Communication-Efficient Proactive MPC for Dynamic Groups with Dishonest Majorities
APPLIED CRYPTOGRAPHY AND NETWORK SECURITY, ACNS 2022(2022)
摘要
Secure multiparty computation (MPC) has recently been increasingly adopted to secure cryptographic keys in enterprises, cloud infrastructure, and cryptocurrency and blockchain-related settings such as wallets and exchanges. Using MPC in blockchains and other distributed systems highlights the need to consider dynamic settings. In such dynamic settings, parties, and potentially even parameters of underlying secret sharing and corruption tolerance thresholds of sub-protocols, may change over the lifetime of the protocol. In particular, stronger threat models - in which mobile adversaries control a changing set of parties (up to t out of n involved parties at any instant), and may eventually corrupt all n parties over the course of a protocol's execution - are becoming increasingly important for such real world deployments; secure protocols designed for such models are known as Proactive MPC (PMPC). In this work, we construct the first efficient PMPC protocol for dynamic groups (where the set of parties changes over time) secure against a dishonest majority of parties. Our PMPC protocol only requires O(n(2)) (amortized) communication per secret, compared to existing PMPC protocols that require O(n(4)) and only consider static groups with dishonest majorities. At the core of our PMPC protocol is a new efficient technique to perform multiplication of secret shared data (shared using a bivariate scheme) with O(n root n) communication with security against a dishonest majority without requiring pre-computation. We also develop a new efficient bivariate batched proactive secret sharing (PSS) protocol for dishonest majorities, which may be of independent interest. This protocol enables multiple dealers to contribute different secrets that are efficiently shared together in one batch; previous batched PSS schemes required all secrets to come from a single dealer.
更多查看译文
AI 理解论文
溯源树
样例
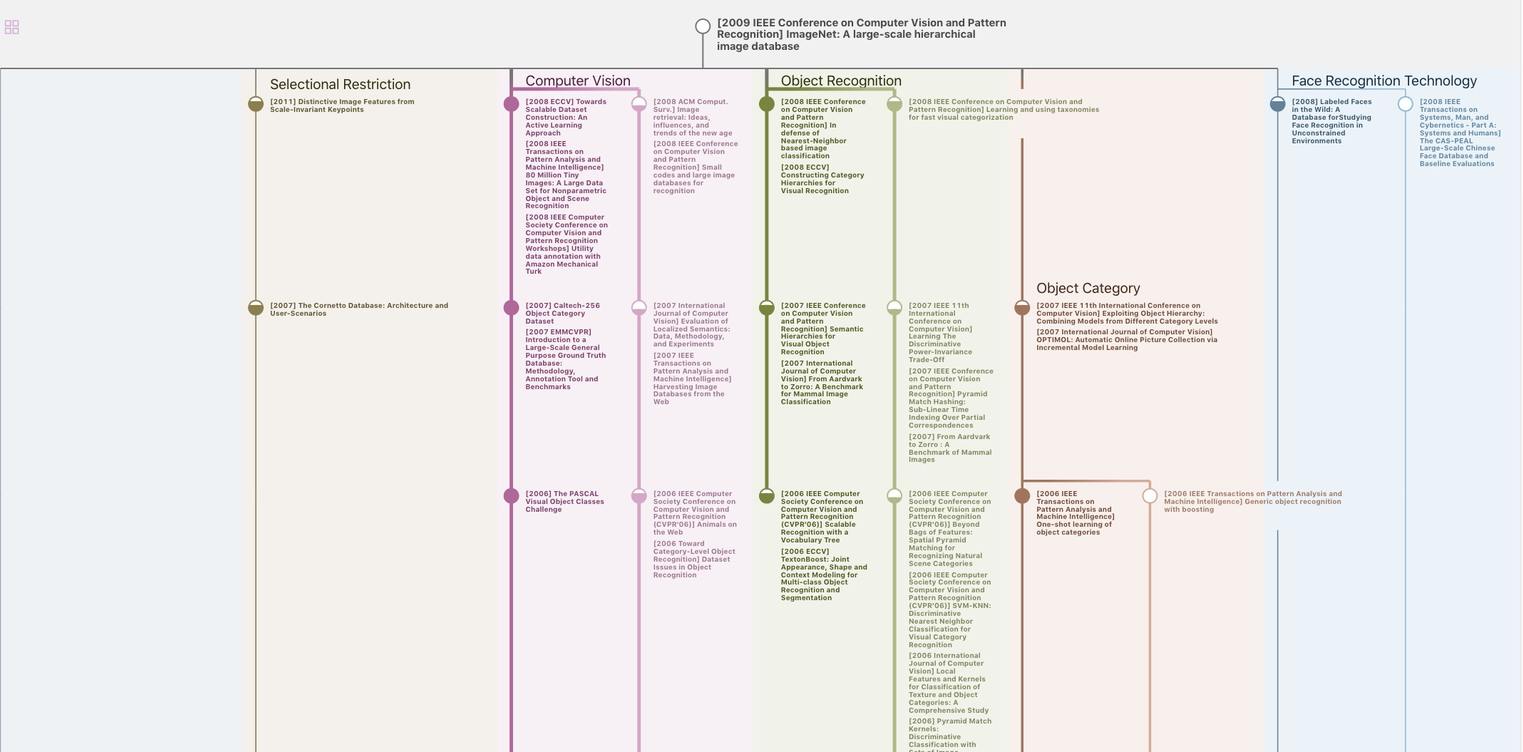
生成溯源树,研究论文发展脉络
Chat Paper
正在生成论文摘要