A Biologically Interpretable Graph Convolutional Network to Link Genetic Risk Pathways and Neuroimaging Phenotypes of Disease
semanticscholar(2021)
摘要
We propose a novel deep neural network for whole-genome imaging-genetics. Our genetics module uses hierarchical graph convolution and pooling operations that mimic the organization of a well-established gene ontology to embed subject-level data into a latent space. The ontology implicitly tracks the convergence of genetic risk across biological pathways, and an attention mechanism automatically identifies the salient edges in our network. We couple the imaging and genetics data using an autoencoder and predictor, which couples the latent embeddings learned for each modality. The predictor uses these embeddings for disease diagnosis, while the decoder regularizes the model. For interpretability, we implement a Bayesian feature selection strategy to extract the discriminative biomarkers of each modality. We evaluate our framework on a population study of schizophrenia that includes two functional MRI (fMRI) paradigms and gene scores derived from Single Nucleotide Polymorphism (SNP) data. Using 10-fold cross-validation, we show that our model achieves better classification performance than the baselines. In an exploratory analysis, we further show that the biomarkers identified by our model are reproducible and closely associated with deficits in schizophrenia.
更多查看译文
AI 理解论文
溯源树
样例
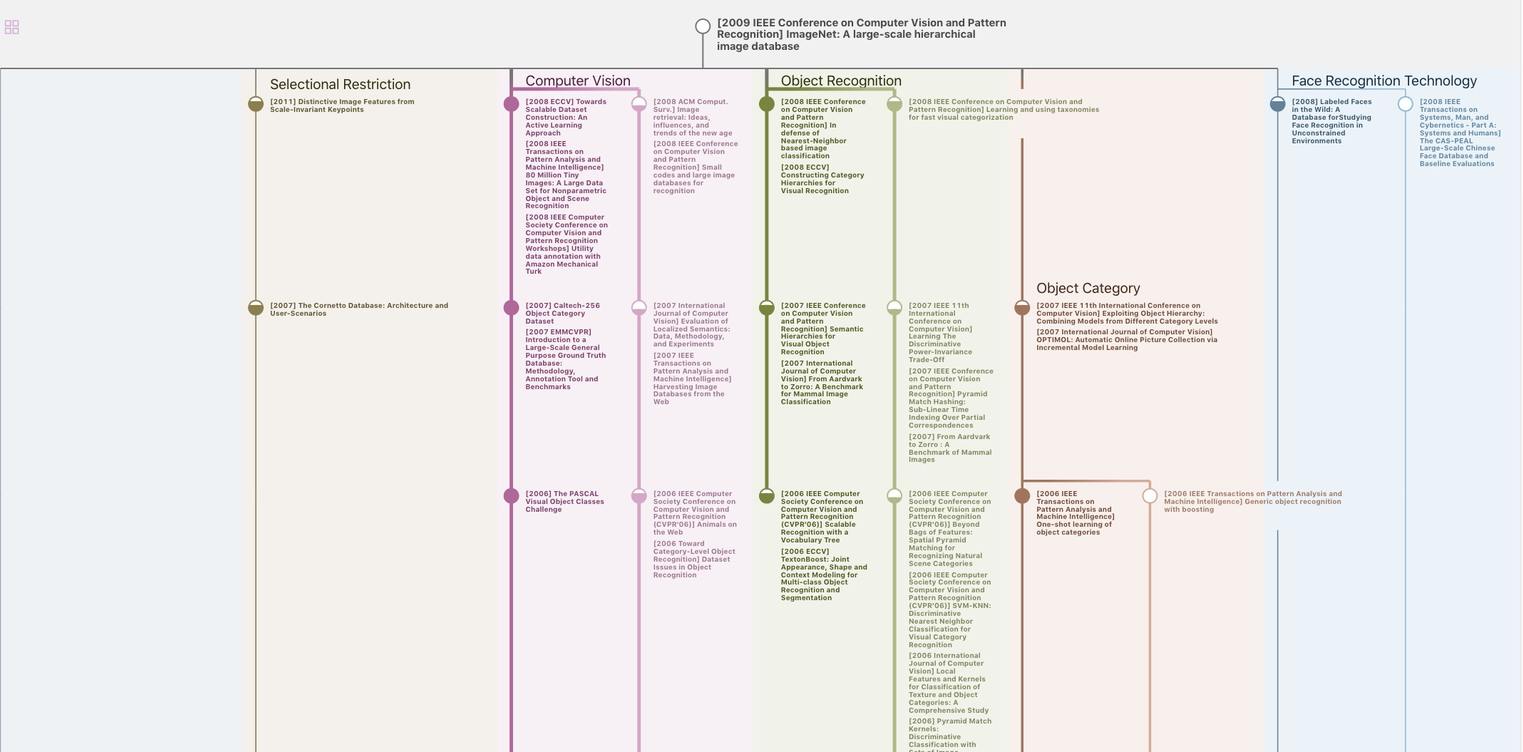
生成溯源树,研究论文发展脉络
Chat Paper
正在生成论文摘要