Performance of deep learning in mapping water quality of Lake Simcoe with long-term Landsat archive
ISPRS Journal of Photogrammetry and Remote Sensing(2022)
摘要
Remote sensing provides full-coverage and dynamic water quality monitoring with high efficiency and low consumption. Deep learning (DL) has been progressively used in water quality retrieval because it efficiently captures the potential relationship between target variables and imagery. In this study, the multimodal deep learning (MDL) models were developed and rigorously validated using atmospherically corrected Landsat remote sensing reflectance data and synchronous water quality measurements for estimating long-term Chlorophyll-a (Chl-a), total phosphorus (TP), and total nitrogen (TN) in Lake Simcoe, Canada. Since TP and TN are non-optically active, their retrievals were based on the fact that they are closely related to the optically active constituents (OACs) such as Chl-a. We trained the MDL models with one in-situ measured data set (for Chl-a, N = 315, for TP and TN, N = 303), validated the models with two independent data sets (N = 147), and compared the model performances with several DL, machine learning, and empirical algorithms. The results indicated that the MDL models adequately estimated Chl-a (mean absolute error (MAE) = 32.57%, Bias = 10.61%), TP (MAE = 42.58%, Bias = −2.82%), and TN (MAE = 35.05%, Bias = 13.66%), and outperformed several other candidate algorithms, namely the progressively decreasing deep neural network (DNN), a DNN with trainable parameters similar to MDL but without splitting input features, the eXtreme Gradient Boosting, the support vector regression, the NASA Ocean Color two-band and three-band ratio algorithms, and another empirical algorithm of Landsat data in clear lakes. Using the MDL models, we reconstructed the historical spatiotemporal patterns of Chl-a, TP, and TN in Lake Simcoe since 1984, and investigated the effects of two water quality improvement programs. In addition, the physical mechanism and interpretability of the MDL models were explored by quantifying the contribution of each feature to the model outputs. The framework proposed in this study provides a practical method for long-term Chl-a, TP, and TN estimation at the regional scale.
更多查看译文
关键词
Deep learning,Remote sensing,Water quality,Chlorophyll-a,Total phosphorous,Total nitrogen
AI 理解论文
溯源树
样例
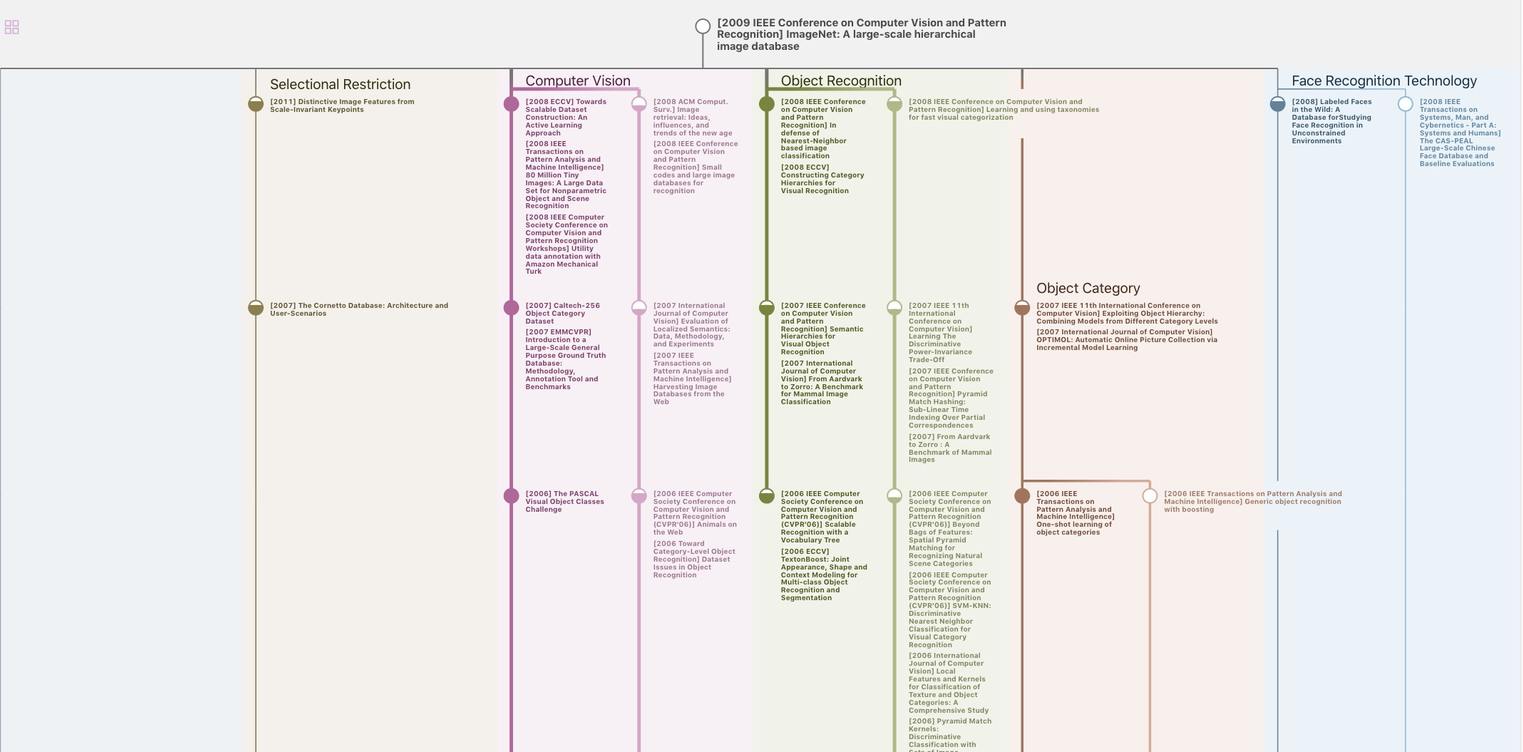
生成溯源树,研究论文发展脉络
Chat Paper
正在生成论文摘要