The Optimal Phenological Phase of Maize for Yield Prediction with High-Frequency UAV Remote Sensing
REMOTE SENSING(2022)
摘要
Unmanned aerial vehicle (UAV)-based multispectral remote sensing effectively monitors agro-ecosystem functioning and predicts crop yield. However, the timing of the remote sensing field campaigns can profoundly impact the accuracy of yield predictions. Little is known on the effects of phenological phases on skills of high-frequency sensing observations used to predict maize yield. It is also unclear how much improvement can be gained using multi-temporal compared to mono-temporal data. We used a systematic scheme to address those gaps employing UAV multispectral observations at nine development stages of maize (from second-leaf to maturity). Next, the spectral and texture indices calculated from the mono-temporal and multi-temporal UAV images were fed into the Random Forest model for yield prediction. Our results indicated that multi-temporal UAV data could remarkably enhance the yield prediction accuracy compared with mono-temporal UAV data (R-2 increased by 8.1% and RMSE decreased by 27.4%). For single temporal UAV observation, the fourteenth-leaf stage was the earliest suitable time and the milking stage was the optimal observing time to estimate grain yield. For multi-temporal UAV data, the combination of tasseling, silking, milking, and dough stages exhibited the highest yield prediction accuracy (R-2 = 0.93, RMSE = 0.77 t center dot ha(-1)). Furthermore, we found that the Normalized Difference Red Edge Index (NDRE), Green Normalized Difference Vegetation Index (GNDVI), and dissimilarity of the near-infrared image at milking stage were the most promising feature variables for maize yield prediction.
更多查看译文
关键词
unmanned aerial vehicle,remote sensing,maize,yield,multispectral
AI 理解论文
溯源树
样例
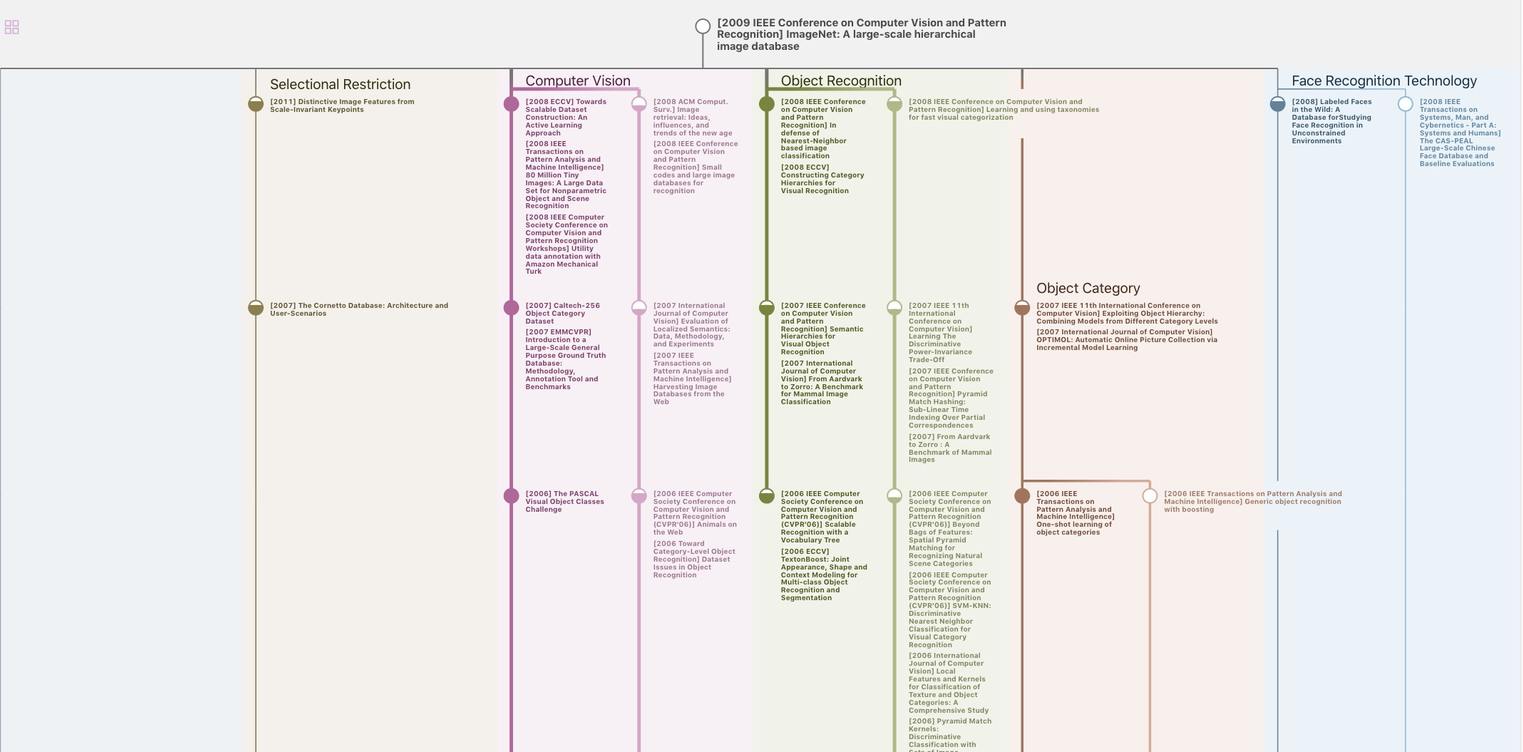
生成溯源树,研究论文发展脉络
Chat Paper
正在生成论文摘要