A UNIFIED ANALYSIS OF DESCENT SEQUENCES IN WEAKLY CONVEX OPTIMIZATION, INCLUDING CONVERGENCE RATES FOR BUNDLE METHODS\ast
SIAM J. Optim.(2023)
摘要
We present a framework for analyzing convergence and local rates of convergence of a class of descent algorithms, assuming the objective function is weakly convex. The framework is general, in the sense that it combines the possibility of explicit iterations (based on the gradient or a subgradient at the current iterate), implicit iterations (using a subgradient at the next iteration, like in the proximal schemes), as well as iterations when the associated subgradient is specially constructed and does not correspond either to the current or the next point (this is the case of descent steps in bundle methods). Under the subdifferential-based error bound on the distance to critical points, linear rates of convergence are established. Our analysis applies, among other techniques, to prox-descent for decomposable functions, the proximal-gradient method for a sum of functions, redistributed bundle methods, and a class of algorithms that can be cast in the feasible descent framework for constrained optimization.
更多查看译文
关键词
weakly convex optimization,descent sequences,convergence rates,unified analysis
AI 理解论文
溯源树
样例
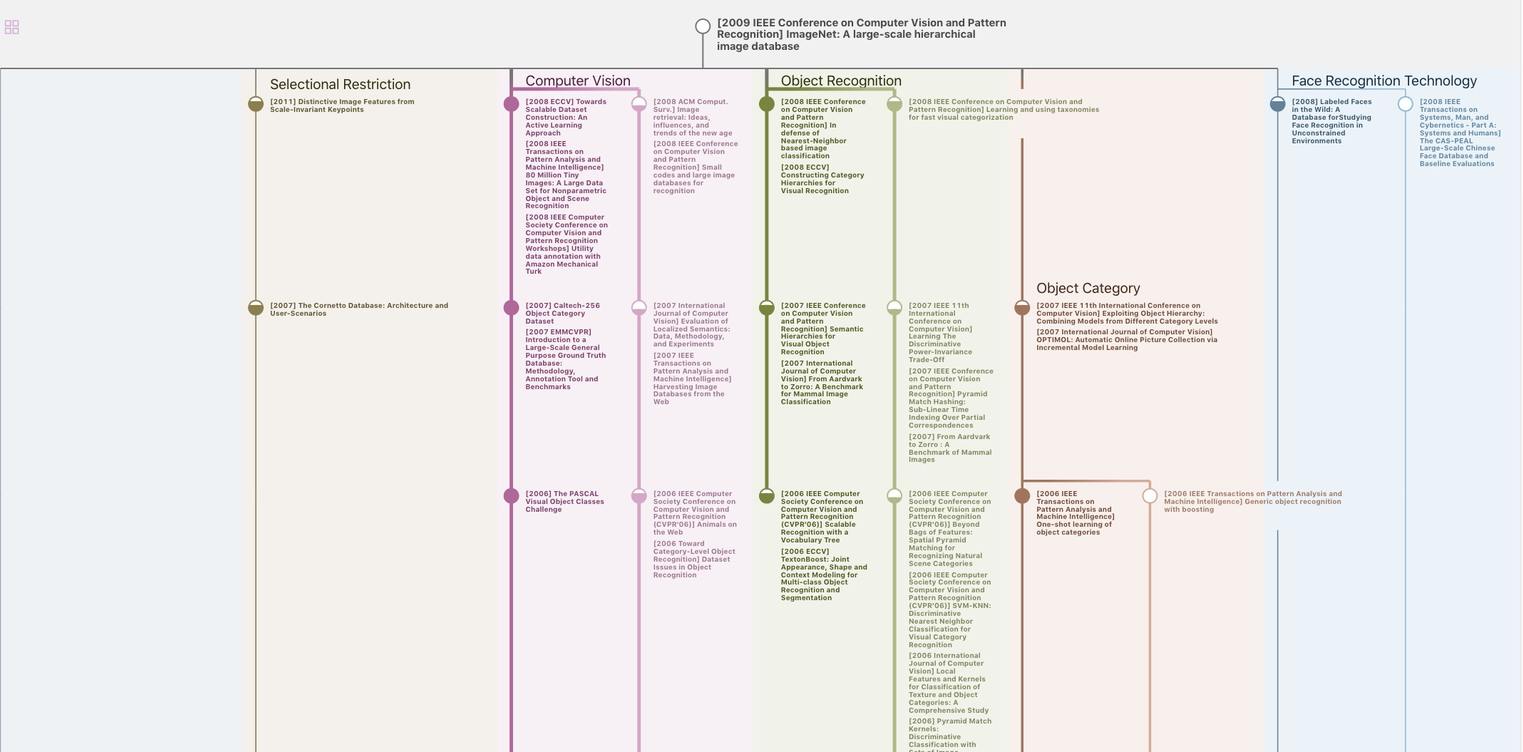
生成溯源树,研究论文发展脉络
Chat Paper
正在生成论文摘要