Measurement of Novelty Difficulty in Monopoly
semanticscholar(2022)
摘要
Novelties in learning algorithms are inherently different than anomalies and outliers. Novelty characterization and detection need to focus and differentiate between these terms. In recent times, DARPA Science of Artificial Intelligence and Learning for Open-world Novelty (SAIL-ON) project has shown advancement in novelty frameworks that discuss novelty characterization, detection and response for learning algorithms in perceptual and action domains. In this work, we implement one of the Novelty Measurement framework in a multi-agent multi-goal based agent domain. First, we breakdown the framework with the goal of implementation and testing for the Monopoly domain. Secondly, we propose a graph representation of the monopoly environment to be used as an effective representation of the agents mental model. Finally, we extend the learning framework of a reinforcement learning based agent to augment the state representation with the graph mental model. Experiments show the empirical calculation of the novelty difficulty according to the framework.
更多查看译文
AI 理解论文
溯源树
样例
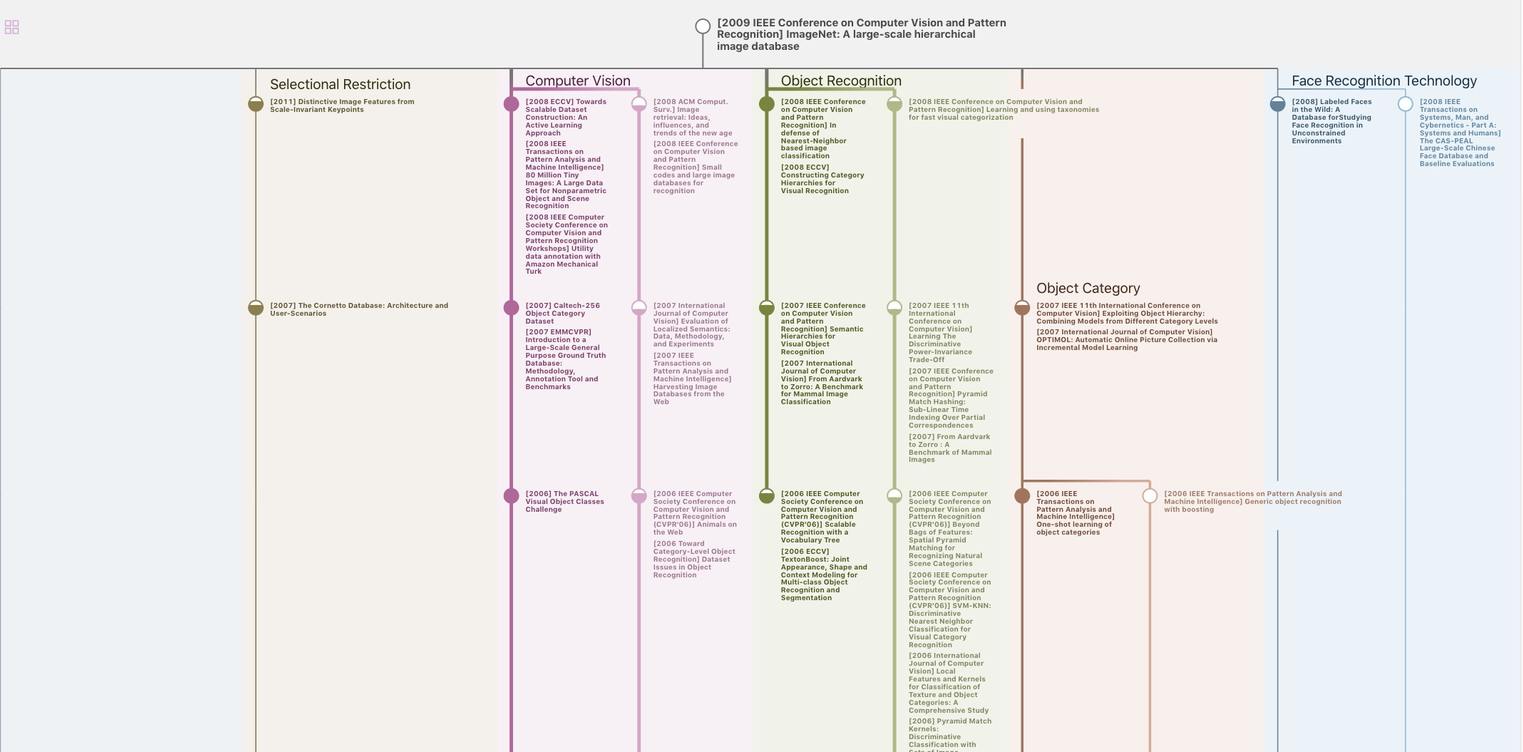
生成溯源树,研究论文发展脉络
Chat Paper
正在生成论文摘要