Maximum A Posteriori Inference of Random Dot Product Graphs Via Conic Programming
SIAM journal on optimization(2022)
摘要
We present a convex cone program to infer the latent probability matrix of a random dot product graph (RDPG). The optimization problem maximizes the Bernoulli maximum likelihood function with an added nuclear norm regularization term. The dual problem has a particularly nice form, related to the well-known semidefinite program relaxation of the maxcut problem. Using the primal-dual optimality conditions, we bound the entries and rank of the primal and dual solutions. Furthermore, we bound the optimal objective value and prove asymptotic consistency of the probability estimates of a slightly modified model under mild technical assumptions. Our experiments on synthetic RDPGs not only recover natural clusters, but also reveal the underlying low-dimensional geometry of the original data. We also demonstrate that the method recovers latent structure in the Karate Club Graph and synthetic U.S. Senate vote graphs and is scalable to graphs with up to a few hundred nodes.
更多查看译文
关键词
 ,random dot product graph,maximum a posteriori,Bayesian inference,regulariza-tion,maximum likelihood estimation,inference,conic programming,convex relaxation,clustering,graph embedding,latent vectors,low rank,consistency
AI 理解论文
溯源树
样例
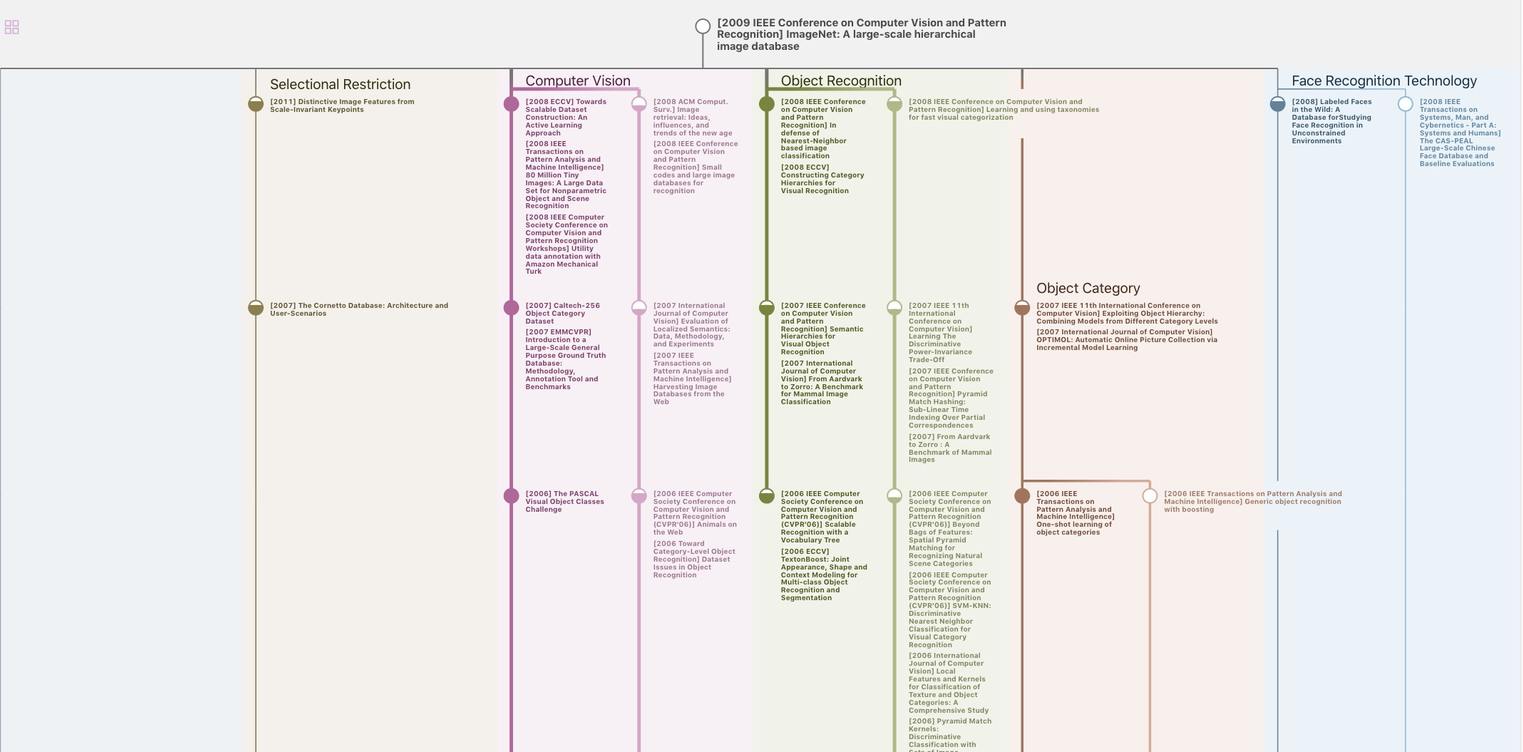
生成溯源树,研究论文发展脉络
Chat Paper
正在生成论文摘要