Stronger symbolic summary statistics for the LHC
semanticscholar(2021)
摘要
Analyzing the high-dimensional data collected at the Large Hadron Collider experiments often requires a balance between maximizing sensitivity and maintaining interpretability by domain experts. We propose a new algorithm to construct powerful summary statistics for LHC processes in the form of simple symbolic expressions. First, we extract latent information from a chain of simulators; through symbolic regression on this data we then learn approximately sufficient statistics. Observables constructed in this way can be used as plug-in replacements for established summary statistics, potentially improving the precision of scientific results without adding any overhead. In Higgs production in weak boson fusion, our algorithm rediscovers well-known heuristics and proposes new, moderately complex formulas that rival the new physics reach of neural networks.
更多查看译文
AI 理解论文
溯源树
样例
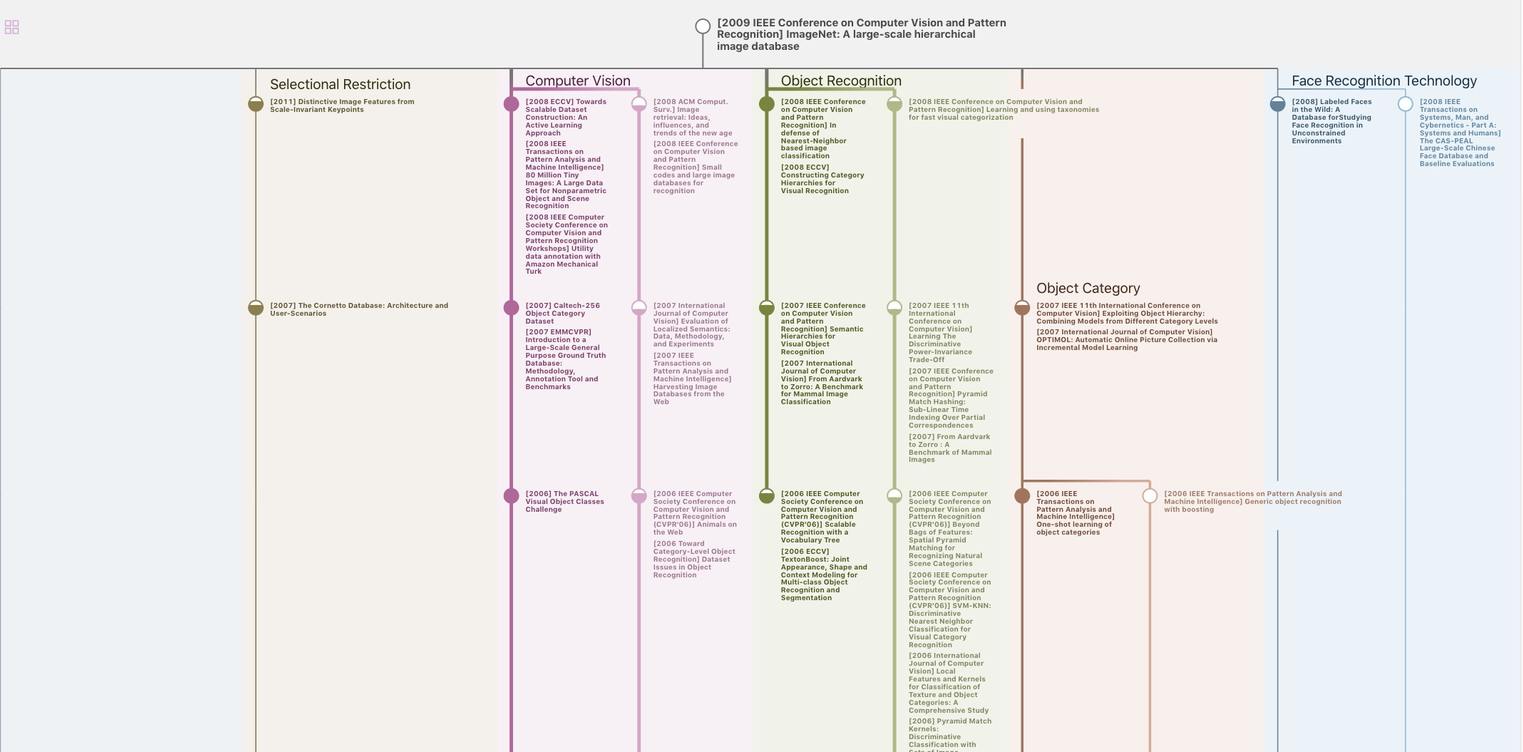
生成溯源树,研究论文发展脉络
Chat Paper
正在生成论文摘要