Adding Interpretability to Neural Knowledge DNA
CYBERNETICS AND SYSTEMS(2022)
摘要
This paper proposes a novel approach that adds the interpretability to Neural Knowledge DNA (NK-DNA) via generating a decision tree. The NK-DNA is a promising knowledge representation approach for acquiring, storing, sharing, and reusing knowledge among machines and computing systems. We introduce the decision tree-based generative method for knowledge extraction and representation to make the NK-DNA more explainable. We examine our approach through an initial case study. The experiment results show that the proposed method can transform the implicit knowledge stored in the NK-DNA into explicitly represented decision trees bringing fair interpretability to neural network-based intelligent systems.
更多查看译文
关键词
Decision trees,deep reinforcement learning,interpretable AI,neural knowledge DNA
AI 理解论文
溯源树
样例
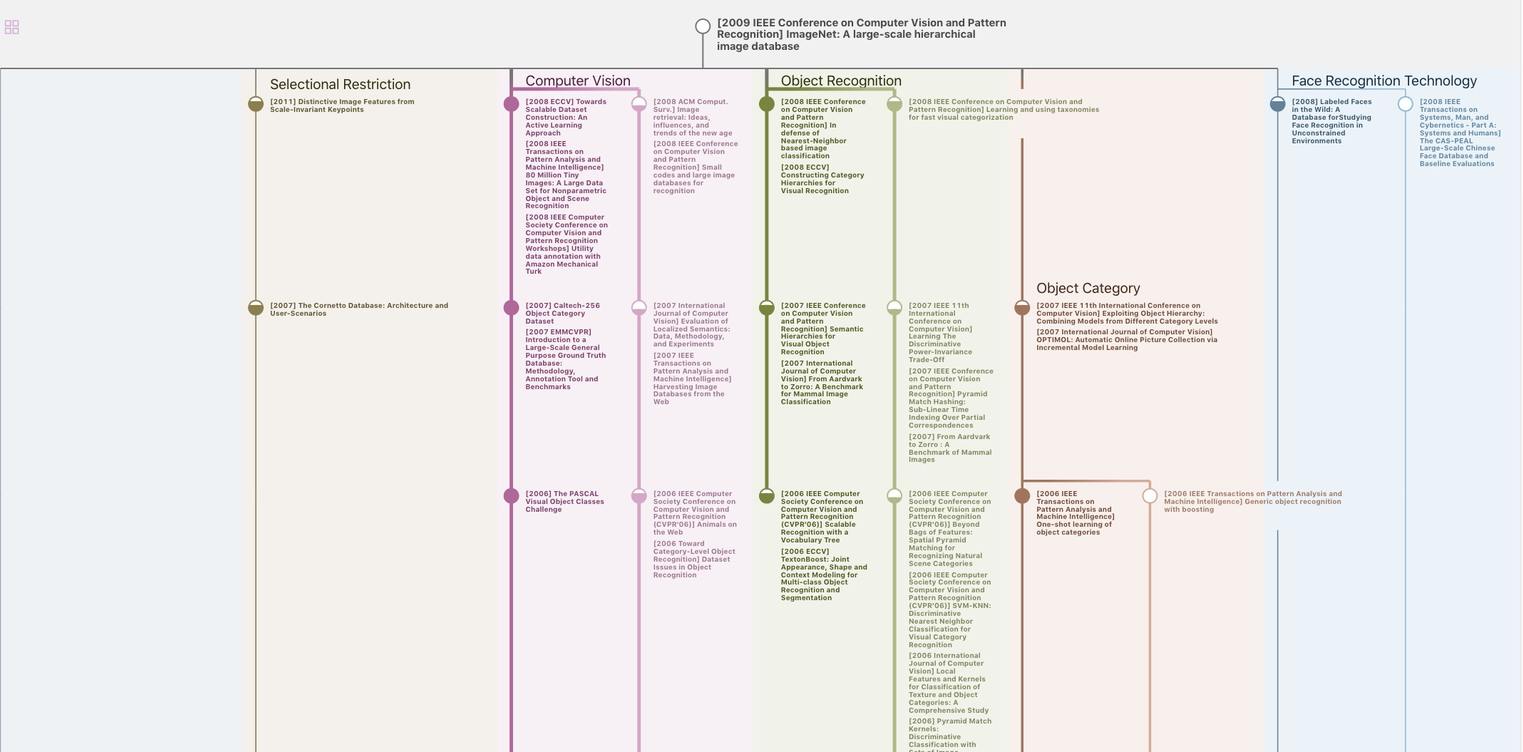
生成溯源树,研究论文发展脉络
Chat Paper
正在生成论文摘要