Multi-Objective Optimisation for Improved Feature Selection.
2021 13th International Conference on Bioinformatics and Biomedical Technology(2021)
摘要
Feature Selection is primordial to modern data science. However, many FS methods exist and choosing an FS method is not straightforward since different FS methods produce different results. Literature abounds with papers on the variability of FS method as well as the many approaches that have been proposed to tackle this variability. In this research, the use of a novel approach based on Multi-Objective Optimization to perform FS was investigated and a solution based thereon was proposed. Different criteria that have been studied previously and have been found to be important but sometimes conflicting as well as other criteria deemed potentially pertinent were studied for optimization. In our experiments feature subsets produced by multiple FS methods on transcriptomics data were merged by using union of pairwise intersections. The stability and performance of these resulting merged subsets for 3 classifiers (Naive Bayes, Bagging and Random Forest) were then calculated and these were the criteria to be optimised. The criteria that were investigated were the Somol, Novovicova, Nogueira, Dice and Lustgarten stability metrics; the average classification accuracy, AUROC and MAE performance metrics across the 3 classifiers and the number of methods used to produce a feature subset. Our results show that the Novovicova stability metric with the 3 average performance metrics and the number of FS methods being considered enabled the user to get a ranking of different feature subsets from which the highest ranking can be selected.
更多查看译文
AI 理解论文
溯源树
样例
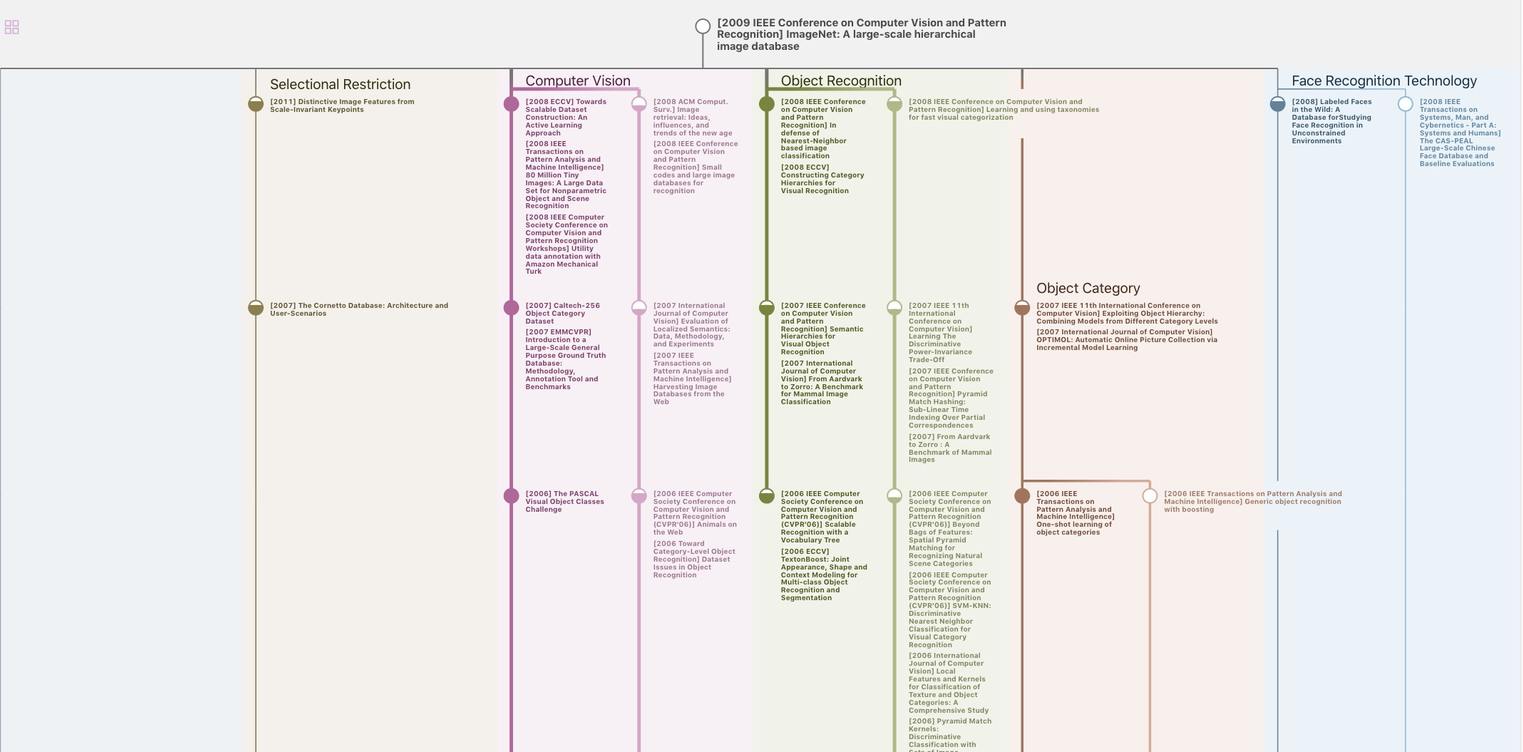
生成溯源树,研究论文发展脉络
Chat Paper
正在生成论文摘要