Congestion-aware motion planning game with Markov decision process dynamics
ArXiv(2022)
摘要
To model a competitive multi-agent motion planning scenario for heterogeneous players with distinct dynamics and objectives, we propose a novel atomic congestion game framework with Markov decision process (MDP) dynamics. We assume that all players share the state-action space but have unique costs and transition dynamics. Each player’s cost is a function of the joint state-action density, and thus is implicitly coupled to the opponents’ policies. With proper cost design, the resulting Nash equilibrium avoids congesting the state-action space and each player optimally achieves its individual objective with respect to the presence of its opponents. For a class of player cost functions, we demonstrate equivalence between players’ Q-value functions and the KKT point of a potential minimization problem, and derive sufficient conditions for the existence of a unique Nash equilibrium. Finally, we outline a learning algorithm for finding the Nash equilibrium that extends single-agent MDP/reinforcement learning algorithms and has linear complexity in the number of players. Throughout the paper, we provide examples for multi-agent motion planning, accumulating in a multi-robot warehouse demonstration, in which robots autonomously retrieve and deliver packages while avoiding collisions.
更多查看译文
AI 理解论文
溯源树
样例
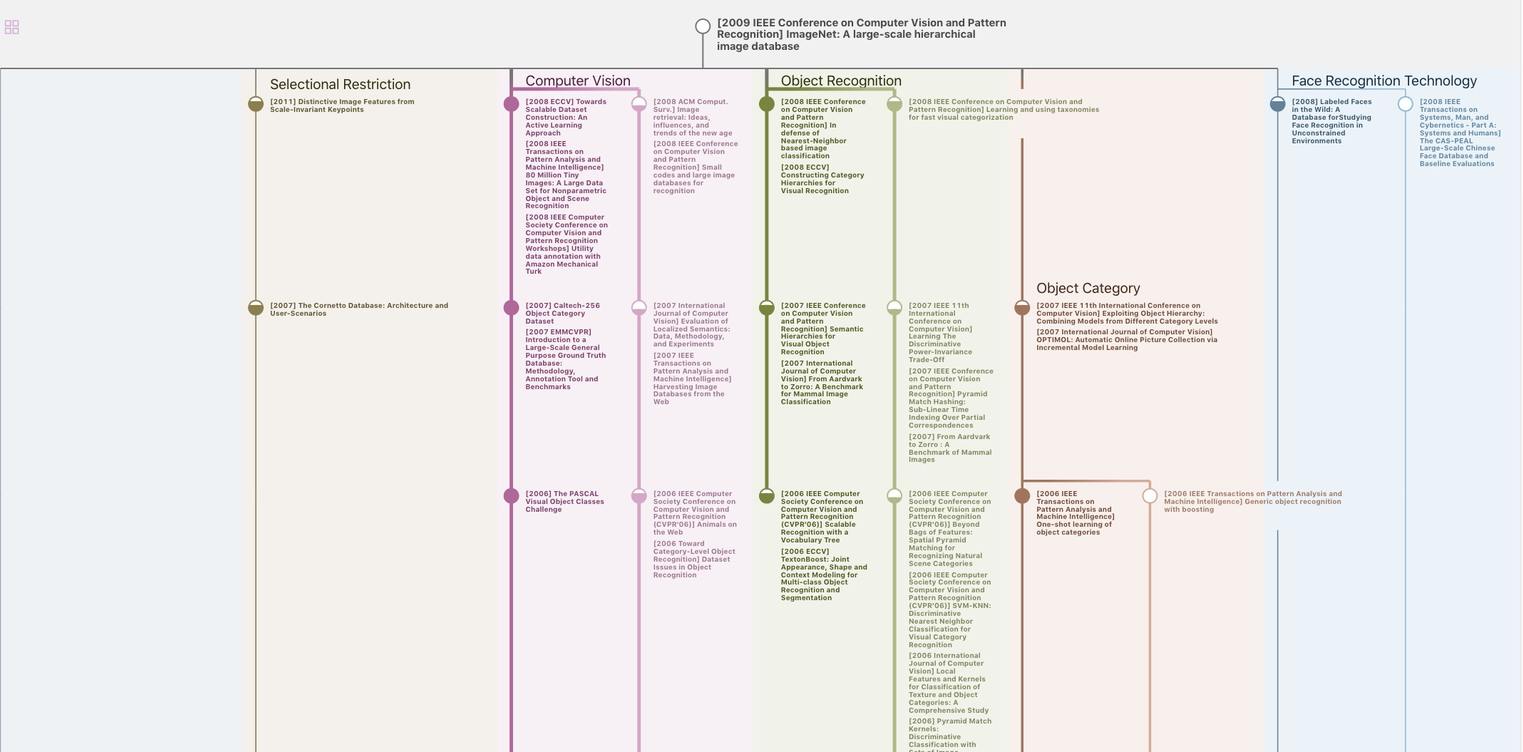
生成溯源树,研究论文发展脉络
Chat Paper
正在生成论文摘要