Human Motion Prediction With Graph Neural Networks
semanticscholar(2021)
摘要
An articulated human body consists of coupled multiple links whose motions affect each other. The effects generated on body parts by the muscles propagate through the body and influence other parts depending on the kinematic and dynamic relations. In this work, we propose to use graph neural networks, propagation networks [1] in particular, to investigate the problem of modelling full-body motion. Given the real movement of one or multiple parts of the body, our work aims to predict the movement of the rest of the body exploiting the underlying graph structure that encodes the task. The body parts and the relations between them are encoded as the nodes of a graph and edges between these nodes. How the nodes are related to each other is learned, and how the effects of multiple nodes on each node should be accumulated is computed in graph structure. A publicly available whole body motion data set is used to train our network. Preliminary results showed that the system could predict motion of body parts given ground truth motion of a subset of hand, foot and head. Previously, [3] and [4] developed deep recurrent neural networks to model human motion, and learned time-dependent short-term representations of human body. [5] and [6] developed vision dependent models which are not time-dependant and they used the model to synthesize new trajectories. Our propagation network model is not time-dependent, the predictions are made through time by chaining predictions back to back. We further explicitly represent each link and let the system learn to propagate the effects of chain of links in each single step.
更多查看译文
AI 理解论文
溯源树
样例
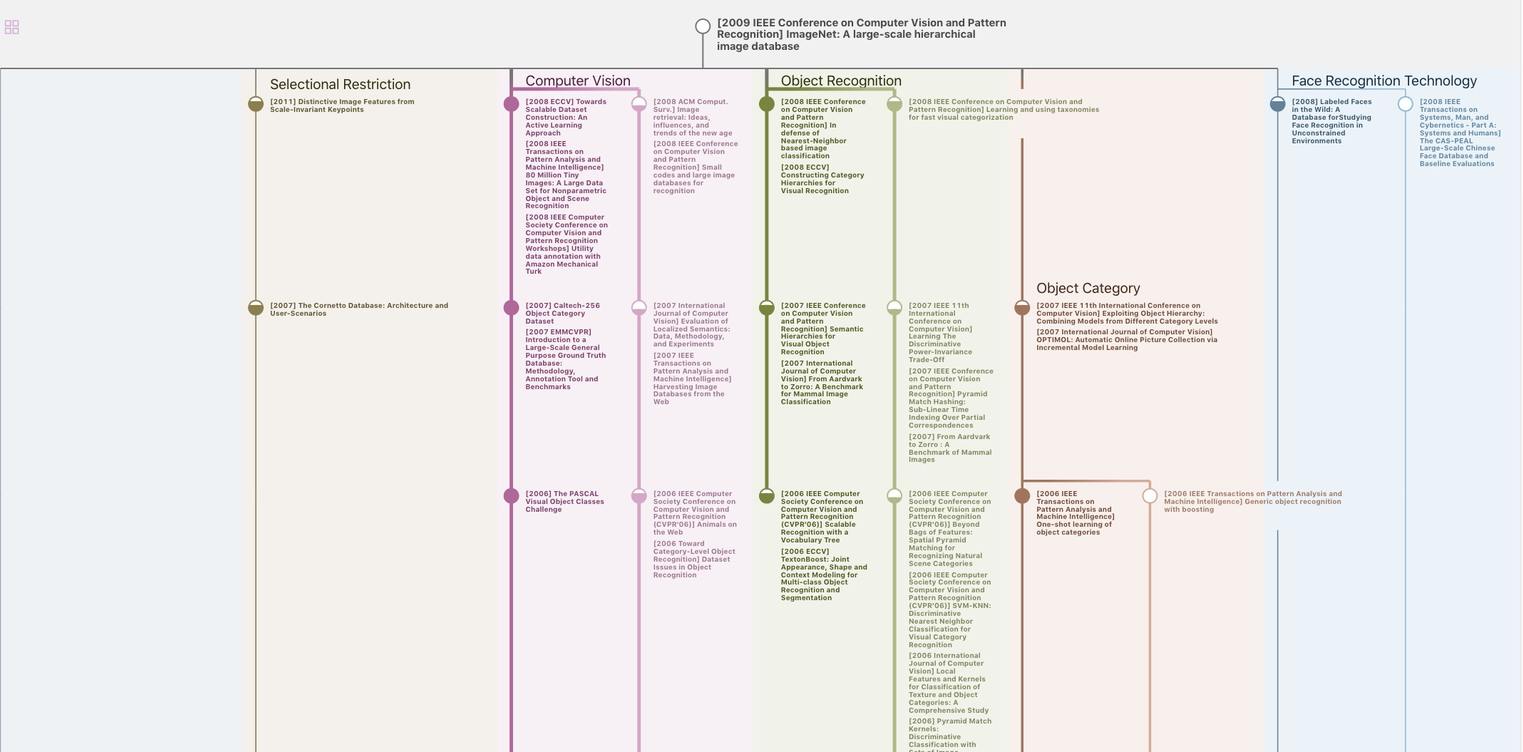
生成溯源树,研究论文发展脉络
Chat Paper
正在生成论文摘要