From Predictions to Decisions: The Importance of Joint Predictive Distributions
arXiv (Cornell University)(2021)
摘要
A fundamental challenge for any intelligent system is prediction: given some inputs, can you predict corresponding outcomes? Most work on supervised learning has focused on producing accurate marginal predictions for each input. However, we show that for a broad class of decision problems, accurate joint predictions are required to deliver good performance. In particular, we establish several results pertaining to combinatorial decision problems, sequential predictions, and multi-armed bandits to elucidate the essential role of joint predictive distributions. Our treatment of multi-armed bandits introduces an approximate Thompson sampling algorithm and analytic techniques that lead to a new kind of regret bound.
更多查看译文
关键词
joint predictive,predictions,decisions,importance
AI 理解论文
溯源树
样例
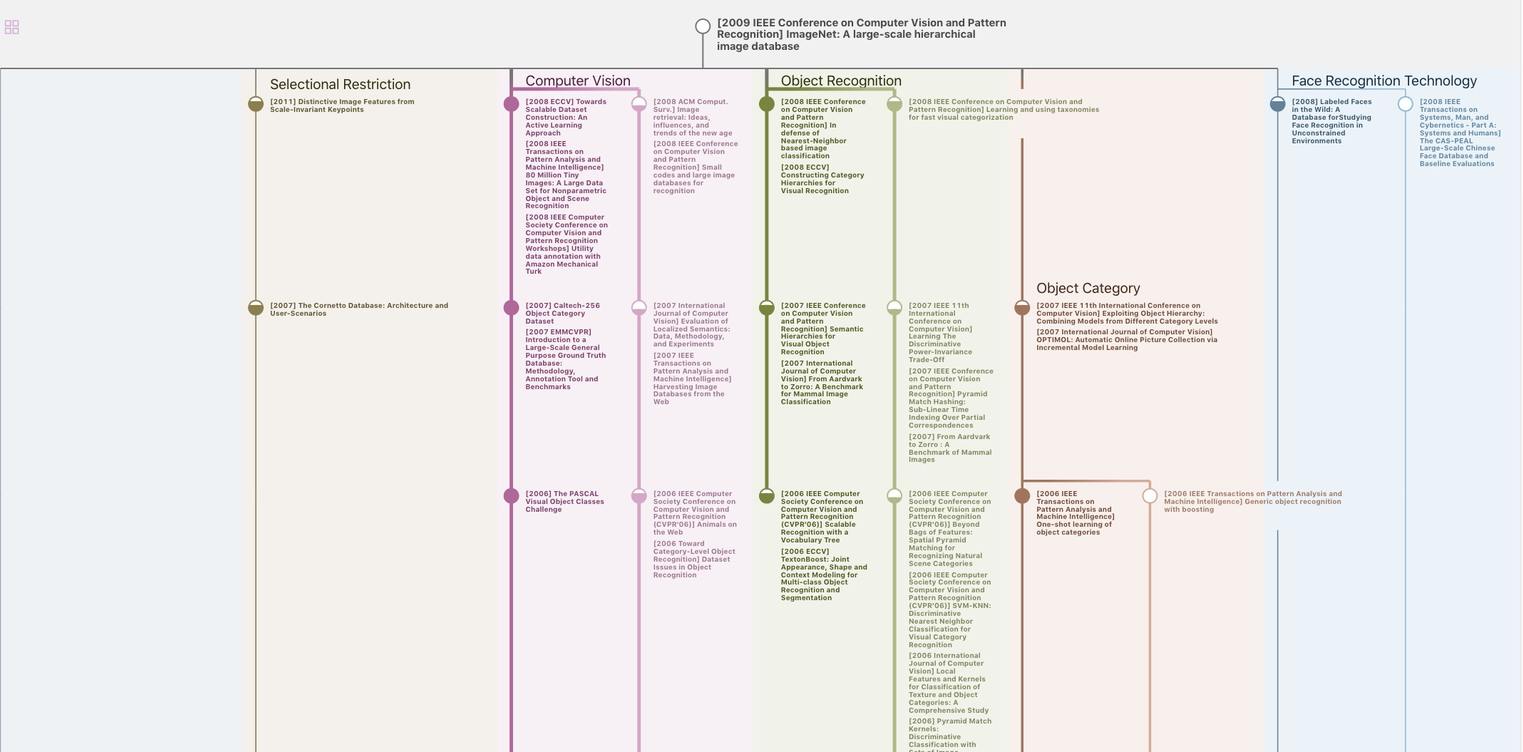
生成溯源树,研究论文发展脉络
Chat Paper
正在生成论文摘要