Multisource Remote Sensing Classification for Coastal Wetland Using Feature Intersecting Learning
IEEE GEOSCIENCE AND REMOTE SENSING LETTERS(2022)
摘要
Accurate remote sensing monitoring of wetland ground objects is of great significance for ecological protection. In this letter, a convolutional neural network based on feature intersecting learning (FIL-CNN) is designed for wetland classification using multisource remote sensing data. The multi-layer shift feature fusion (MSFF) and attention feature selection (AFS) modules are designed to extract the complementary merits. Specifically, the MSFF is applied to each feature extraction unit, and the asymmetric information fusion is achieved through the spatial position shift of grouped features. Thus, the diversified feature representation is achieved. In the prediction stage, the AFS is executed to explore the channel mutually exclusive relationship between multisource features, resulting in emphasizing the meaningful features and eliminating the unnecessary ones. The experimental results prove the effectiveness and generalization of the proposed FIL-CNN on the wetland datasets.
更多查看译文
关键词
Feature extraction,Wetlands,Rivers,Remote sensing,Convolutional neural networks,Support vector machines,Spatial resolution,Asymmetric information fusion,attention feature selection (AFS),convolutional neural network (CNN),multisource wetland classification
AI 理解论文
溯源树
样例
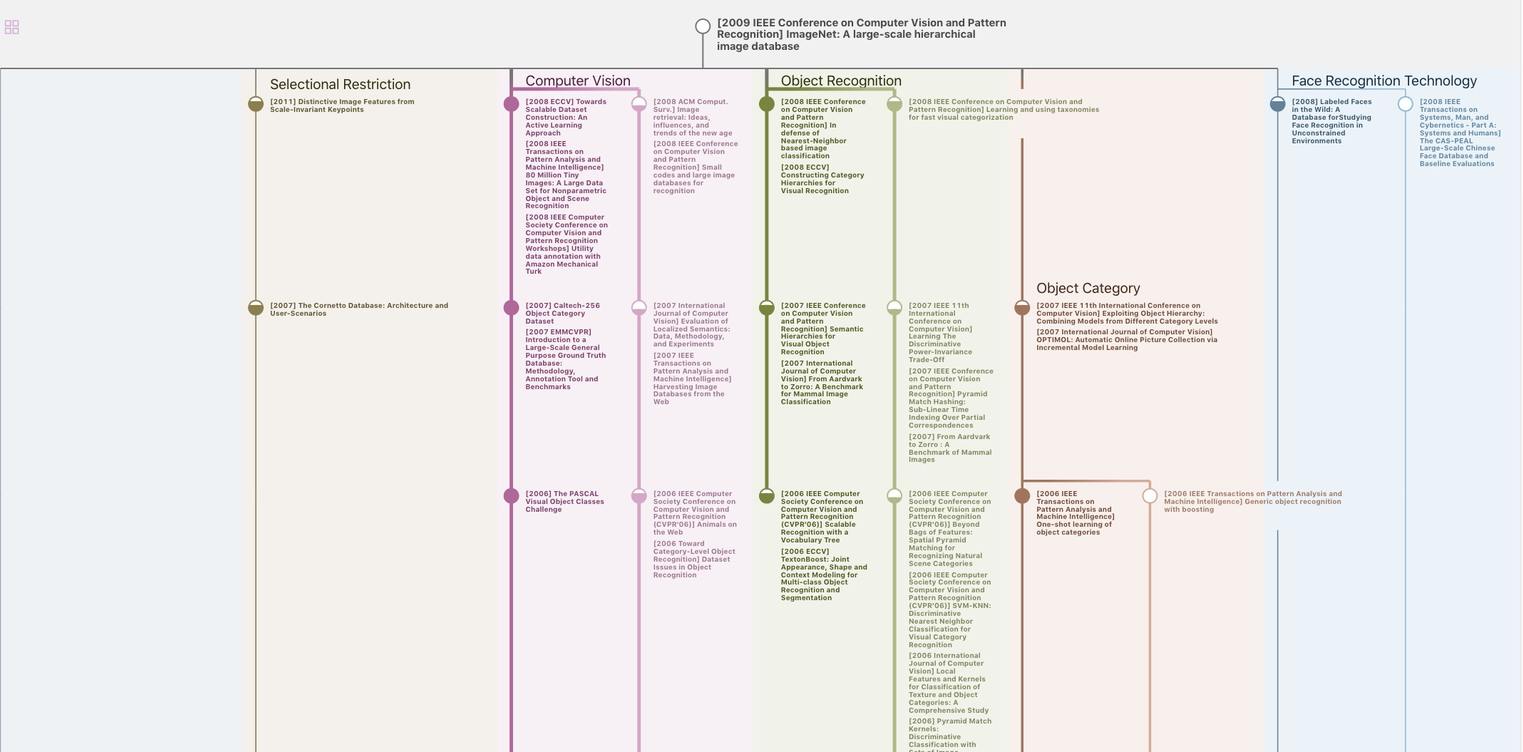
生成溯源树,研究论文发展脉络
Chat Paper
正在生成论文摘要