FELIX: A Ferroelectric FET Based Low Power Mixed-Signal In-Memory Architecture for DNN Acceleration.
ACM Trans. Embed. Comput. Syst.(2022)
摘要
AbstractToday, a large number of applications depend on deep neural networks (DNN) to process data and perform complicated tasks at restricted power and latency specifications. Therefore, processing-in-memory (PIM) platforms are actively explored as a promising approach to improve the throughput and the energy efficiency of DNN computing systems. Several PIM architectures adopt resistive non-volatile memories as their main unit to build crossbar-based accelerators for DNN inference. However, these structures suffer from several drawbacks such as reliability, low accuracy, large ADCs/DACs power consumption and area, high write energy, and so on. In this article, we present a new mixed-signal in-memory architecture based on the bit-decomposition of the multiply and accumulate (MAC) operations. Our in-memory inference architecture uses a single FeFET as a non-volatile memory cell. Compared to the prior work, this system architecture provides a high level of parallelism while using only 3-bit ADCs. Also, it eliminates the need for any DAC. In addition, we provide flexibility and a very high utilization efficiency even for varying tasks and loads. Simulations demonstrate that we outperform state-of-the-art efficiencies with 36.5 TOPS/W and can pack 2.05 TOPS with 8-bit activation and 4-bit weight precision in an area of 4.9 mm2 using 22 nm FDSOI technology. Employing binary operation, we obtain 1169 TOPS/W and over 261 TOPS/W/mm2 on system level.
更多查看译文
关键词
FeFET crossbar array,in-memory computation,convolution neural networks,mixed-signal processing,bit-decomposition,current-mode ADC
AI 理解论文
溯源树
样例
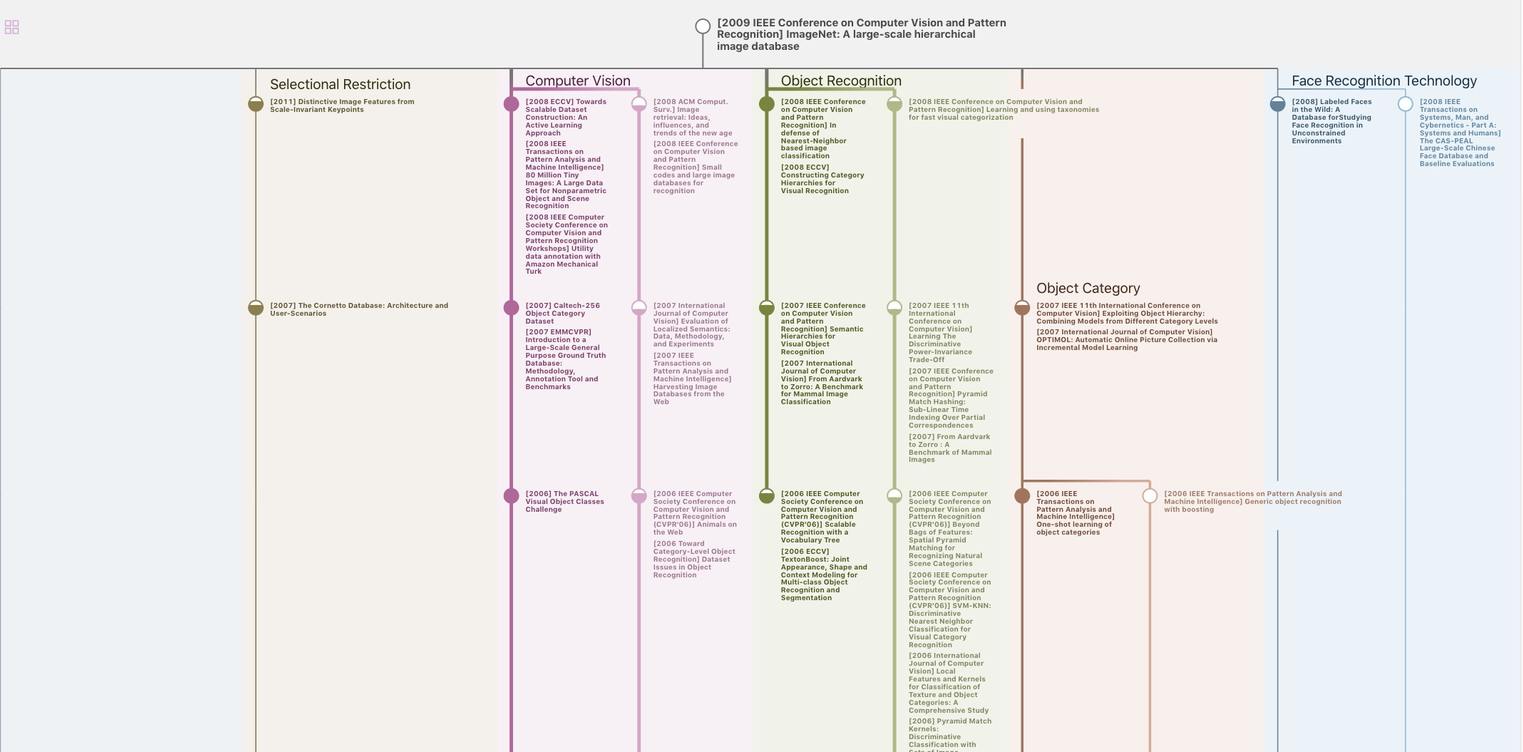
生成溯源树,研究论文发展脉络
Chat Paper
正在生成论文摘要