CARL: Control-Augmented Reinforcement Learning for Decision-Making with Stochastic Factors
semanticscholar(2022)
摘要
We study finite-horizon dynamic decision-making in the presence of uncontrolled system variables, referred to as stochastic factors. Existing solutions fall into two distinctive categories: (i) Reinforcement learning employs end-to-end policy learning with flexible factor representation, but does not precisely model the dynamics of the problem; (ii) control-based methods, in contrast, take advantage of explicitly modeled dynamics via model fitting (calibration) but is constrained on factor representation. We propose CARL (controlaugmented RL), a decision-making framework that interpolates between the two extremes by simultaneously conducting policy learning and model fitting. We prove the convergence of CARL and provide performance guarantees via a finitesample error bound. On three diverse tasks ranging from linear-quadratic control, portfolio optimization, and epidemic spread management, we observe that CARL can outperform four leading methods for these tasks.
更多查看译文
AI 理解论文
溯源树
样例
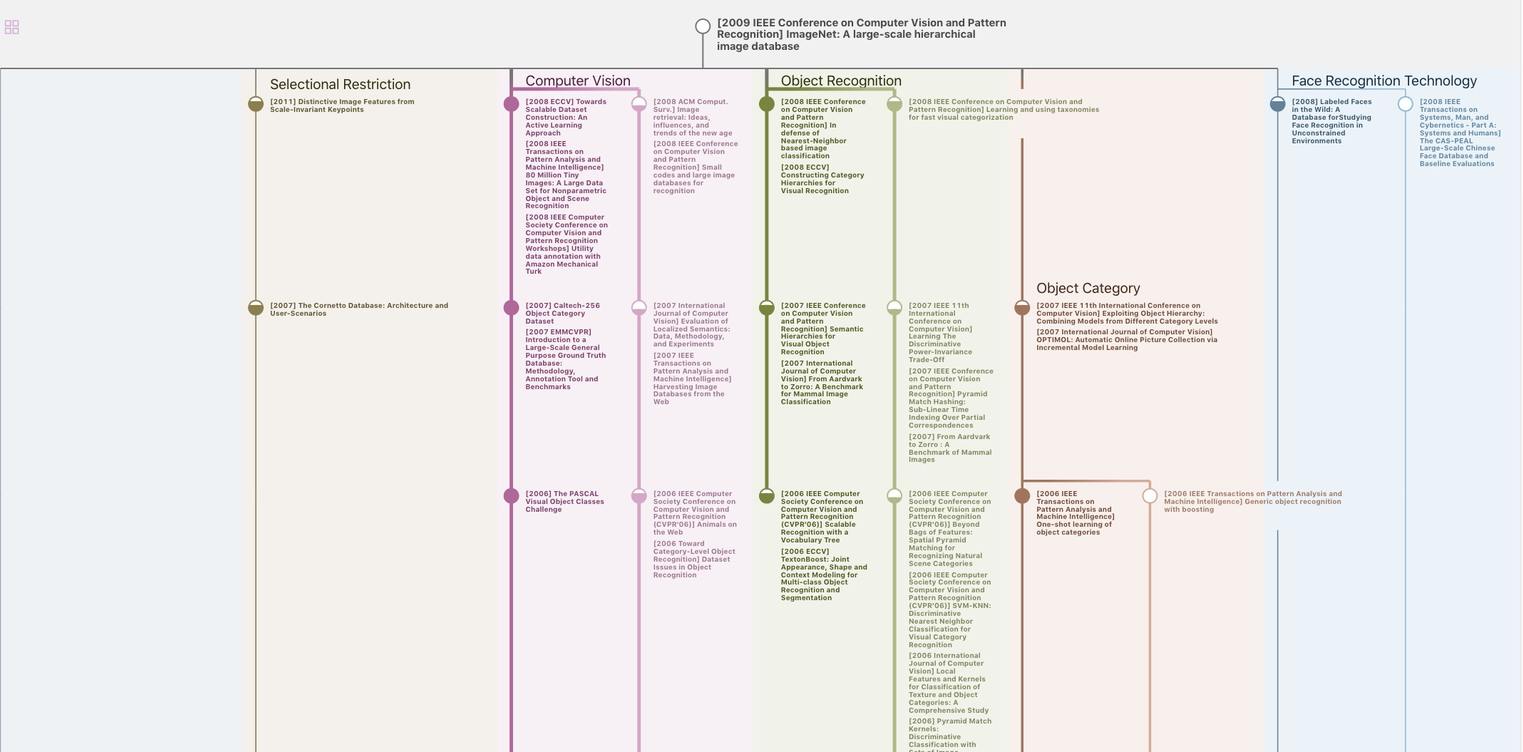
生成溯源树,研究论文发展脉络
Chat Paper
正在生成论文摘要