A Survey on Safety-Critical Scenario Generation for Autonomous Driving – A Methodological Perspective
semanticscholar(2022)
摘要
Autonomous driving systems have witnessed a great development during the past years thanks to the advance in sensing and decision-making. One critical obstacle for their massive deployment in the real world is the evaluation of safety. Most existing driving systems are still trained and evaluated on naturalistic scenarios that account for the vast majority of daily life or heuristically-generated adversarial ones. However, the large population of cars requires an extremely low collision rate, indicating safety-critical scenarios collected in the real world would be rare. Thus, methods to artificially generate artificial scenarios becomes critical to manage the risk and reduce the cost. In this survey, we focus on the algorithms of safety-critical scenario generation. We first provide a comprehensive taxonomy of existing algorithms by dividing them into three categories: data-driven generation, adversarial generation, and knowledge-based generation. Then, we discuss useful tools for scenario generation, including simulation platforms and packages. Finally, we extend our discussion to five main challenges of current works – fidelity, efficiency, diversity, transferability, controllability – and the research opportunities lighted up by these challenges.
更多查看译文
AI 理解论文
溯源树
样例
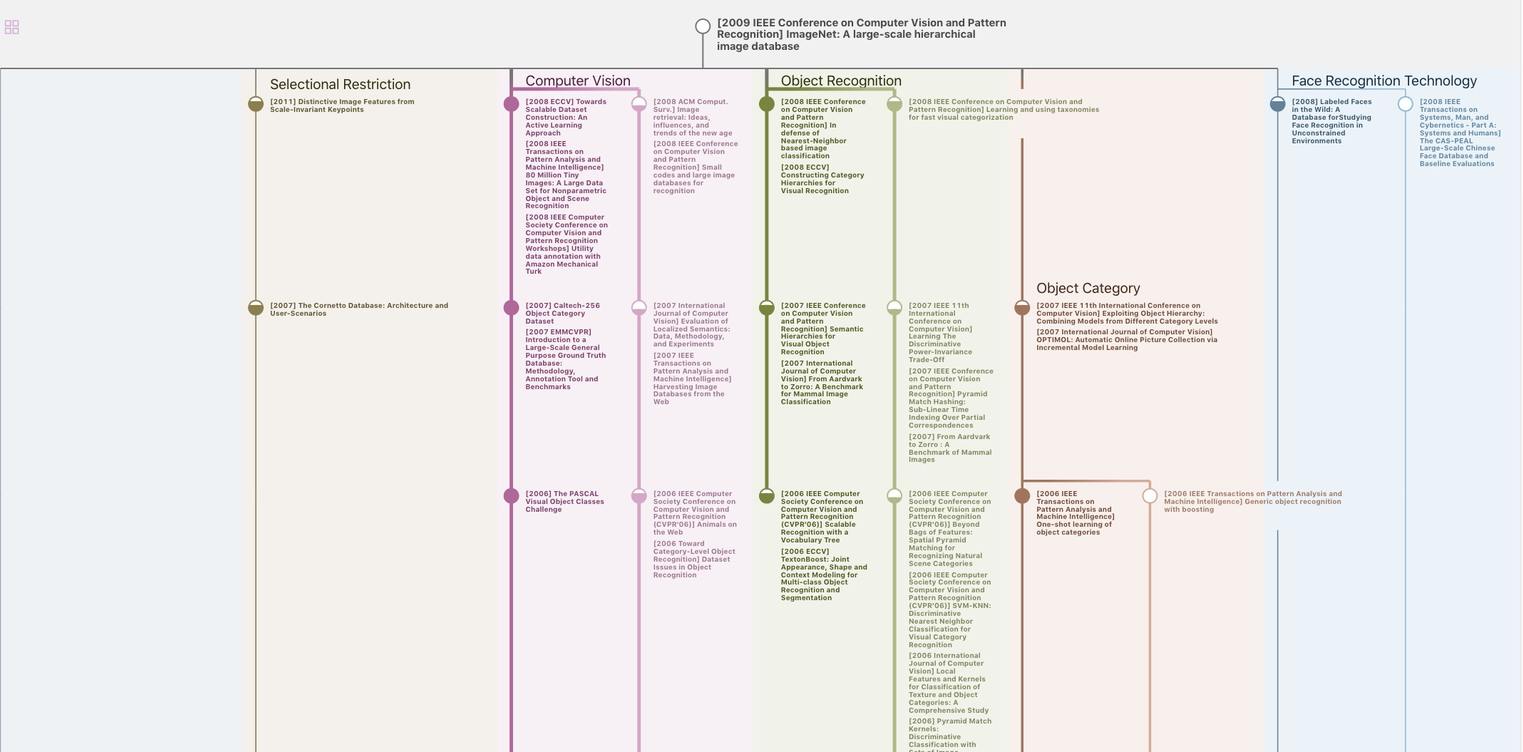
生成溯源树,研究论文发展脉络
Chat Paper
正在生成论文摘要