CENTRAL LIMIT THEOREM AND BOOTSTRAP APPROXIMATION IN HIGH DIMENSIONS: NEAR 1/root n RATES VIA IMPLICIT SMOOTHING
ANNALS OF STATISTICS(2022)
摘要
Nonasymptotic bounds for Gaussian and bootstrap approximation have recently attracted significant interest in high-dimensional statistics. This paper studies Berry-Esseen bounds for such approximations with respect to the multivariate Kolmogorov distance, in the context of a sum of n random vectors that are p-dimensional and i.i.d. Up to now, a growing line of work has established bounds with mild logarithmic dependence on p. However, the problem of developing corresponding bounds with near n(-1/2) dependence on n has remained largely unresolved. Within the setting of random vectors that have sub-Gaussian or subexponential entries, this paper establishes bounds with near n(-1/2) dependence, for both Gaussian and bootstrap approximation. In addition, the proofs are considerably distinct from other recent approaches, and make use of an "implicit smoothing" operation in the Lindeberg interpolation.
更多查看译文
关键词
Central limit theorem, bootstrap, Berry-Esseen theorem, high dimensions
AI 理解论文
溯源树
样例
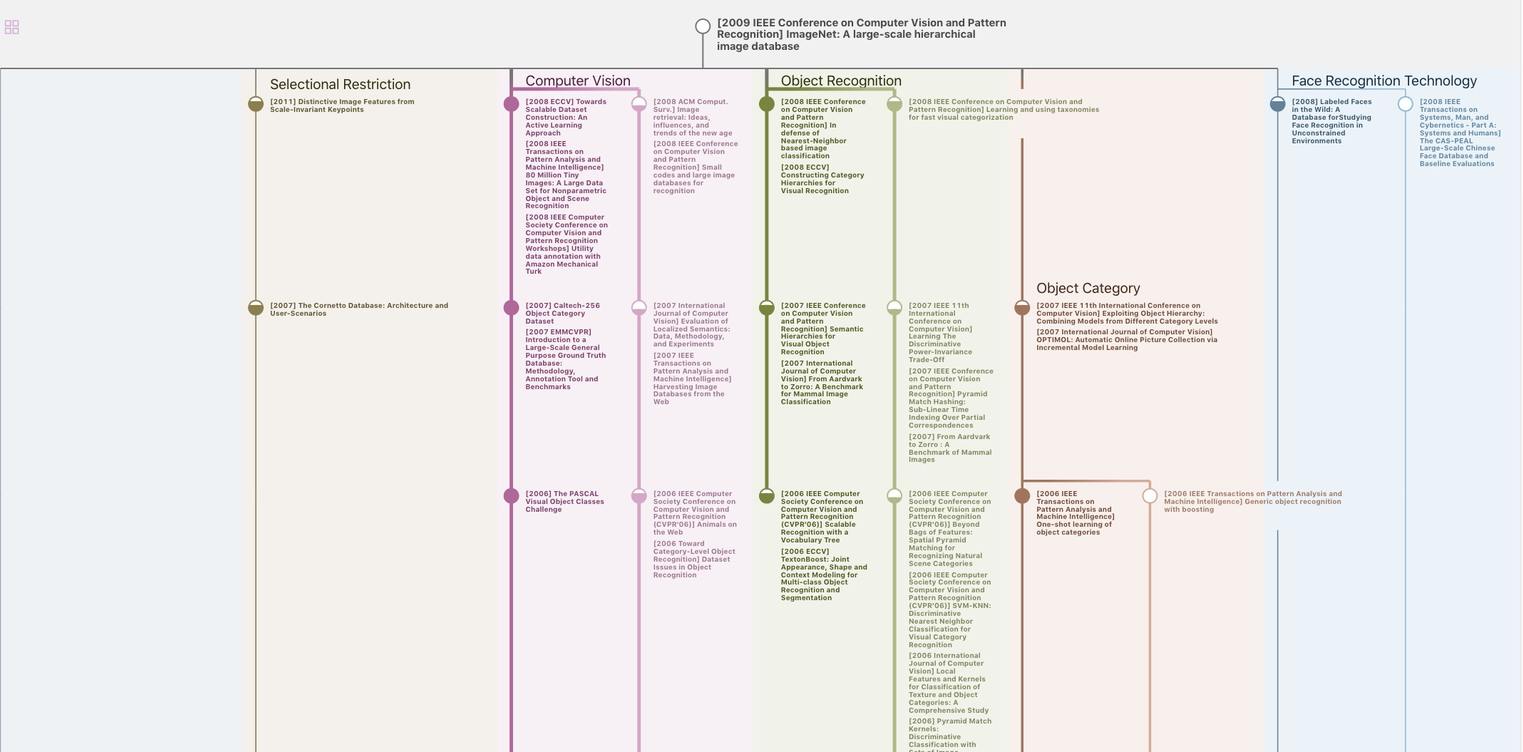
生成溯源树,研究论文发展脉络
Chat Paper
正在生成论文摘要