Efficient pneumatic actuation modeling using hybrid physics-based and data-driven framework
Cell Reports Physical Science(2022)
摘要
Soft robotics, characterized by structural compliance, offers advantages when handling fragile objects and interacting with humans. Modeling the mechanical behavior of soft robots, however, is often overlooked in previous studies due to the difficulty of capturing their nonlinear deformation. In this work, we present a highly efficient predictor for pneumatic actuation utilizing a combination of data-driven surrogate modeling and physics-based optimization. This hybrid methodology explicitly captures the nonlinear mechanics of pneumatic components, which greatly accelerates the searching of static equilibrium, and thus help elucidate how the overall grasping system will interact with the environment. We have additively manufactured and tested a robotic device on various objects. Results show that our model can predict pneumatic actuation very closely matching to that of the experiments with orders of magnitude faster computational speed than traditional numerical simulations. Moreover, the proposed framework can be extended to model various soft robotic devices with nonlinear actuation components.
更多查看译文
关键词
pneumatic actuator,soft robotics,finite element method,neural network,machine learning
AI 理解论文
溯源树
样例
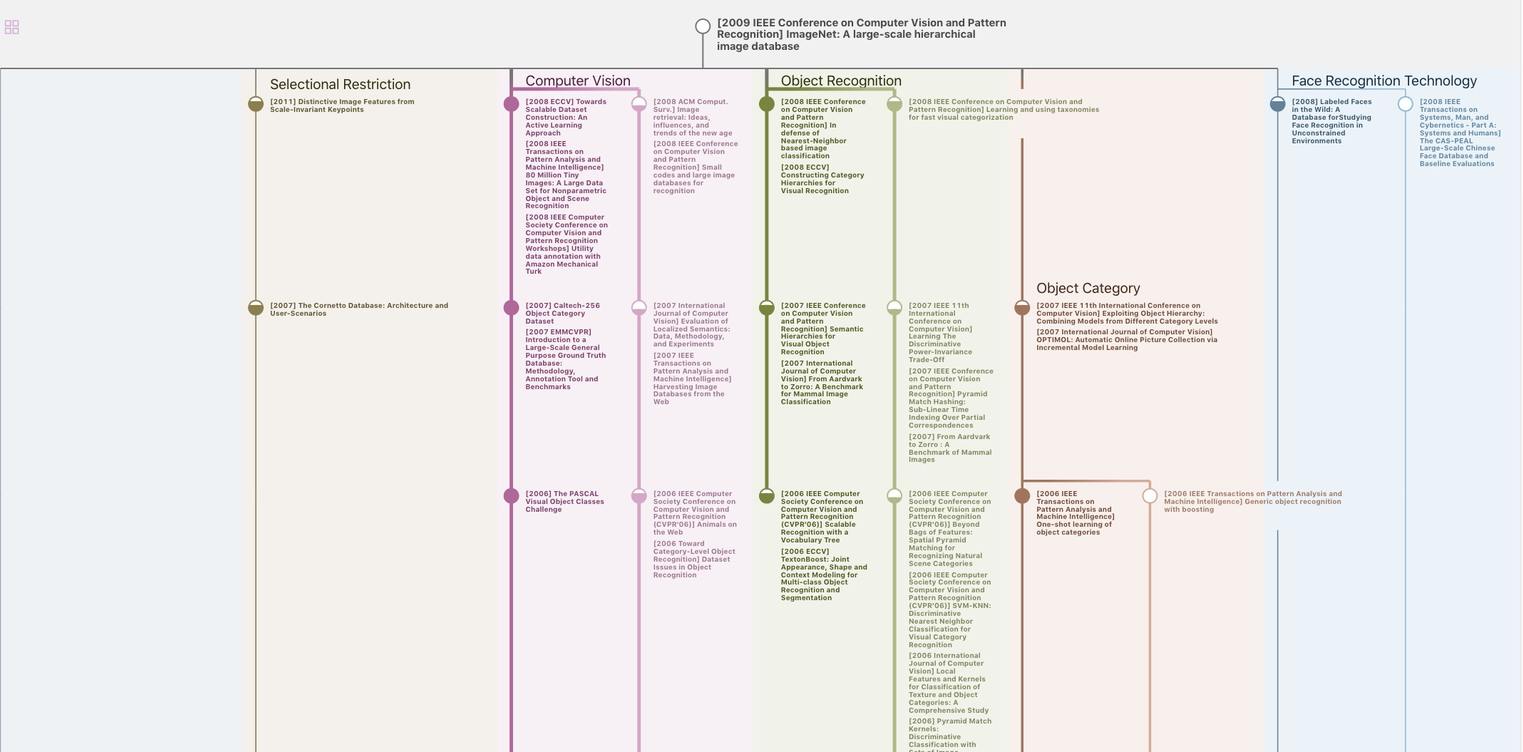
生成溯源树,研究论文发展脉络
Chat Paper
正在生成论文摘要