Machine Learning Methods for Predicting Long-term Mortality in Patients after Cardiac Surgery
FRONTIERS IN CARDIOVASCULAR MEDICINE(2021)
摘要
Background
Establishing a mortality prediction model of patients undergoing cardiac surgery might be useful for clinicians for alerting, judgment, and intervention, while few predictive tools for long-term mortality have been developed targeting patients post-cardiac surgery.
Objective
We aimed to construct and validate several machine learning (ML) algorithms to predict long-term mortality and identify risk factors in unselected patients after cardiac surgery during a 4-year follow-up.
Methods
The Medical Information Mart for Intensive Care (MIMIC-III) database was used to perform a retrospective administrative database study. Candidate predictors consisted of the demographics, comorbidity, vital signs, laboratory test results, prognostic scoring systems, and treatment information on the first day of ICU admission. 4-year mortality was set as the study outcome. We used the ML methods of logistic regression (LR), artificial neural network (NNET), naïve bayes (NB), gradient boosting machine (GBM), adapting boosting (Ada), random forest (RF), bagged trees (BT), and eXtreme Gradient Boosting (XGB). The prognostic capacity and clinical utility of these ML models were compared using the area under the receiver operating characteristic curves (AUC), calibration curves, and decision curve analysis (DCA).
Results
Of 7,368 patients in MIMIC-III included in the final cohort, a total of 1,337 (18.15%) patients died during a 4-year follow-up. Among 65 variables extracted from the database, a total of 25 predictors were selected using recursive feature elimination (RFE) and included in the subsequent analysis. The Ada model performed best among eight models in both discriminatory ability with the highest AUC of 0.801 and goodness of fit (visualized by calibration curve). Moreover, the DCA shows that the net benefit of the RF, Ada, and BT models surpassed that of other ML models for almost all threshold probability values. Additionally, through the Ada technique, we determined that red blood cell distribution width (RDW), blood urea nitrogen (BUN), SAPS II, anion gap (AG), age, urine output, chloride, creatinine, congestive heart failure, and SOFA were the Top 10 predictors in the feature importance rankings.
Conclusions
The Ada model performs best in predicting long-term mortality after cardiac surgery among the eight ML models. The ML-based algorithms might have significant application in the development of early warning systems for patients following operations.
更多查看译文
关键词
prediction model, machine learning, cardiac surgery, intensive care unit, long-term mortality, MIMIC-III database
AI 理解论文
溯源树
样例
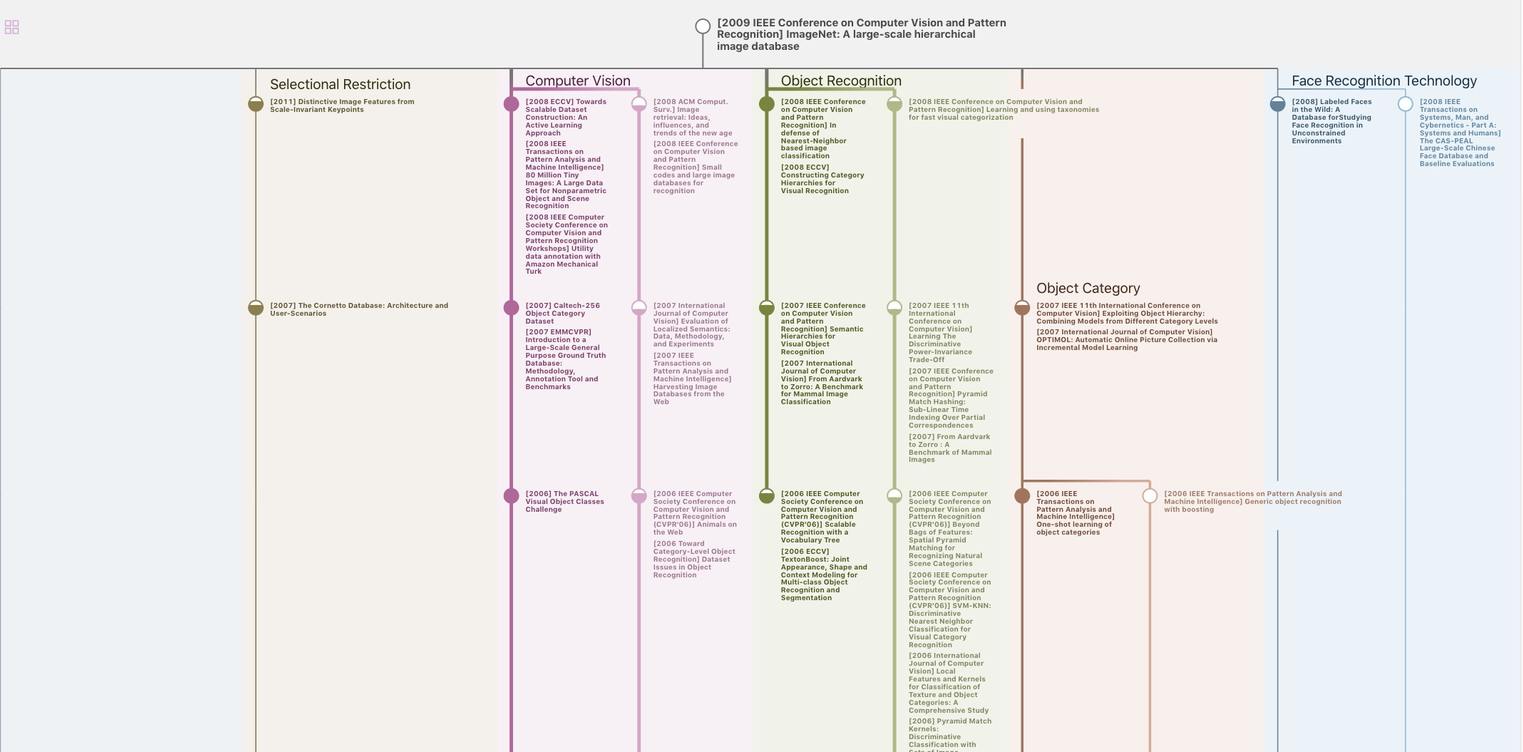
生成溯源树,研究论文发展脉络
Chat Paper
正在生成论文摘要