Fine-Tuning Dependencies with Parameters
International Conference on Extending Database Technology (EDBT)(2022)
摘要
Discovering dependencies from data has been studied extensively, with applications in data mining, data integration and data cleaning. Recent work has proposedmanually parameterized relaxations of traditional dependencies. For example, a Metric Functional Dependency (MFD) with a parameter δ asserts that any pair of tuples that agree on the antecedent attribute values must have similar (not necessarily equal) values of the consequent attributes within a distance of δ . To avoid human burden, we present a framework to automatically fine-tune parameterized dependencies and a proof-of-concept implementation for MFDs. To fine-tune a dependency, we produce a concise pattern tableau, i.e., conditions representing semantically meaningful subsets of the data, along with parameter values that hold within each subset. Our solution is based on a variant of the weighted set cover problem to ensure conciseness, data coverage, and tight parameter values. We demonstrate the efficiency and effectiveness of our approach by analyzing its performance on two real-life datasets.
更多查看译文
AI 理解论文
溯源树
样例
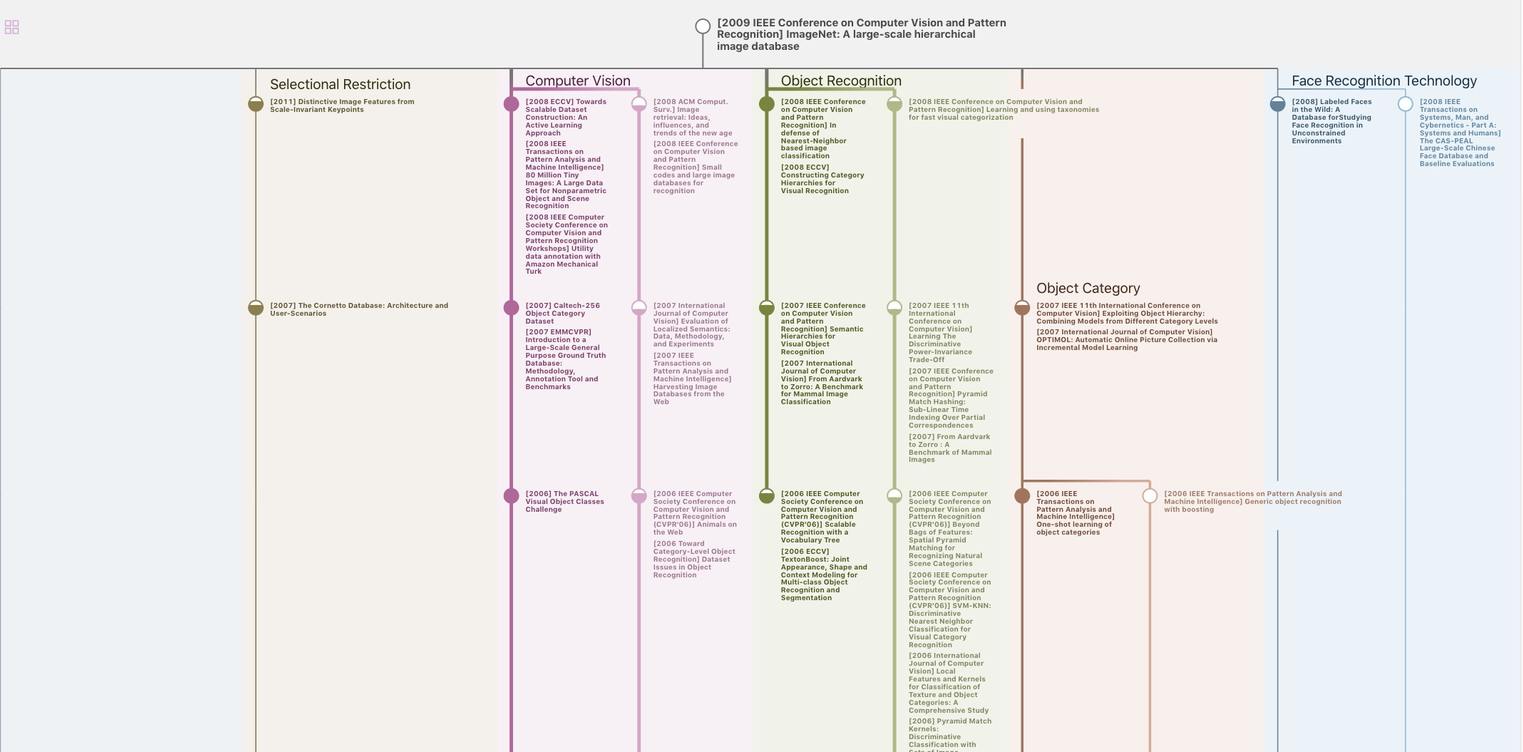
生成溯源树,研究论文发展脉络
Chat Paper
正在生成论文摘要