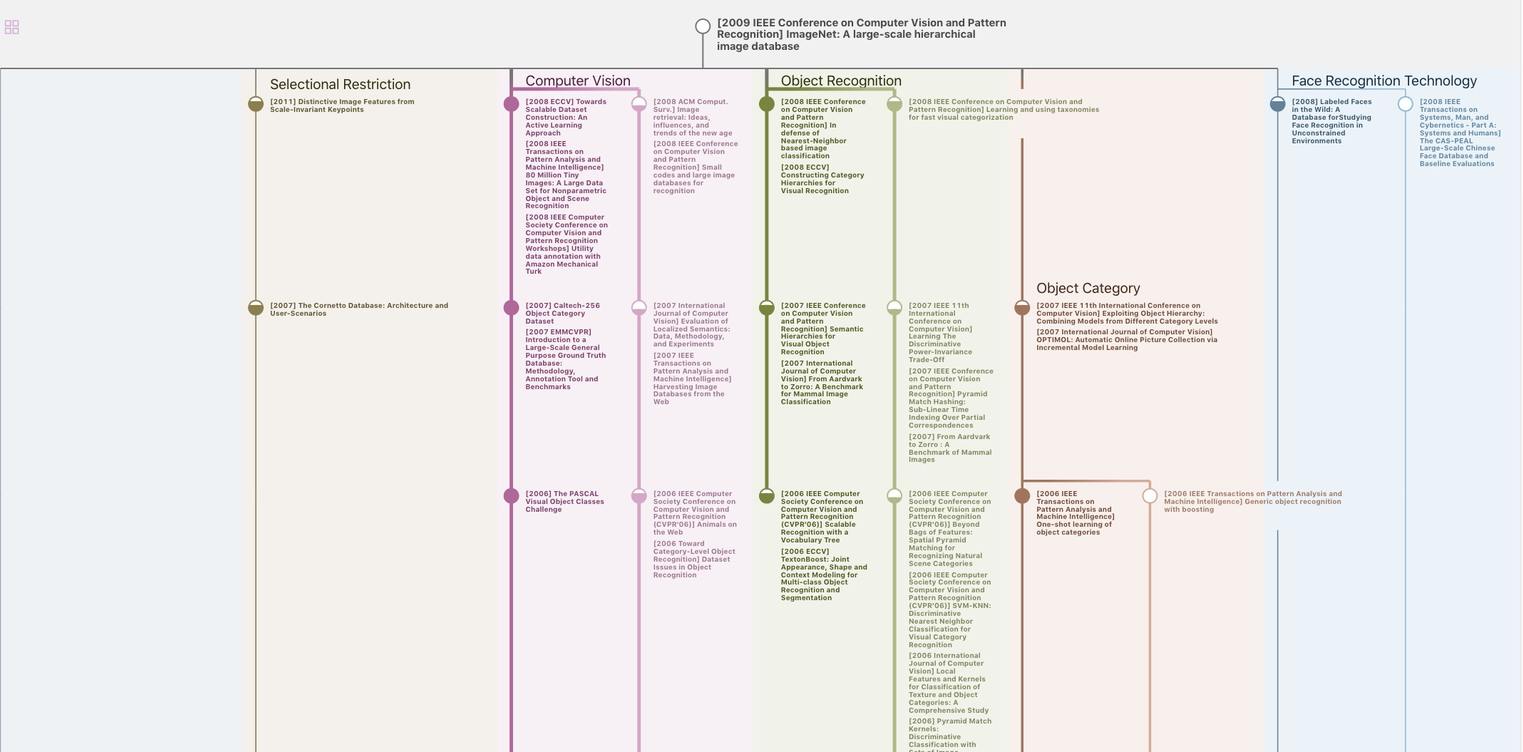
生成溯源树,研究论文发展脉络
Chat Paper
正在生成论文摘要