Sparse to Dense Dynamic 3D Facial Expression Generation
HAL (Le Centre pour la Communication Scientifique Directe)(2022)
摘要
In this paper, we propose a solution to the task of generating dynamic 3D facial expressions from a neutral 3D face and an expression label. This involves solving two sub-problems: (i) modeling the temporal dynamics of expressions, and (ii) deforming the neutral mesh to obtain the expressive counterpart. We represent the temporal evolution of expressions using the motion of a sparse set of 3D landmarks that we learn to generate by training a manifold-valued GAN (Motion3DGAN). To better encode the expression-induced deformation and disentangle it from the identity information, the generated motion is represented as per-frame displacement from a neutral configuration. To generate the expressive meshes, we train a Sparse2Dense mesh Decoder (S2D-Dec) that maps the landmark displacements to a dense, per-vertex displacement. This allows us to learn how the motion of a sparse set of landmarks influences the deformation of the overall face surface, independently from the identity. Experimental results on the CoMA and D3DFACS datasets show that our solution brings significant improvements with respect to previous solutions in terms of both dynamic expression generation and mesh reconstruction, while retaining good generalization to unseen data. Code and models are available at https://github.com/CRISTAL-3DSAM/Sparse2Dense.
更多查看译文
关键词
Face and gestures,3D from multi-view and sensors,Image and video synthesis and generation
AI 理解论文
溯源树
样例
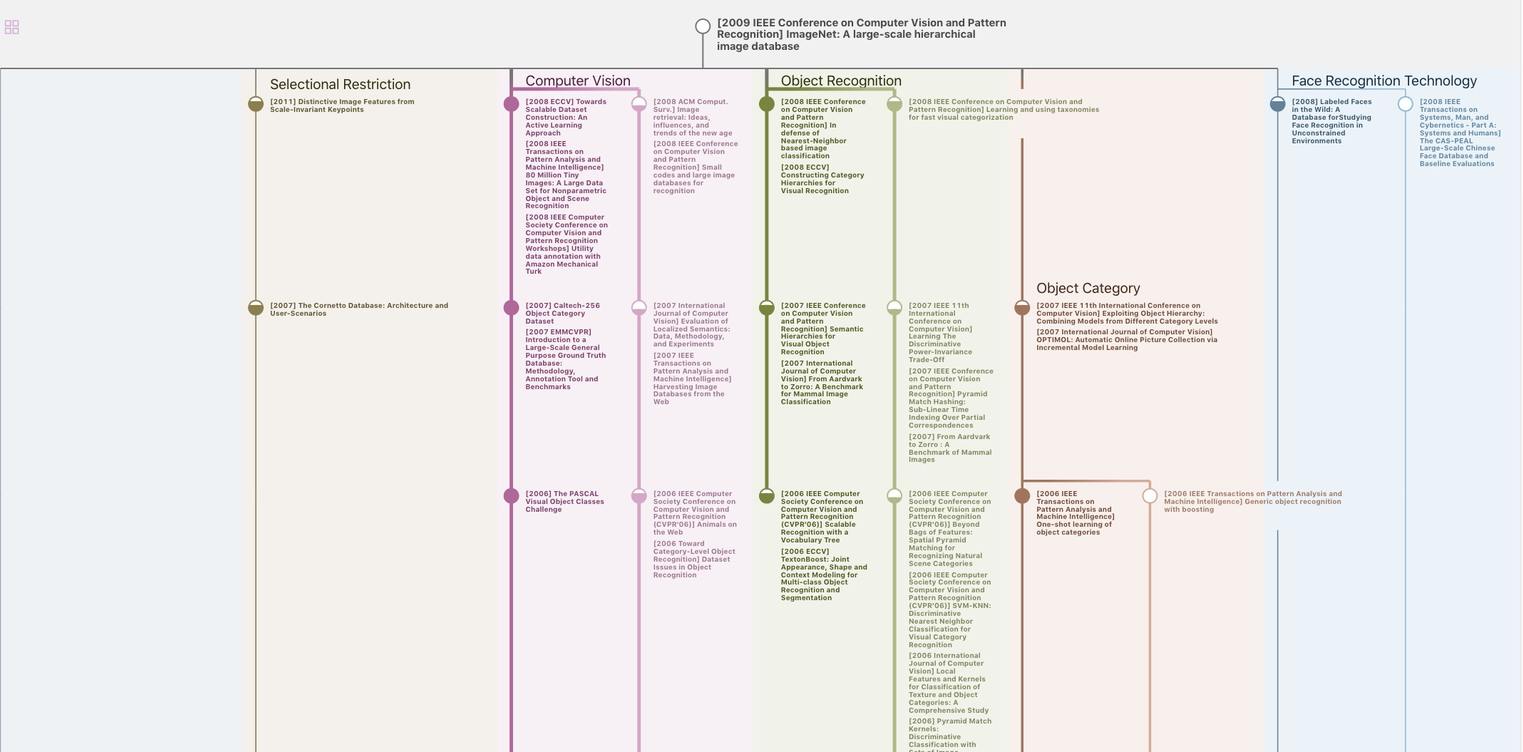
生成溯源树,研究论文发展脉络
Chat Paper
正在生成论文摘要