Multi-Party Verifiable Privacy-Preserving Federated k-Means Clustering in Outsourced Environment
SECURITY AND COMMUNICATION NETWORKS(2021)
摘要
As a commonly used algorithm in data mining, clustering has been widely applied in many fields, such as machine learning, information retrieval, and pattern recognition. In reality, data to be analyzed are often distributed to multiple parties. Moreover, the rapidly increasing data volume puts heavy computing pressure on data owners. Thus, data owners tend to outsource their own data to cloud servers and obtain data analysis results for the federated data. However, the existing privacy-preserving outsourced k-means schemes cannot verify whether participants share consistent data. Considering the scenarios with multiple data owners and sensitive information security in an outsourced environment, we propose a verifiable privacy-preserving federated k-means clustering scheme. In this article, cloud servers and participants perform k-means clustering algorithm over encrypted data without exposing private data and intermediate results in each iteration. In particular, our scheme can verify the shares from participants when updating the cluster centers based on secret sharing, hash function and blockchain, so that our scheme can resist inconsistent share attacks by malicious participants. Finally, the security and experimental analysis are carried out to show that our scheme can protect private data and get high-accuracy clustering results.
更多查看译文
AI 理解论文
溯源树
样例
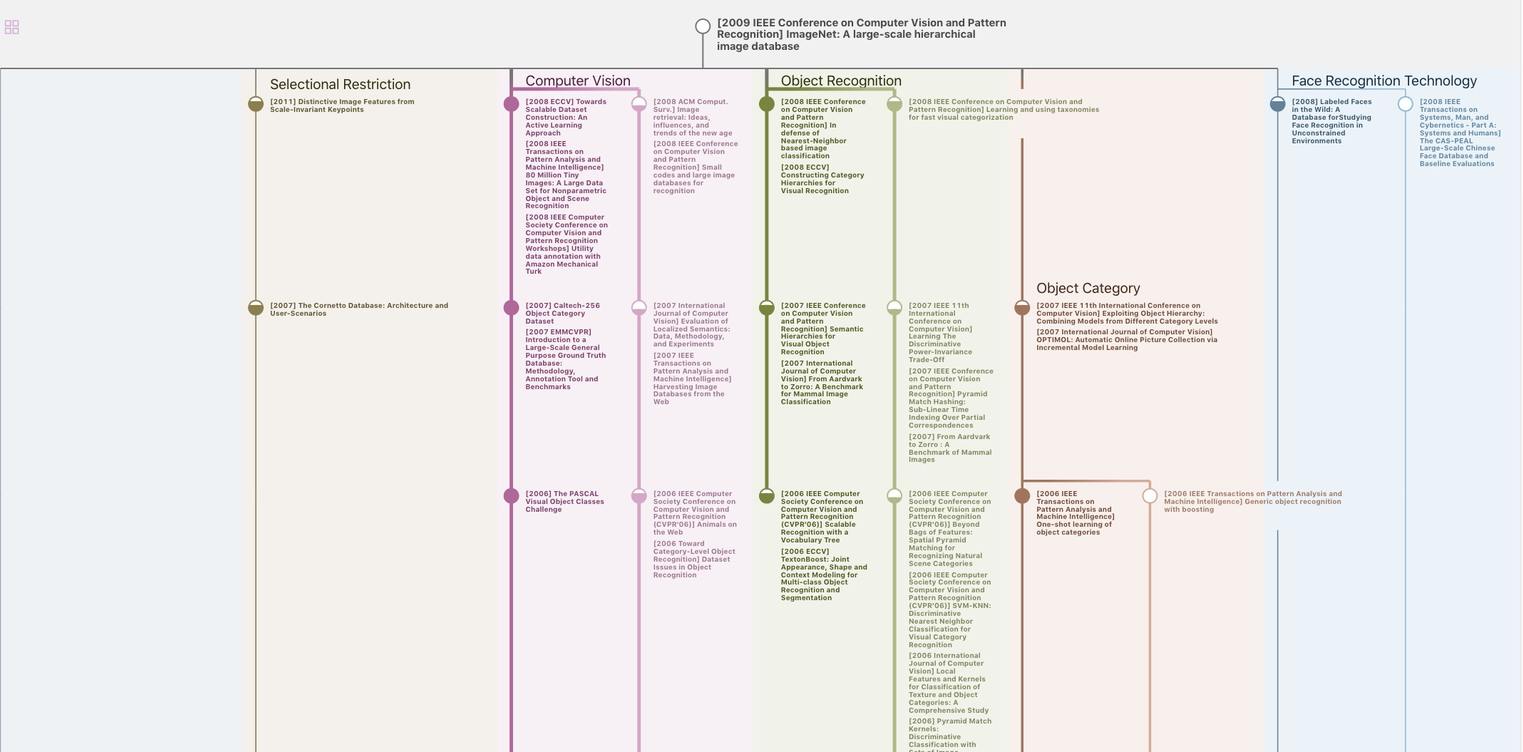
生成溯源树,研究论文发展脉络
Chat Paper
正在生成论文摘要