Predicting pedestrian crash occurrence and injury severity in Texas using tree-based machine learning models
TRANSPORTATION PLANNING AND TECHNOLOGY(2023)
摘要
This study investigates the frequency and injury severity of pedestrian crashes across Texas using tree-based machine learning models. Ten years of police records are used along with roadway inventory and other sources to map 78,000 + pedestrian crashes over 700,000 road segments. Methods like random forests (RF), gradient boosting (LightGBM and XGBoost), and Bayesian additive regression trees (XBART) are applied and compared. The crash frequency models indicate that highway design variables have significant impacts on crash frequencies. Severity models show how higher speed limits significantly increase the likelihood of pedestrian fatalities and severe injuries, and how intoxication (of drivers or pedestrians) lead to more severe injuries. The 4 specifications perform similarly in predicting crash counts, with LightGBM having much faster computing times. Across the crash-severity models, XBART achieved greater precision values but with significantly higher computating times.
更多查看译文
关键词
Pedestrian safety,crash counts,injury severity,machine learning,random forest,gradient boosting
AI 理解论文
溯源树
样例
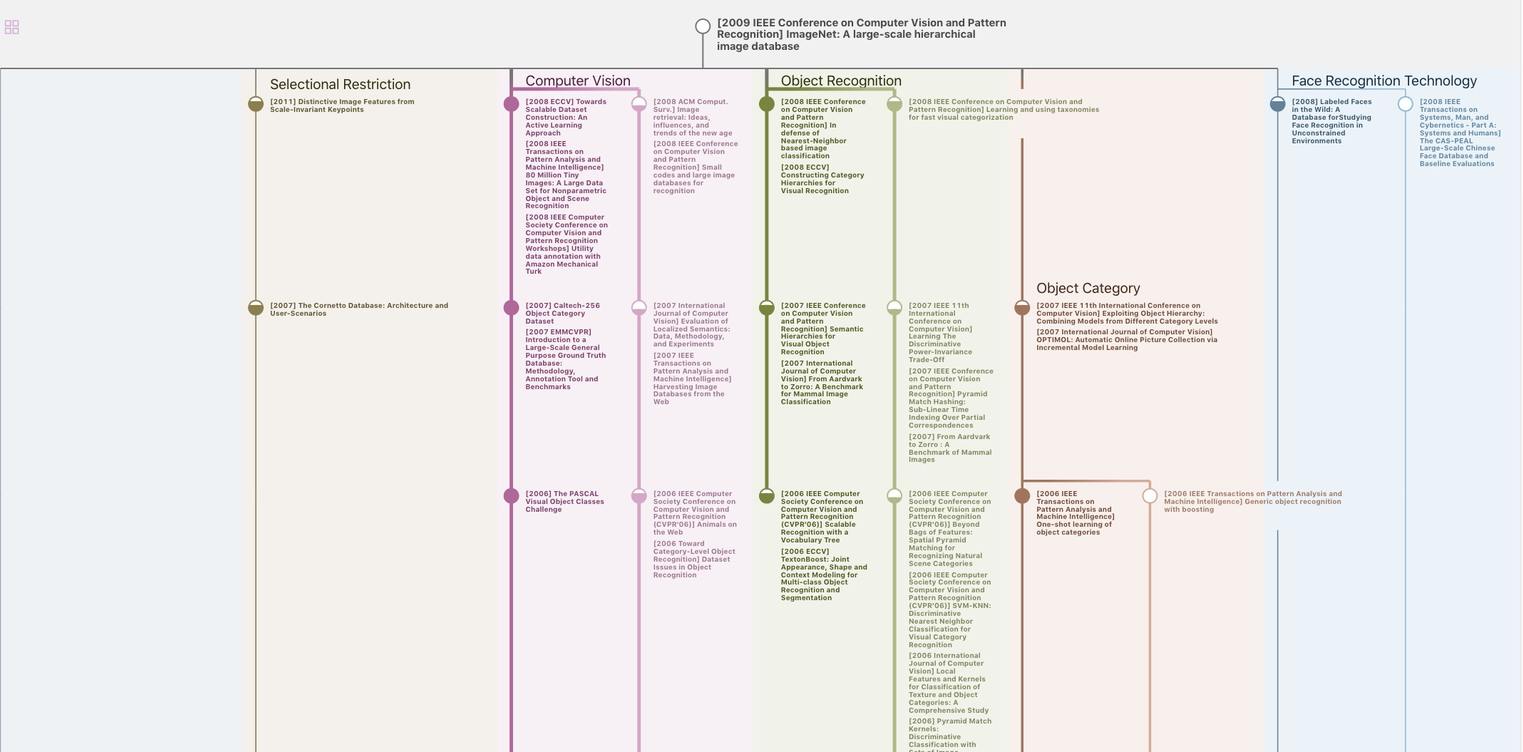
生成溯源树,研究论文发展脉络
Chat Paper
正在生成论文摘要