Training-Induced Class Imbalance in Crowdsourced Data
CSW@VLDB(2021)
摘要
In this paper, we examine how the design of data-collection systems can lead to imbalanced data. Specifically, we scrutinize how training affects the imbalance of data in a data crowdsourcing experiment. We randomly assigned contributors to explicitly trained, implicitly trained, and untrained (control) groups and asked them to report artificial insect sightings in a simulated crowdsourcing task. We posit that training contributors can lead them to selectively pay attention to and report specific aspects of observations while ignoring others. In the experiment, explicitly trained contributors reported less balanced data than untrained and implicitly trained contributors did. We then explored the effect of training-induced imbalance on an unsupervised classification task and found that the purity of classes formed was lower for explicitly trained contributors than for the other two types of contributors. We conclude by discussing the implications of artificial imbalance for the usefulness and insightfulness of crowdsourced data.
更多查看译文
AI 理解论文
溯源树
样例
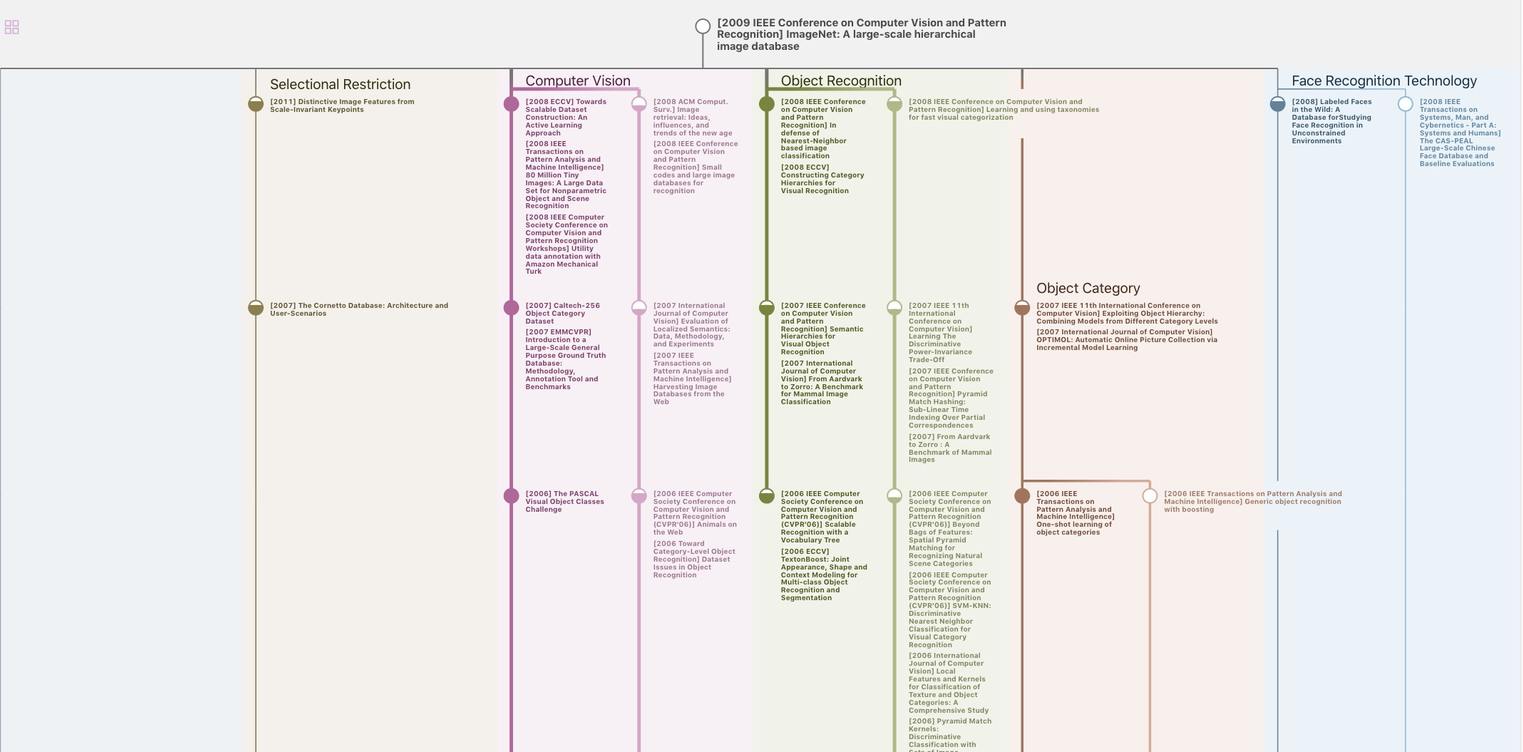
生成溯源树,研究论文发展脉络
Chat Paper
正在生成论文摘要